Energy performance estimation for large building portfolios with machine learning-based techniques
Acta Polytechnica CTU Proceedings(2022)
摘要
Building operation is responsible for 28% of the world’s carbon emissions. In this context, establishing priorities in refurbishment strategies at the scale of a city or a group of buildings is important. Such procedures are usually led by experts in energy performance and, therefore, they are rarely carried out due to their long and costly nature.This research aims at the estimation of building energy performance to pave the way towards finding near-optimal refurbishment strategies. Thanks to the identification of easily-accessible building characteristics, the method applies machine learning models to scan a building portfolio based on a low level of details. The results show good potential to identify low-performer buildings with simple machine learning methods. It also opens the door for further improvements through the inclusion of supplementary building features at the input of the predictive system.This work includes (a) the integration of a knowledge database thanks to the Swiss CECB energy performance certificates, referencing more than 70 000 buildings, (b) the preparation of a training data set through the selection of relevant physical characteristics of buildings (input) and the corresponding energy consumption labels (output), (c) the development of predictive models used in a supervised way, (d) their evaluation on an independent test set.
更多查看译文
关键词
large building portfolios,energy performance,learning-based
AI 理解论文
溯源树
样例
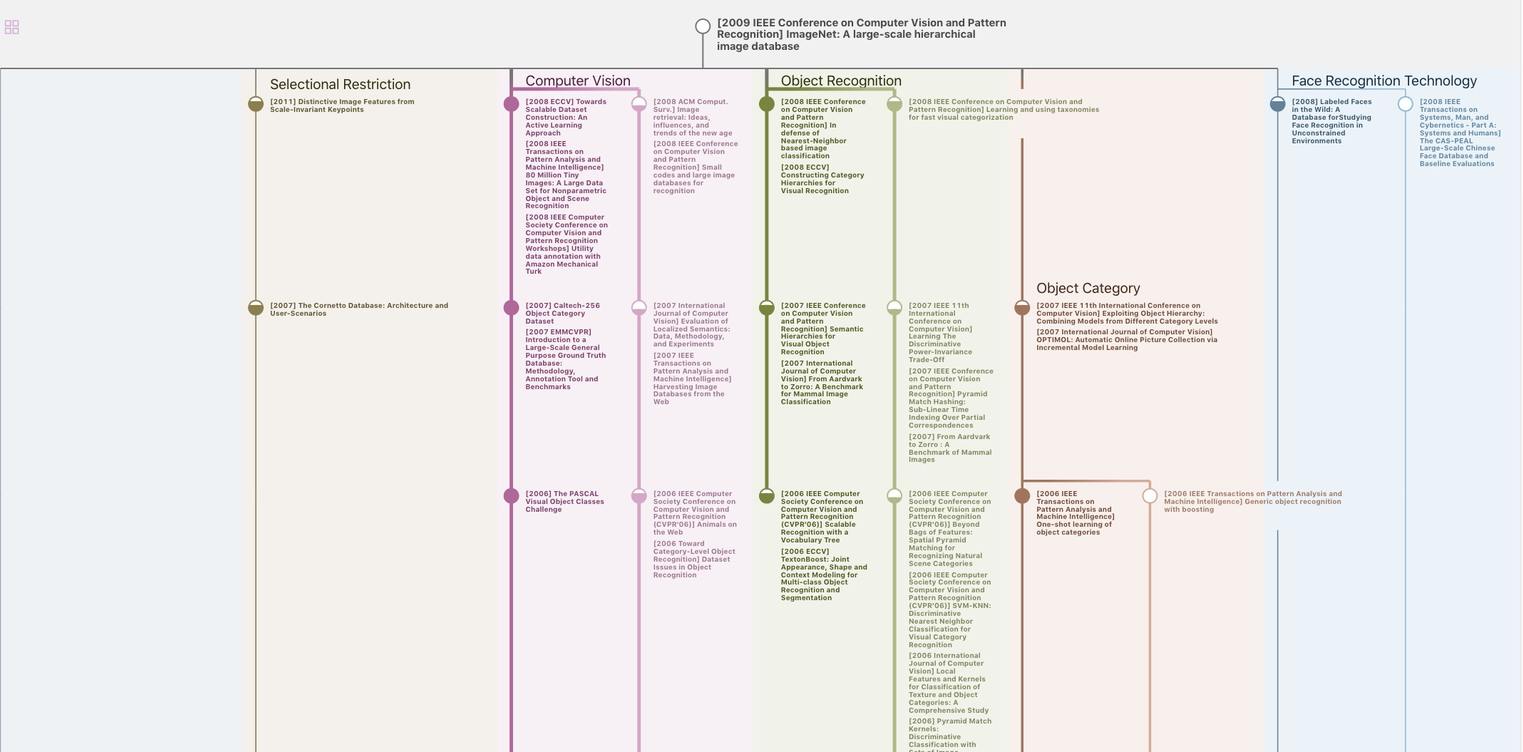
生成溯源树,研究论文发展脉络
Chat Paper
正在生成论文摘要