Learning domain-specific causal discovery from time series
arXiv (Cornell University)(2022)
摘要
Causal discovery (CD) from time-varying data is important in neuroscience, medicine, and machine learning. Techniques for CD encompass randomized experiments, which are generally unbiased but expensive, and algorithms such as Granger causality, conditional-independence-based, structural-equation-based, and score-based methods that are only accurate under strong assumptions made by human designers. However, as demonstrated in other areas of machine learning, human expertise is often not entirely accurate and tends to be outperformed in domains with abundant data. In this study, we examine whether we can enhance domain-specific causal discovery for time series using a data-driven approach. Our findings indicate that this procedure significantly outperforms human-designed, domain-agnostic causal discovery methods, such as Mutual Information, VAR-LiNGAM, and Granger Causality on the MOS 6502 microprocessor, the NetSim fMRI dataset, and the Dream3 gene dataset. We argue that, when feasible, the causality field should consider a supervised approach in which domain-specific CD procedures are learned from extensive datasets with known causal relationships, rather than being designed by human specialists. Our findings promise a new approach toward improving CD in neural and medical data and for the broader machine learning community.
更多查看译文
关键词
discovery,time series,learning,domain-specific
AI 理解论文
溯源树
样例
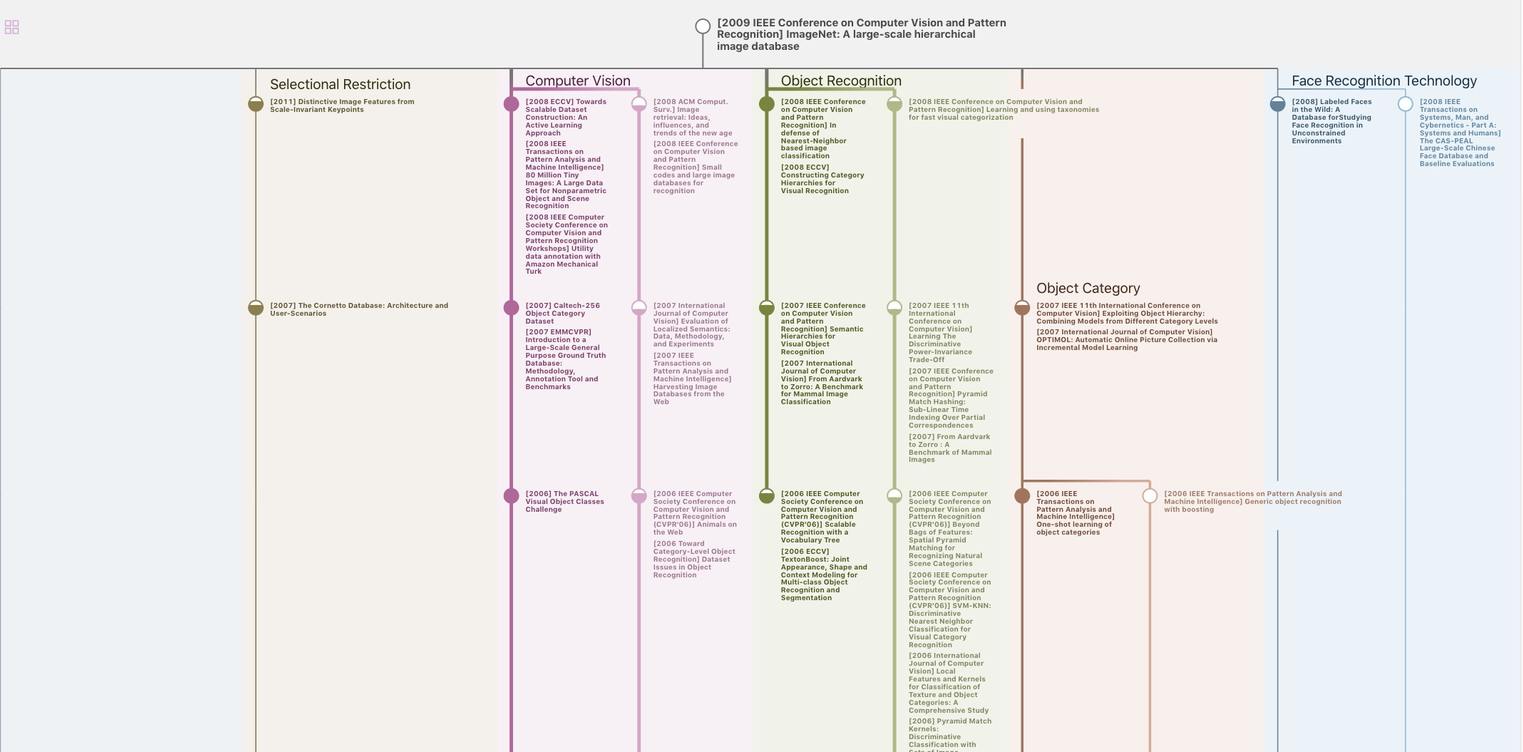
生成溯源树,研究论文发展脉络
Chat Paper
正在生成论文摘要