Fix Bugs with Transformer through a Neural-Symbolic Edit Grammar
arXiv (Cornell University)(2022)
摘要
We introduce NSEdit (neural-symbolic edit), a novel Transformer-based code repair method. Given only the source code that contains bugs, NSEdit predicts an editing sequence that can fix the bugs. The edit grammar is formulated as a regular language, and the Transformer uses it as a neural-symbolic scripting interface to generate editing programs. We modify the Transformer and add a pointer network to select the edit locations. An ensemble of rerankers are trained to re-rank the editing sequences generated by beam search. We fine-tune the rerankers on the validation set to reduce over-fitting. NSEdit is evaluated on various code repair datasets and achieved a new state-of-the-art accuracy ($24.04\%$) on the Tufano small dataset of the CodeXGLUE benchmark. NSEdit performs robustly when programs vary from packages to packages and when buggy programs are concrete. We conduct detailed analysis on our methods and demonstrate the effectiveness of each component.
更多查看译文
关键词
transformer,neural-symbolic
AI 理解论文
溯源树
样例
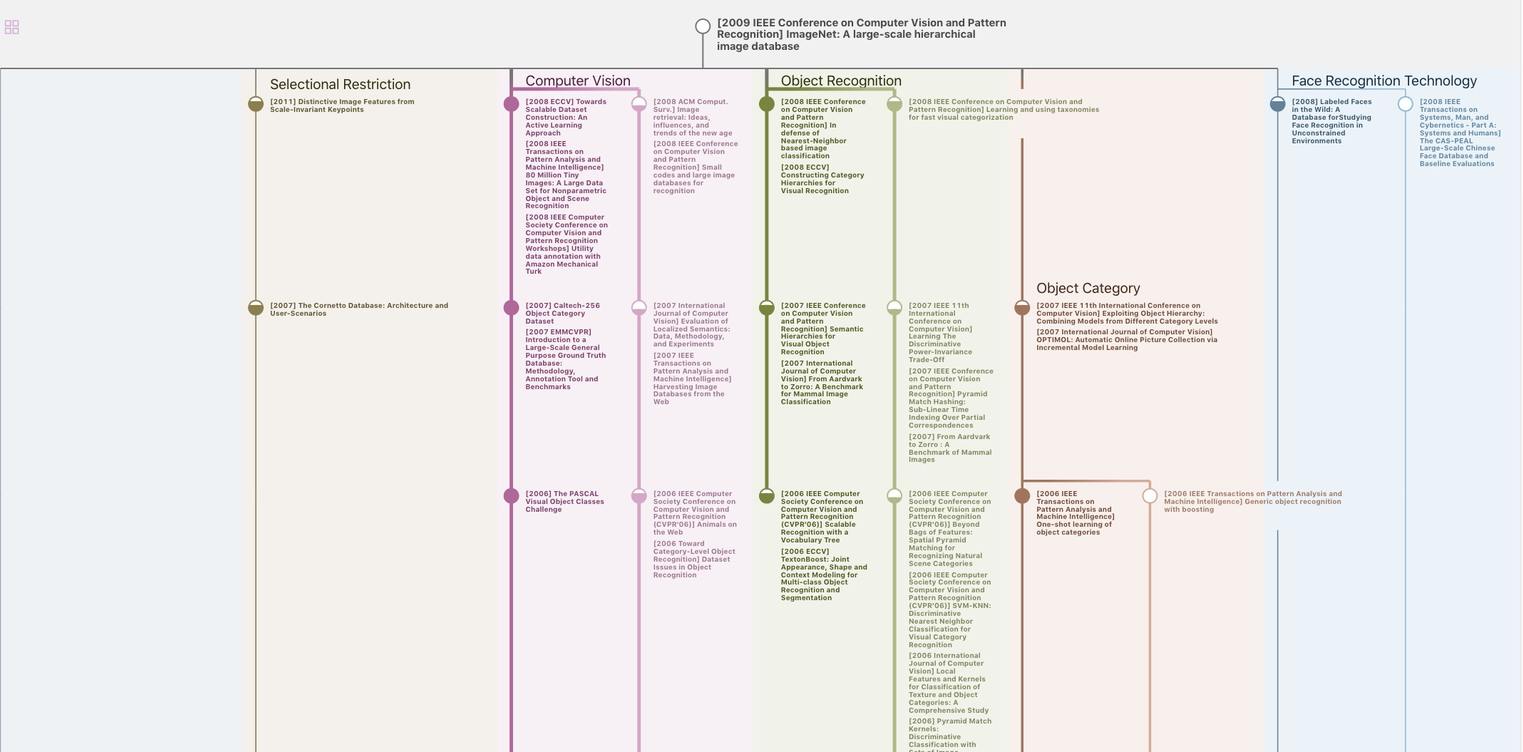
生成溯源树,研究论文发展脉络
Chat Paper
正在生成论文摘要