Implementation and Learning of Quantum Hidden Markov Models
arXiv (Cornell University)(2022)
摘要
In this article we use the theory of quantum channels and open quantum systems to provide an efficient unitary characterization of a class of stochastic generators known as quantum hidden Markov models (QHMMs). By utilizing the unitary characterization, we demonstrate that any QHMM can be implemented as a quantum circuit with mid-circuit measurement. We prove that QHMMs are more efficient definitions of stochastic process languages compared to the equivalent classical hidden Markov Models (HMMs). Starting with the formulation of QHMMs as quantum channels, we employ Stinespring's construction to represent these models as unitary quantum circuits with mid-circuit measurement. By utilizing the unitary parameterization of QHMMs, we define a formal QHMM learning model. The model formalizes the empirical distributions of target stochastic process languages, defines hypothesis space of quantum circuits, and introduces an empirical stochastic divergence measure - hypothesis fitness - as a success criterion for learning. We demonstrate that the learning model has a smooth search landscape due to the continuity of Stinespring's dilation. The smooth mapping between the hypothesis and fitness spaces enables the development of efficient heuristic and gradient descent learning algorithms. We propose two practical learning algorithms for QHMMs. The first algorithm is a hyperparameter-adaptive evolutionary search. The second algorithm learns the QHMM as a quantum ansatz circuit using a multi-parameter non-linear optimization technique.
更多查看译文
关键词
models,learning,implementation
AI 理解论文
溯源树
样例
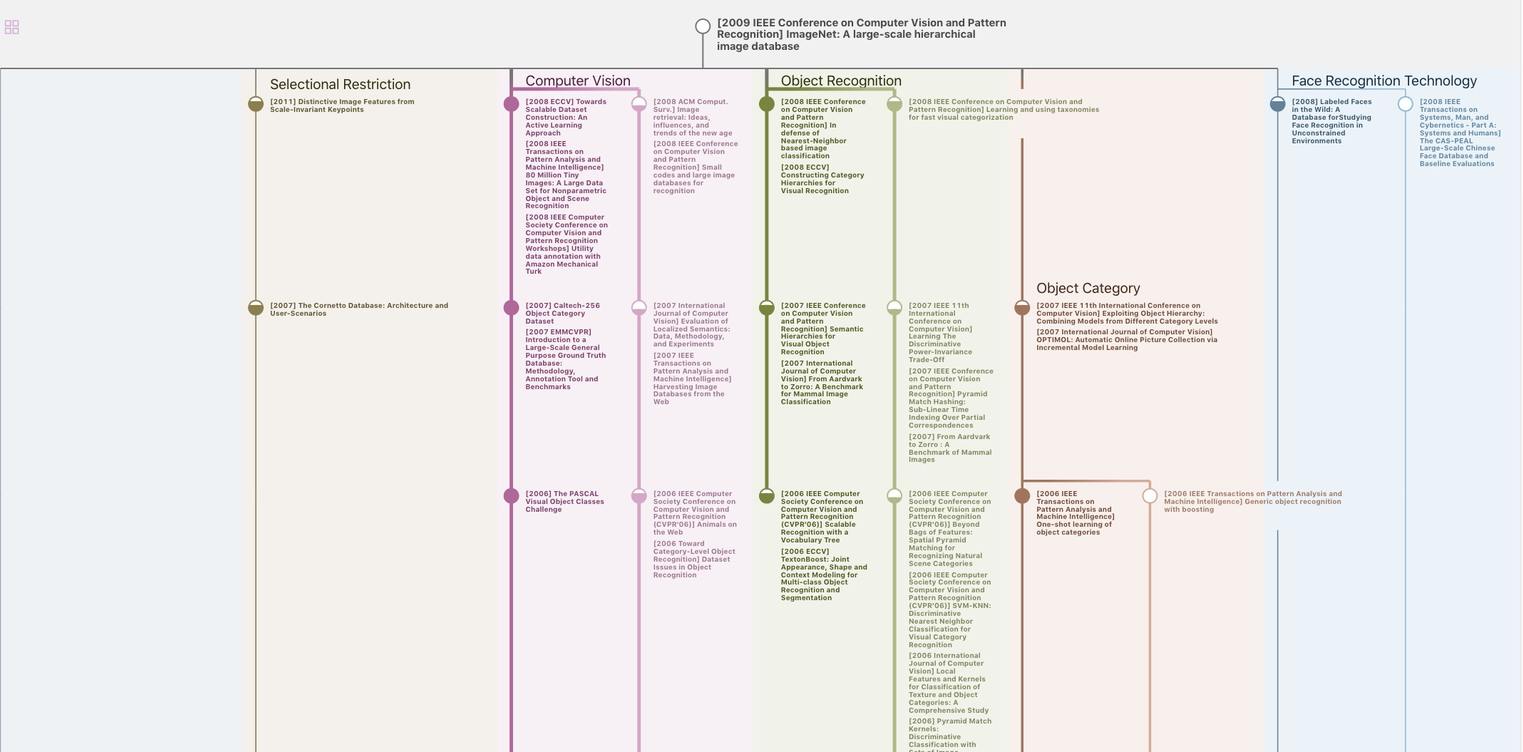
生成溯源树,研究论文发展脉络
Chat Paper
正在生成论文摘要