EICO: Improving Few-Shot Text Classification via Explicit and Implicit Consistency Regularization
Findings of the Association for Computational Linguistics: ACL 2022(2022)
摘要
While the prompt-based fine-tuning methods had advanced few-shot natural language understanding tasks, self-training methods are also being explored. This work revisits the consistency regularization in self-training and presents explicit and implicit consistency regularization enhanced language model (EICO). By employing both explicit and implicit consistency regularization, EICO advances the performance of prompt-based few-shot text classification. For implicit consistency regularization, we generate pseudo-label from the weakly-augmented view and predict pseudo-label from the strongly-augmented view. For explicit consistency regularization, we minimize the difference between the prediction of the augmentation view and the prediction of the original view. We conducted extensive experiments on six text classification datasets and found that with sixteen labeled examples, EICO achieves competitive performance compared to existing self-training few-shot learning methods.
更多查看译文
关键词
regularization,classification,consistency,few-shot
AI 理解论文
溯源树
样例
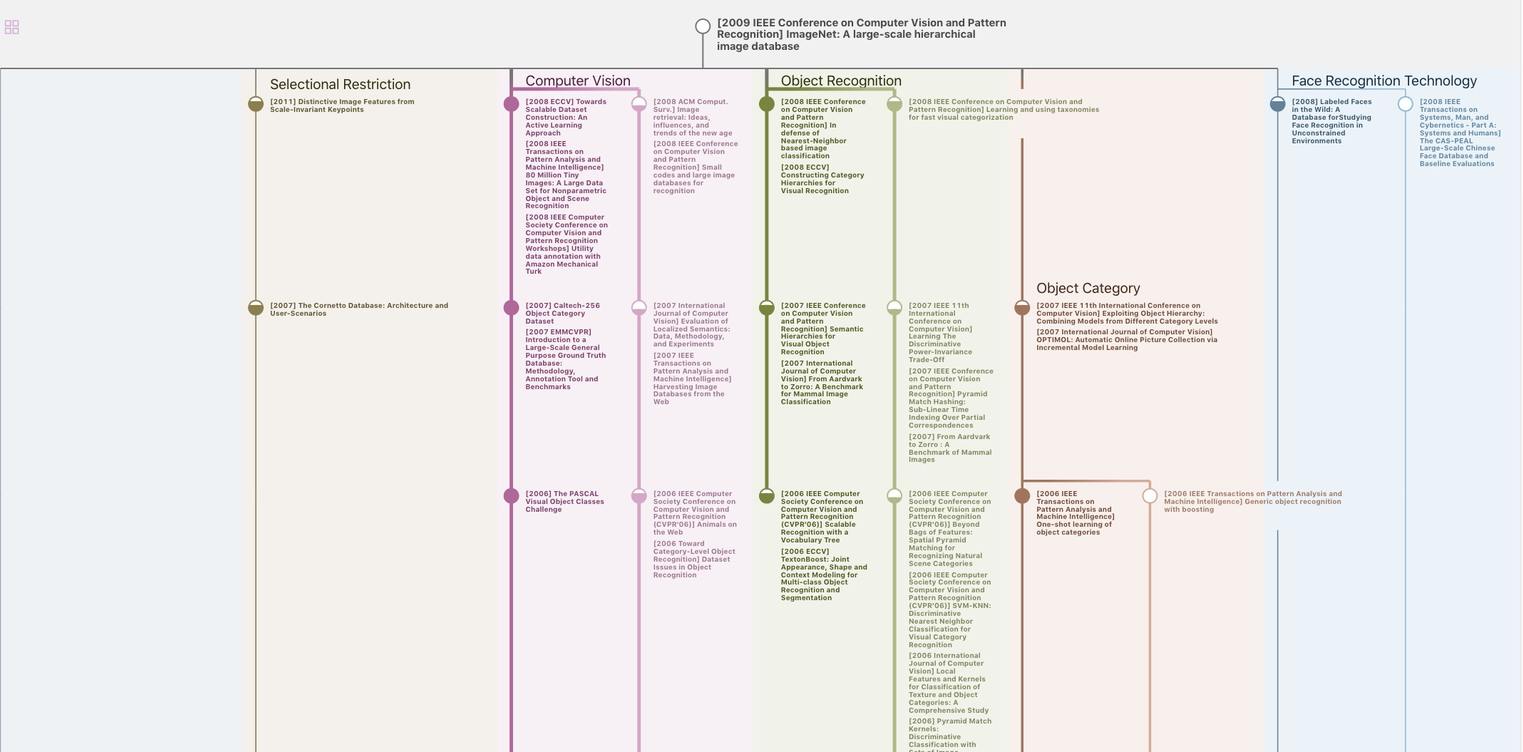
生成溯源树,研究论文发展脉络
Chat Paper
正在生成论文摘要