Subject Granular Differential Privacy in Federated Learning
arXiv (Cornell University)(2022)
摘要
This paper considers subject level privacy in the FL setting, where a subject is an individual whose private information is embodied by several data items either confined within a single federation user or distributed across multiple federation users. We propose two new algorithms that enforce subject level DP at each federation user locally. Our first algorithm, called LocalGroupDP, is a straightforward application of group differential privacy in the popular DP-SGD algorithm. Our second algorithm is based on a novel idea of hierarchical gradient averaging (HiGradAvgDP) for subjects participating in a training mini-batch. We also show that user level Local Differential Privacy (LDP) naturally guarantees subject level DP. We observe the problem of horizontal composition of subject level privacy loss in FL - subject level privacy loss incurred at individual users composes across the federation. We formally prove the subject level DP guarantee for our algorithms, and also show their effect on model utility loss. Our empirical evaluation on FEMNIST and Shakespeare datasets shows that LocalGroupDP delivers the best performance among our algorithms. However, its model utility lags behind that of models trained using a DP-SGD based algorithm that provides a weaker item level privacy guarantee. Privacy loss amplification due to subject sampling fractions and horizontal composition remain key challenges for model utility.
更多查看译文
关键词
subject granular differential privacy,learning
AI 理解论文
溯源树
样例
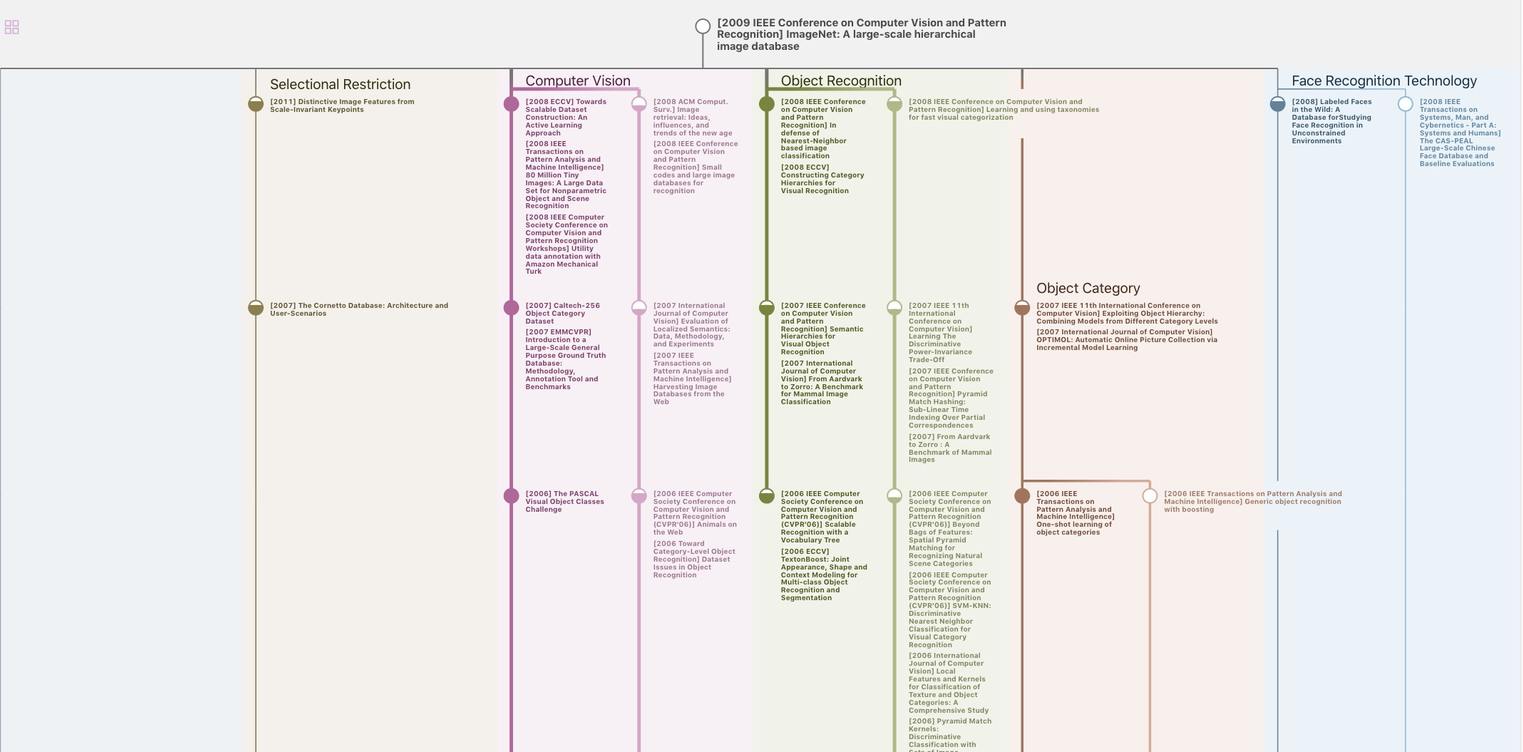
生成溯源树,研究论文发展脉络
Chat Paper
正在生成论文摘要