Multi-target tracking with transferable convolutional neural networks
2023 IEEE 9TH INTERNATIONAL WORKSHOP ON COMPUTATIONAL ADVANCES IN MULTI-SENSOR ADAPTIVE PROCESSING, CAMSAP(2023)
摘要
Multi-target tracking (MTT) is a classical signal processing task, where the goal is to estimate the states of an unknown number of moving targets from noisy sensor measurements. In this paper, we revisit MTT from a deep learning perspective and propose a convolutional neural network (CNN) architecture to tackle it. We represent the target states and sensor measurements as images and recast the problem as an image-to-image prediction task. Then we train a fully convolutional model at small tracking areas and transfer it to much larger areas with numerous targets and sensors. This transfer learning approach enables MTT at a large scale and is also theoretically supported by our novel analysis that bounds the generalization error. In practice, the proposed transferable CNN architecture outperforms random finite set filters on the MTT task with 10 targets and transfers without re-training to a larger MTT task with 250 targets with a 29% performance improvement.
更多查看译文
关键词
Multi-target tracking,convolutional neural networks,transfer learning,stationarity,shift-equivariance
AI 理解论文
溯源树
样例
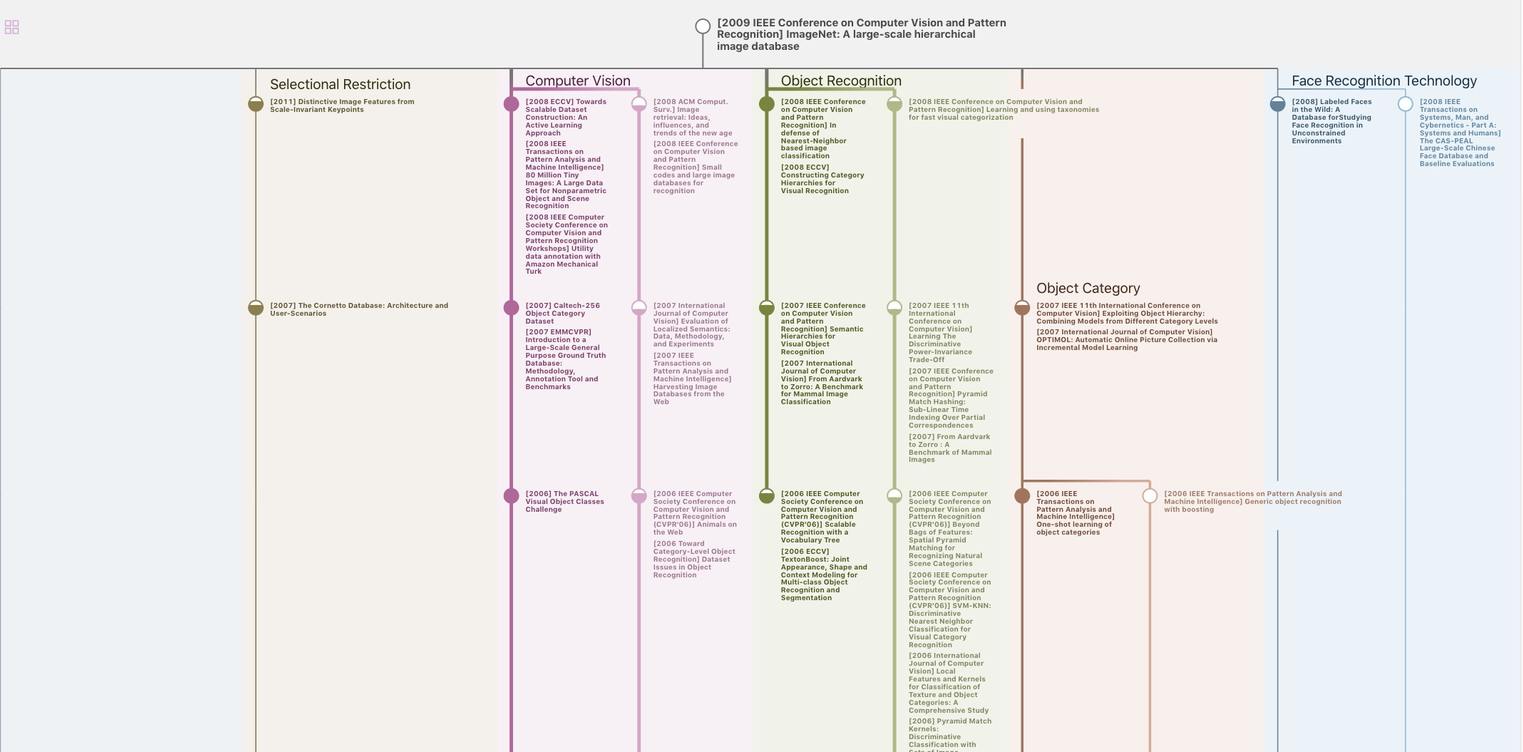
生成溯源树,研究论文发展脉络
Chat Paper
正在生成论文摘要