Dynamic Population Cooperative
International Journal of Swarm Intelligence Research(2022)
摘要
Particle swarm optimization (PSO) has attracted wide attention in the recent decade. Although PSO is an efficient and simple evolutionary algorithm and has been successfully applied to solve optimization problems in many real-world fields, premature maturation and poor local search capability remain two critical issues for PSO. Therefore, to alleviate these disadvantages, a dynamic population cooperative particle swarm optimization for global optimization problems (DPCPSO) is proposed. Firstly, to enhance local search capability, an elite neighborhood learning strategy is constructed by leveraging information from elite particles. Meanwhile, to make the particle easily jump out of the local optimum, a crossover-mutation mechanism is utilized. Finally, a dynamic population partitioning mechanism is designed to balance exploration and exploitation capabilities. 16 classic benchmark functions and 1 real-world optimization problem are used to test the proposed algorithm against with 6 typical PSO algorithms. The experimental results show that DPCPSO is statistically and significantly better than the compared algorithms for most of the test problems. Moreover, the convergence speed and convergence accuracy of DPCPSO are also significantly improved. Therefore, the algorithm is highly competitive in solving global optimization problems.
更多查看译文
关键词
population
AI 理解论文
溯源树
样例
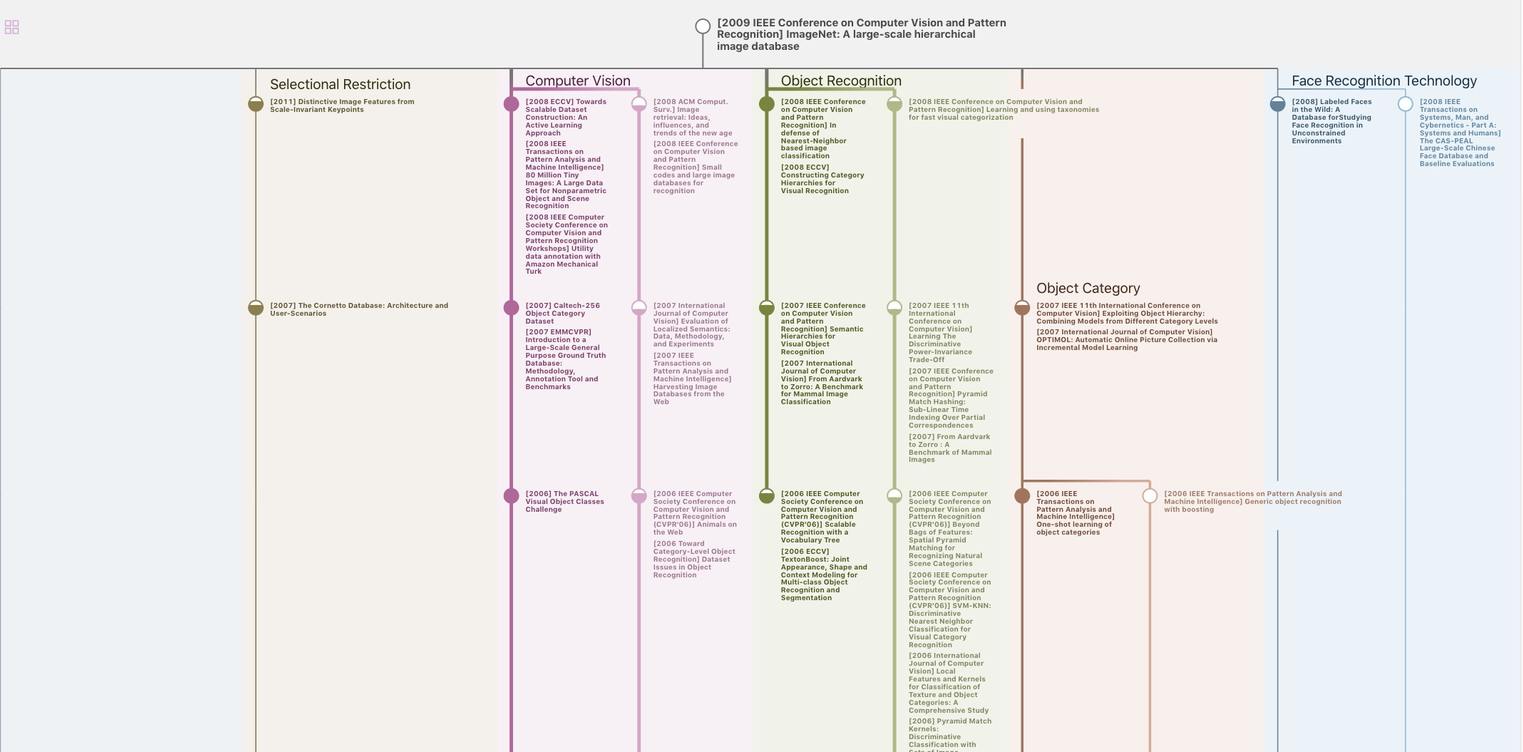
生成溯源树,研究论文发展脉络
Chat Paper
正在生成论文摘要