Learning Informative Health Indicators Through Unsupervised Contrastive Learning
arXiv (Cornell University)(2022)
摘要
Condition monitoring is essential to operate industrial assets safely and efficiently. To achieve this goal, the development of robust health indicators has recently attracted significant attention. These indicators, which provide quantitative real-time insights into the health status of industrial assets over time, serve as valuable tools for fault detection and prognostics. In this study, we propose a novel and universal approach to learn health indicators based on unsupervised contrastive learning. Operational time acts as a proxy for the asset's degradation state, enabling the learning of a contrastive feature space that facilitates the construction of a health indicator by measuring the distance to the healthy condition. To highlight the universality of the proposed approach, we assess the proposed contrastive learning framework in two distinct tasks - wear assessment and fault detection - across two different case studies: a milling machines case study and a real condition monitoring case study of railway wheels from operating trains. First, we evaluate if the health indicator is able to learn the real health condition on a milling machine case study where the ground truth wear condition is continuously measured. Second, we apply the proposed method on a real case study of railway wheels where the ground truth health condition is not known. Here, we evaluate the suitability of the learned health indicator for fault detection of railway wheel defects. Our results demonstrate that the proposed approach is able to learn the ground truth health evolution of milling machines and the learned health indicator is suited for fault detection of railway wheels operated under various operating conditions by outperforming state-of-the-art methods. Further, we demonstrate that our proposed approach is universally applicable to different systems and different health conditions.
更多查看译文
关键词
contrastive feature learning,fault detection,railway
AI 理解论文
溯源树
样例
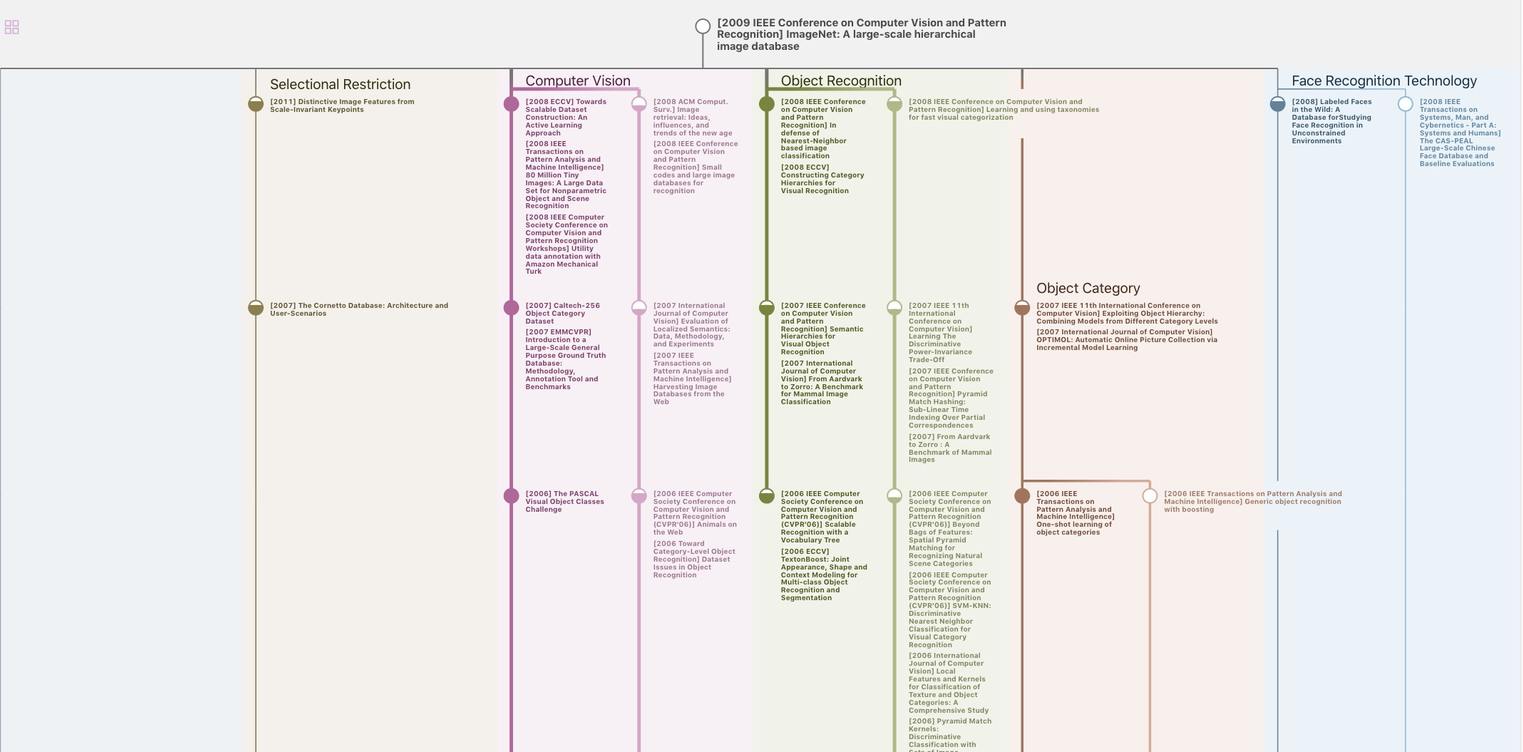
生成溯源树,研究论文发展脉络
Chat Paper
正在生成论文摘要