Real-world knowledge extraction via disentangled variational autoencoders for photon diagnostics
arXiv (Cornell University)(2022)
摘要
We present a real-world application of data processing with neural networks by deploying the use of disentangled variational autoencoders on measured electron time-of-flight data of a diagnostic instrument for online wavelength monitoring at the free electron laser FLASH in Hamburg. Without a-priori knowledge the network is able to find representations of single-shot FEL spectra, which have a low signal-to-noise ratio, that reveal in a directly human-interpretable way crucial information about the photon properties. The central photon energy and the intensity as well as very detector-specific features are identified. The network is also capable of data cleaning, i.e. denoising as well as removal of artefacts. In the reconstruction, this allows for identification of signatures with very low intensity which are hardly recognisable in the raw data. In the present case the network enhances the quality of the diagnostic analysis at FLASH, though it has the potential to improve the analysis of similar kind of spectroscopy data.
更多查看译文
关键词
photon,autoencoders,real-world
AI 理解论文
溯源树
样例
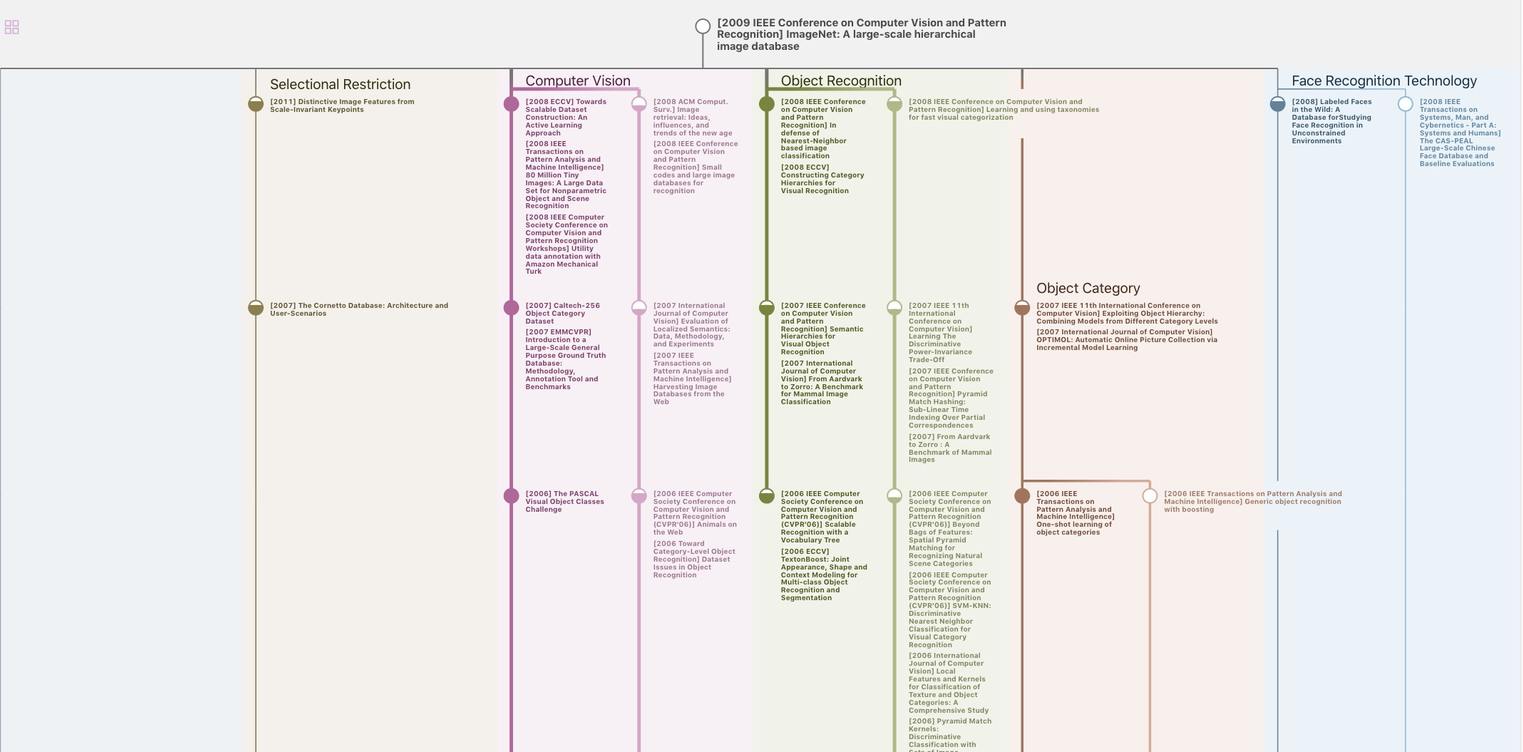
生成溯源树,研究论文发展脉络
Chat Paper
正在生成论文摘要