554 Artificial intelligence (AI)-powered immune phenotyping of advanced or metastatic urothelial carcinoma (aUC) clinical trial samples from hematoxylin and eosin (H&E)-stained whole slide images (WSI)
Regular and Young Investigator Award Abstracts(2022)
摘要
Background
CD8 immune phenotype status is associated with response to anti–PD-L1 therapy in aUC. To assess the tumor microenvironment in aUC, we developed machine learning (ML)–based models to identify cell types and tissue regions in digitized H&E-stained WSI from the JAVELIN Bladder 100 trial, which showed that avelumab (anti–PD-L1) first-line maintenance plus best supportive care (BSC) significantly prolonged overall survival vs BSC alone in patients with aUC. ML-quantified features were used for subsequent slide-level immune phenotyping of these clinical trial samples.Methods
Models previously trained using samples from The Cancer Genome Atlas were refined using 25,926 tissue regions annotations and 183,259 cell type annotations on 704 formalin-fixed, paraffin-embedded, H&E-stained WSI scanned on MIRAX (40x) to identify artifacts, tissue regions (cancer, stroma, and necrosis), and cell types (cancer epithelial cells, lymphocytes, macrophages, fibroblasts, and granulocytes). Precision, recall, and F1 scores were calculated to evaluate model performance. Following pathologist guidelines, H&E slide-level digital immune phenotypes (excluded, inflamed, and desert) were determined using thresholds of lymphocyte area within stroma and proportion of lymphocytes vs all cells in cancer epithelium. The distribution of H&E slide-level digital immune phenotypes was calculated across samples in both trial arms, and the association between immune phenotype and CD8 score at the tumor core was determined using the Kruskal-Wallis and Mann-Whitney U tests.Results
Precision, recall, and F1 scores for model predictions were comparable to those of an average annotator across cell types. The model’s concordance with consensus was also higher than that of an average human annotator (Cohen’s kappa, 0.816 vs 0. 680). Calculation of the distribution of H&E slide-level digital immune phenotypes across trial arms showed that most samples in each arm had an excluded immune phenotype, and a slightly higher proportion of samples in the BSC-only arm had an inflamed phenotype. Association between immune phenotype and the gold-standard CD8 immunohistochemistry (IHC) score at the tumor core showed that samples with the inflamed immune phenotype had higher CD8 scores at the tumor core than samples with the excluded immune phenotype (Mann-Whitney, p<0.026).Conclusions
We show an AI-powered approach to determine slide-level immune phenotypes directly from digitized H&E-stained WSI of clinical aUC samples. An association between inflamed immune phenotypes and higher CD8 scores at the tumor core supports the potential use of this method as an alternative to CD8 IHC-based approaches to identify patients who may derive optimal benefit from anti–PD-L1 treatment.Acknowledgements
This research was supported by the healthcare business of Merck KGaA, Darmstadt, Germany (CrossRef Funder ID: 10.13039/100009945) as part of an alliance between the healthcare business of Merck KGaA, Darmstadt, Germany and Pfizer.Trial Registration
NCT02603432 (ClinicalTrials.gov)Ethics Approval
The trial protocol was approved by the independent ethics committee or institutional review board at each participating center.查看译文
关键词
metastatic urothelial carcinoma,ai-powered,artificial intelligence,hematoxylin
AI 理解论文
溯源树
样例
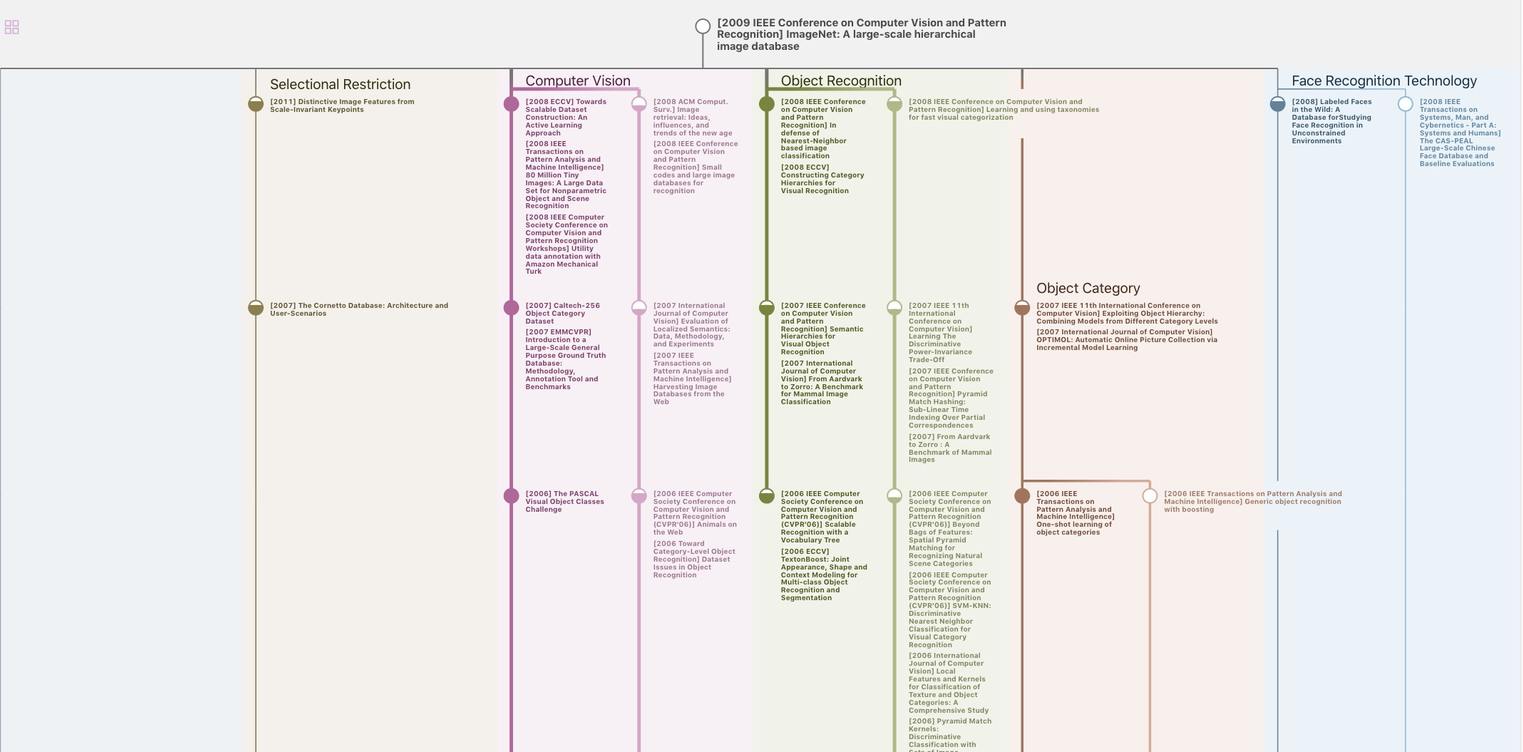
生成溯源树,研究论文发展脉络
Chat Paper
正在生成论文摘要