TE2Rules: Explaining Tree Ensembles using Rules
arXiv (Cornell University)(2022)
摘要
Tree Ensemble (TE) models (like Gradient Boosted Trees) often provide higher prediction performance compared to single decision trees. However, TE models generally lack transparency and interpretability, as humans have difficulty understanding their decision logic. This paper presents a novel approach to convert a TE trained for a binary classification task, to a rule list (RL) that closely approximates the TE and is interpretable for a human. This RL can effectively explain the model even on the minority class predicted by the model. Experiments on benchmark datasets demonstrate that, (i) predictions from the RL generated by TE2Rules have higher fidelity (with respect to the original TE) compared to state-of-the-art methods, (ii) the run-time of TE2Rules is comparable to that of some other similar baselines and (iii) the run-time of TE2Rules algorithm can be traded off at the cost of a slightly lower fidelity.
更多查看译文
关键词
tree ensembles,te2rules
AI 理解论文
溯源树
样例
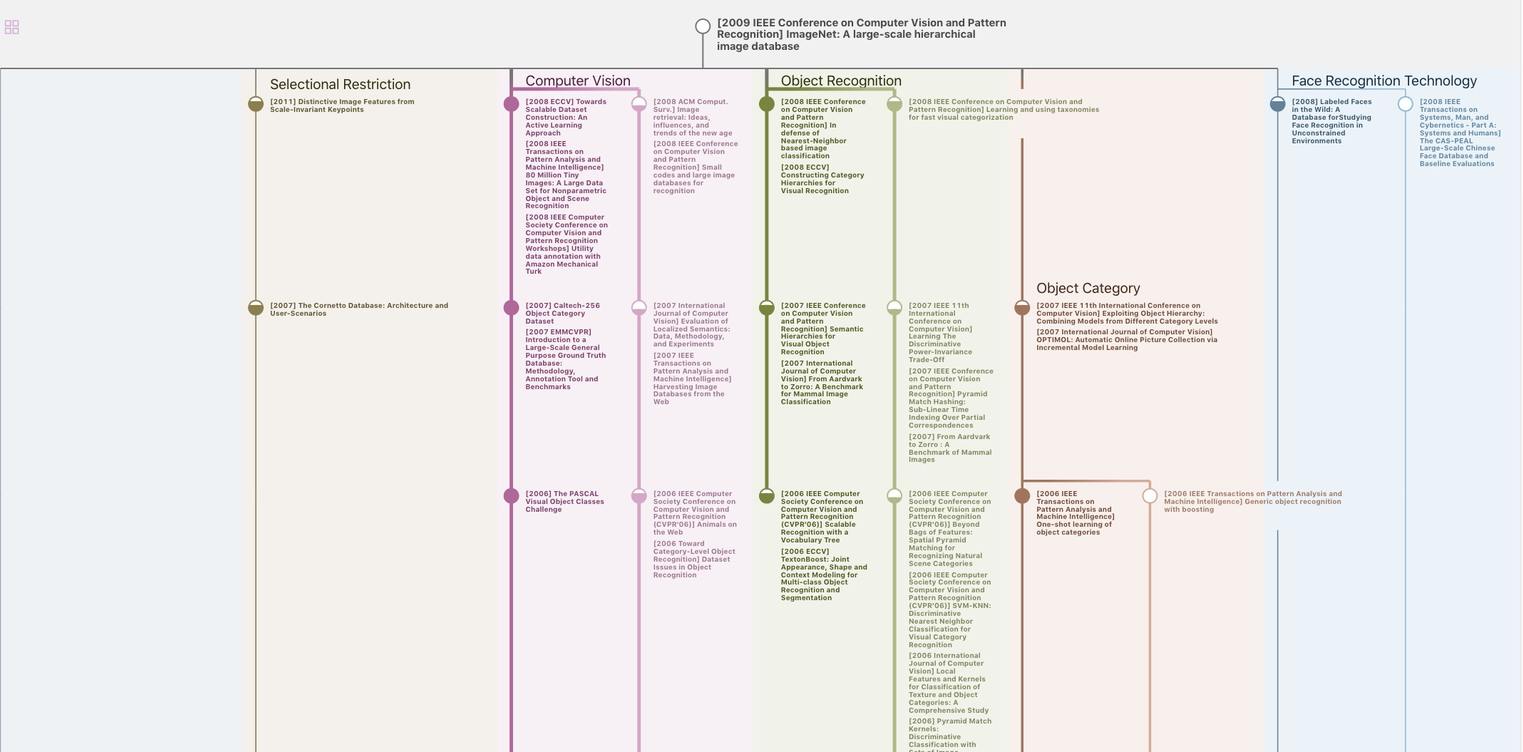
生成溯源树,研究论文发展脉络
Chat Paper
正在生成论文摘要