Data-driven reconstruction of spectral conductivity and chemical potential from thermoelectric transport data
arXiv (Cornell University)(2022)
摘要
The spectral conductivity, i.e., the electrical conductivity as a function of the Fermi energy, is a cornerstone in determining the thermoelectric transport properties of electrons. However, the spectral conductivity depends on sample-specific properties such as carrier concentrations, vacancies, charge impurities, chemical compositions, and material microstructures, making it difficult to relate the experimental result with the theoretical prediction directly. Here, we propose a data-driven approach based on machine learning to reconstruct the spectral conductivity and chemical potential from the thermoelectric transport data. Using this machine learning method, we first demonstrate that the spectral conductivity and temperature-dependent chemical potentials can be recovered within a simple toy model. In a second step, we apply our method to experimental data in doped one-dimensional telluride Ta$_4$SiTe$_4$~[T. Inohara, \textit{et al.}, Appl. Phys. Lett. \textbf{110}, 183901 (2017)] to reconstruct the spectral conductivity and chemical potential for each sample. Furthermore, the thermal conductivity of electrons and the maximal figure of merit $ZT$ are estimated from the reconstructed spectral conductivity, which provides accurate estimates beyond the Wiedemann-Franz law. Our study clarifies the connection between the thermoelectric transport properties and the low-energy electronic states of real materials, and establishes a promising route to incorporate experimental data into traditional theory-driven workflows.
更多查看译文
关键词
thermoelectric transport data-driven,spectral conductivity
AI 理解论文
溯源树
样例
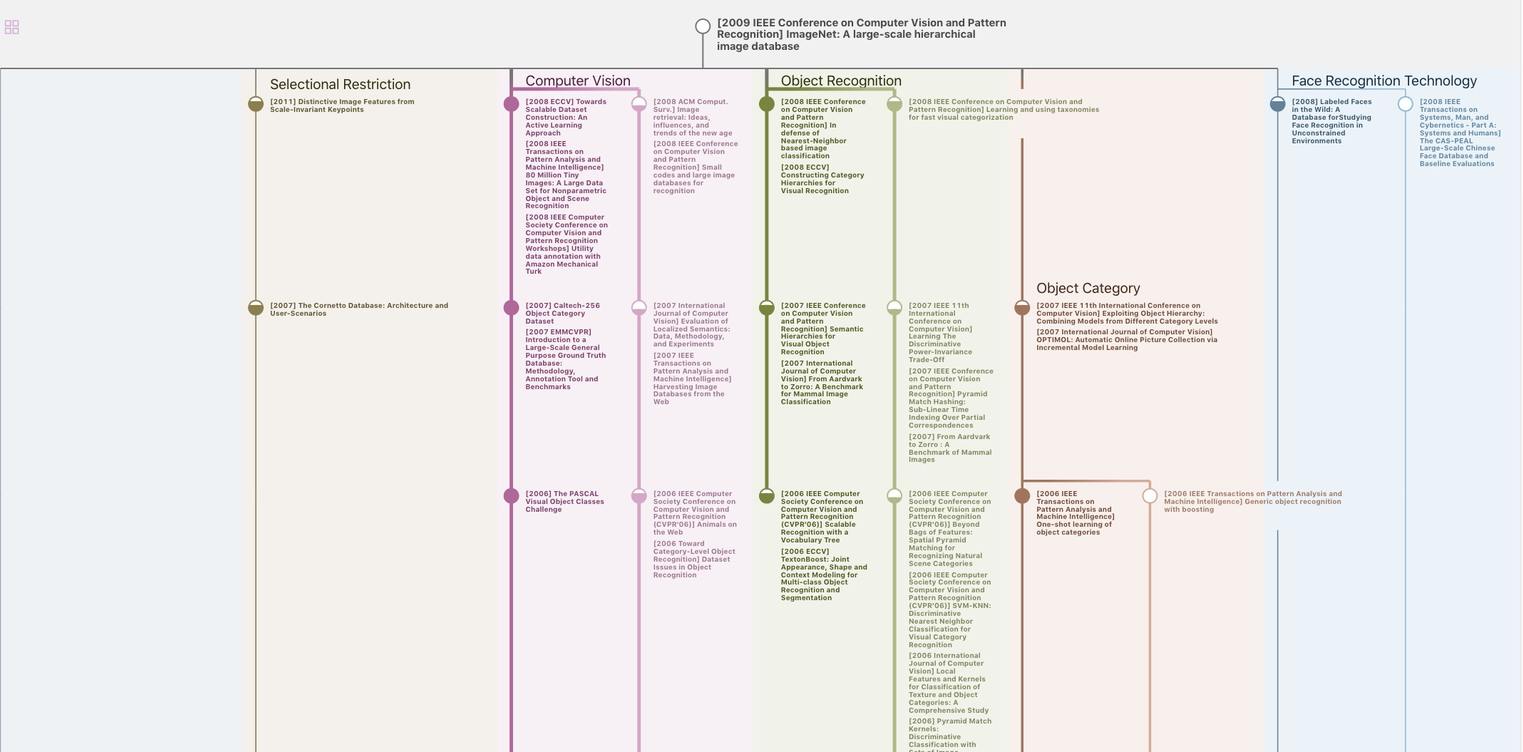
生成溯源树,研究论文发展脉络
Chat Paper
正在生成论文摘要