Provably Efficient Kernelized Q-Learning
arXiv (Cornell University)(2022)
摘要
We propose and analyze a kernelized version of Q-learning. Although a kernel space is typically infinite-dimensional, extensive study has shown that generalization is only affected by the effective dimension of the data. We incorporate such ideas into the Q-learning framework and derive regret bounds for arbitrary kernels. In particular, we provide concrete bounds for linear kernels and Gaussian RBF kernels; notably, the latter bound looks almost identical to the former, only that the actual dimension is replaced by a different notion of dimensionality. Finally, we test our algorithm on a suite of classic control tasks; remarkably, under the Gaussian RBF kernel, it achieves reasonably good performance after only 1000 environmental steps, while its neural network counterpart, deep Q-learning, still struggles.
更多查看译文
关键词
efficient,q-learning
AI 理解论文
溯源树
样例
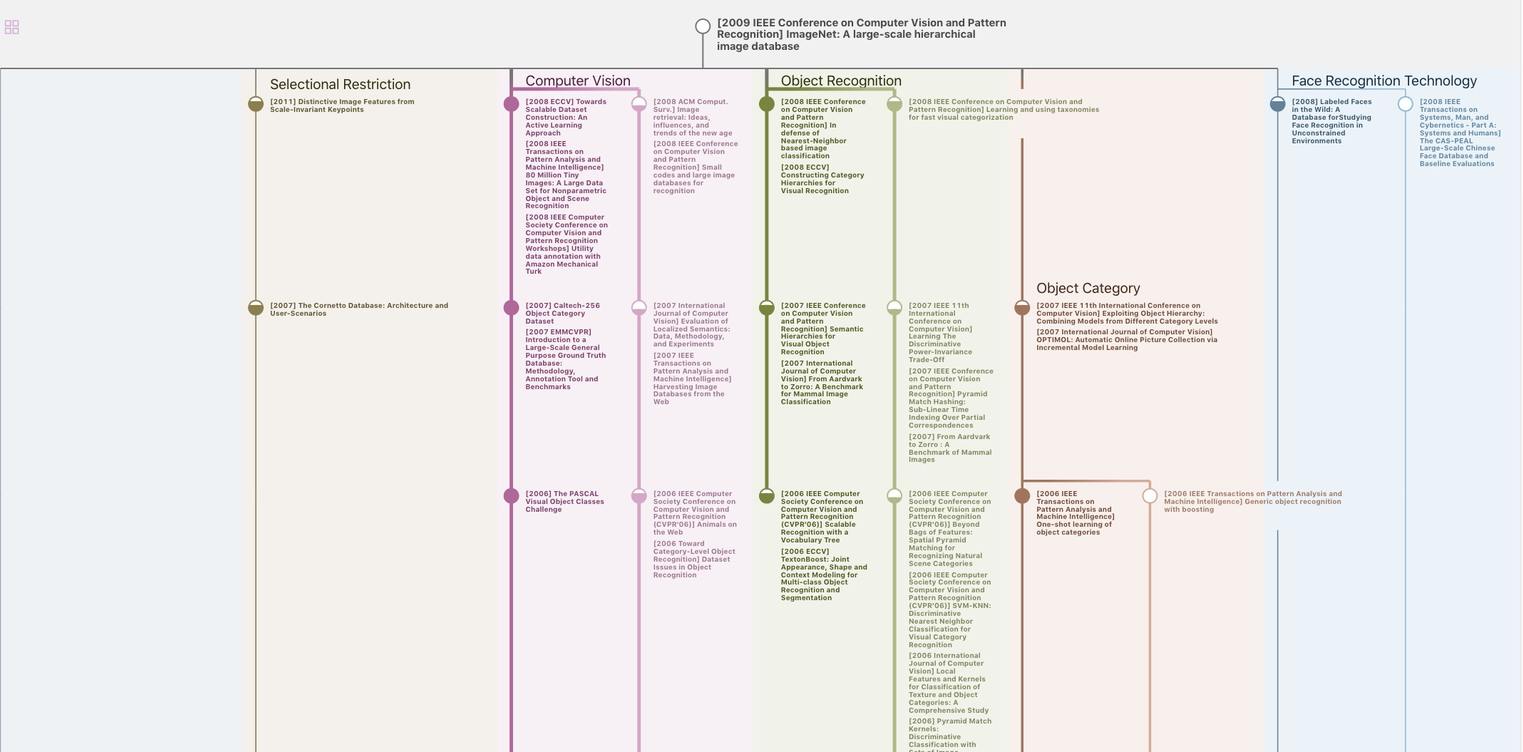
生成溯源树,研究论文发展脉络
Chat Paper
正在生成论文摘要