Learn and Review: Enhancing Continual Named Entity Recognition via Reviewing Synthetic Samples
Findings of the Association for Computational Linguistics: ACL 2022(2022)
摘要
Traditional methods for named entity recognition (NER) classify mentions into a fixed set of pre-defined entity types. However, in many real-world scenarios, new entity types are incrementally involved. To investigate this problem, continual learning is introduced for NER. However, the existing method depends on the relevance between tasks and is prone to inter-type confusion.In this paper, we propose a novel two-stage framework Learn-and-Review (L&R) for continual NER under the type-incremental setting to alleviate the above issues.Specifically, for the learning stage, we distill the old knowledge from teacher to a student on the current dataset. For the reviewing stage, we first generate synthetic samples of old types to augment the dataset. Then, we further distill new knowledge from the above student and old knowledge from the teacher to get an enhanced student on the augmented dataset. This stage has the following advantages: (1) The synthetic samples mitigate the gap between the old and new task and thus enhance the further distillation; (2) Different types of entities are jointly seen during training which alleviates the inter-type confusion. Experimental results show that L&R outperforms the state-of-the-art method on CoNLL-03 and OntoNotes-5.0.
更多查看译文
关键词
continual named entity recognition,reviewing,samples
AI 理解论文
溯源树
样例
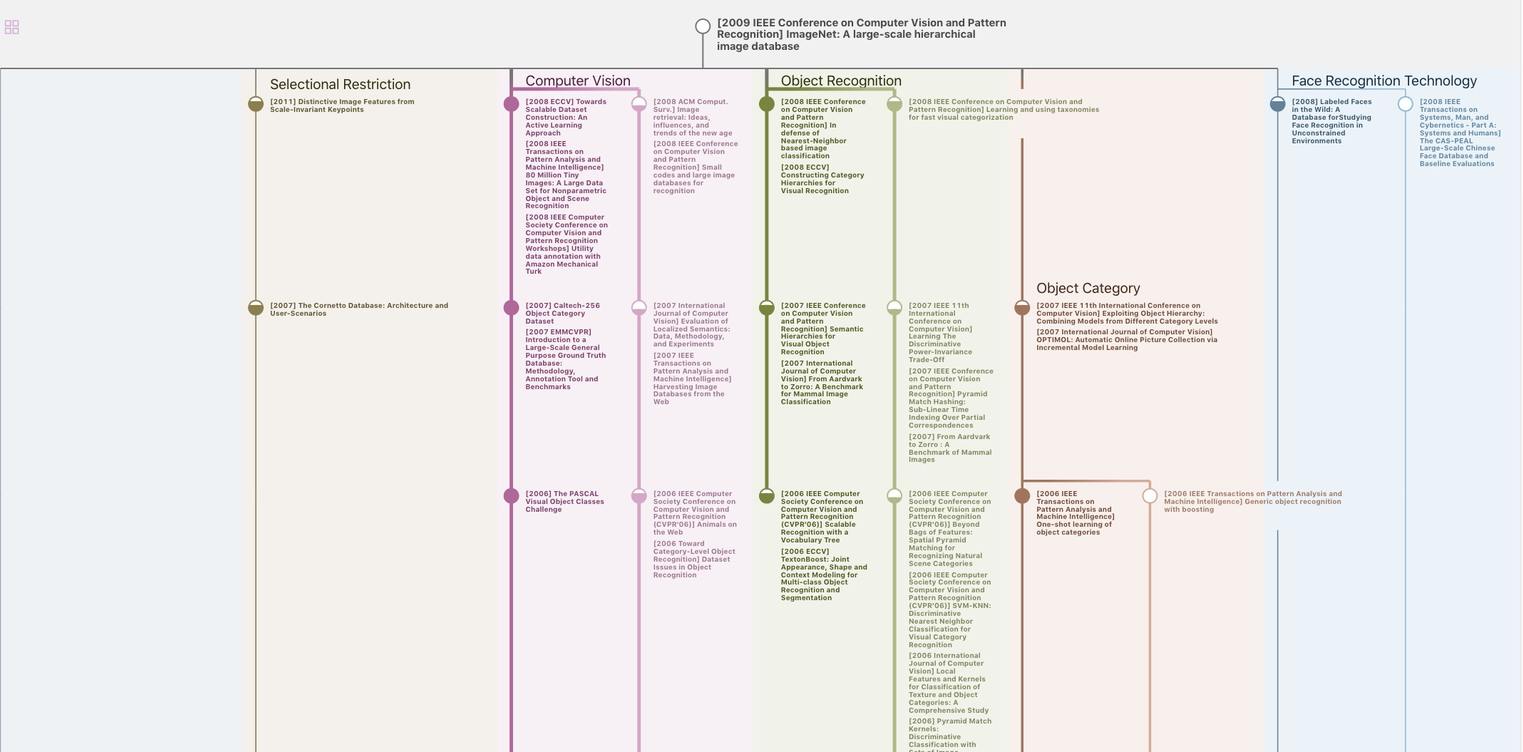
生成溯源树,研究论文发展脉络
Chat Paper
正在生成论文摘要