Cold-Start Data Selection for Few-shot Language Model Fine-tuning: A Prompt-Based Uncertainty Propagation Approach
arXiv (Cornell University)(2022)
摘要
Large Language Models have demonstrated remarkable few-shot performance, but the performance can be sensitive to the selection of few-shot instances. We propose PATRON, a new method that uses prompt-based uncertainty estimation for data selection for pre-trained language model fine-tuning under cold-start scenarios, i.e., no initial labeled data are available. In PATRON, we design (1) a prompt-based uncertainty propagation approach to estimate the importance of data points and (2) a partition-then-rewrite (PTR) strategy to promote sample diversity when querying for annotations. Experiments on six text classification datasets show that PATRON outperforms the strongest cold-start data selection baselines by up to 6.9%. Besides, with 128 labels only, PATRON achieves 91.0% and 92.1% of the fully supervised performance based on vanilla fine-tuning and prompt-based learning respectively. Our implementation of PATRON is available at \url{https://github.com/yueyu1030/Patron}.
更多查看译文
关键词
selection,uncertainty propagation approach,cold-start,few-shot,fine-tuning,prompt-based
AI 理解论文
溯源树
样例
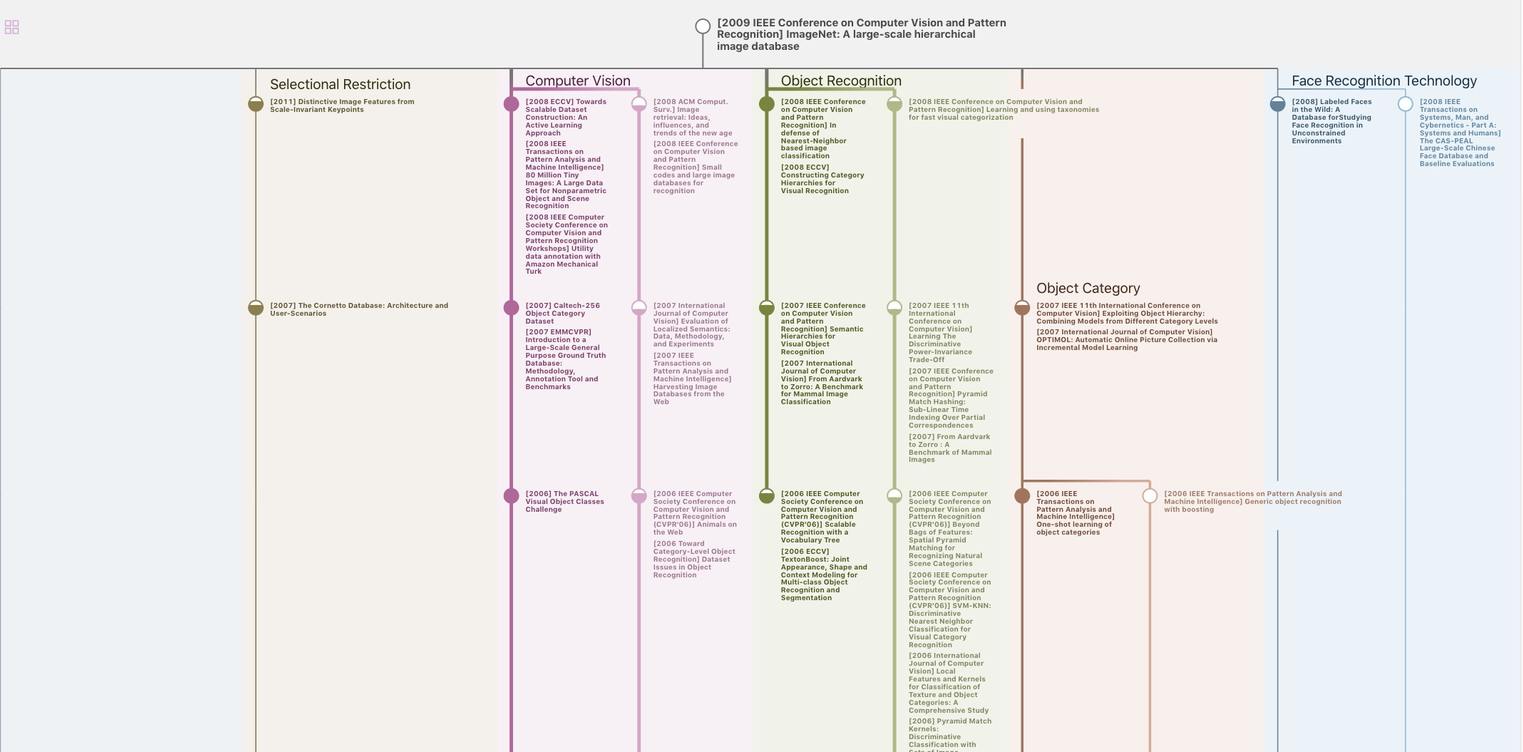
生成溯源树,研究论文发展脉络
Chat Paper
正在生成论文摘要