Truncated Cross-entropy: A New Loss Function for Multi-category Classification
Proceedings of the 2022 2nd International Conference on Control and Intelligent Robotics(2022)
摘要
Deep learning is one of the hottest research topics which has received a lot of attention in recent years. During the training of deep learning models, the loss function is a critical indicating objective which measures the difference between the predicted value and the distribution of the real data. It is also an important indicator for evaluating the performance of a deep learning model. The most popular loss functions used in deep learning include mean square error (MSE), cross-entropy error, etc. Obviously, the loss function has non-negligible influence to the optimizer. The most common optimizers include stochastic gradient descent method (SGD), mini-batch stochastic gradient descent method (MBGD) and Adaptive moment estimation (ADAM). Among them, the MBGD is widely used for its equilibrium between accuracy and speed. However, how to set the batch size is a big challenge. If the batch size is too large, the cost of computation and memory increases accordingly. On the other hand, the gradient descent process could be more oscillated with small batch size. Therefore, this paper proposes a improved loss function named truncated cross-entropy to stabilize the convergence procedure of the optimizer. Experiments show that the proposed method could speed up the convergence of training and reduce the oscillation. The proposed method can achieve similar performance to large-batch-size training with relatively small batch size.
更多查看译文
关键词
classification,new loss function,cross-entropy,multi-category
AI 理解论文
溯源树
样例
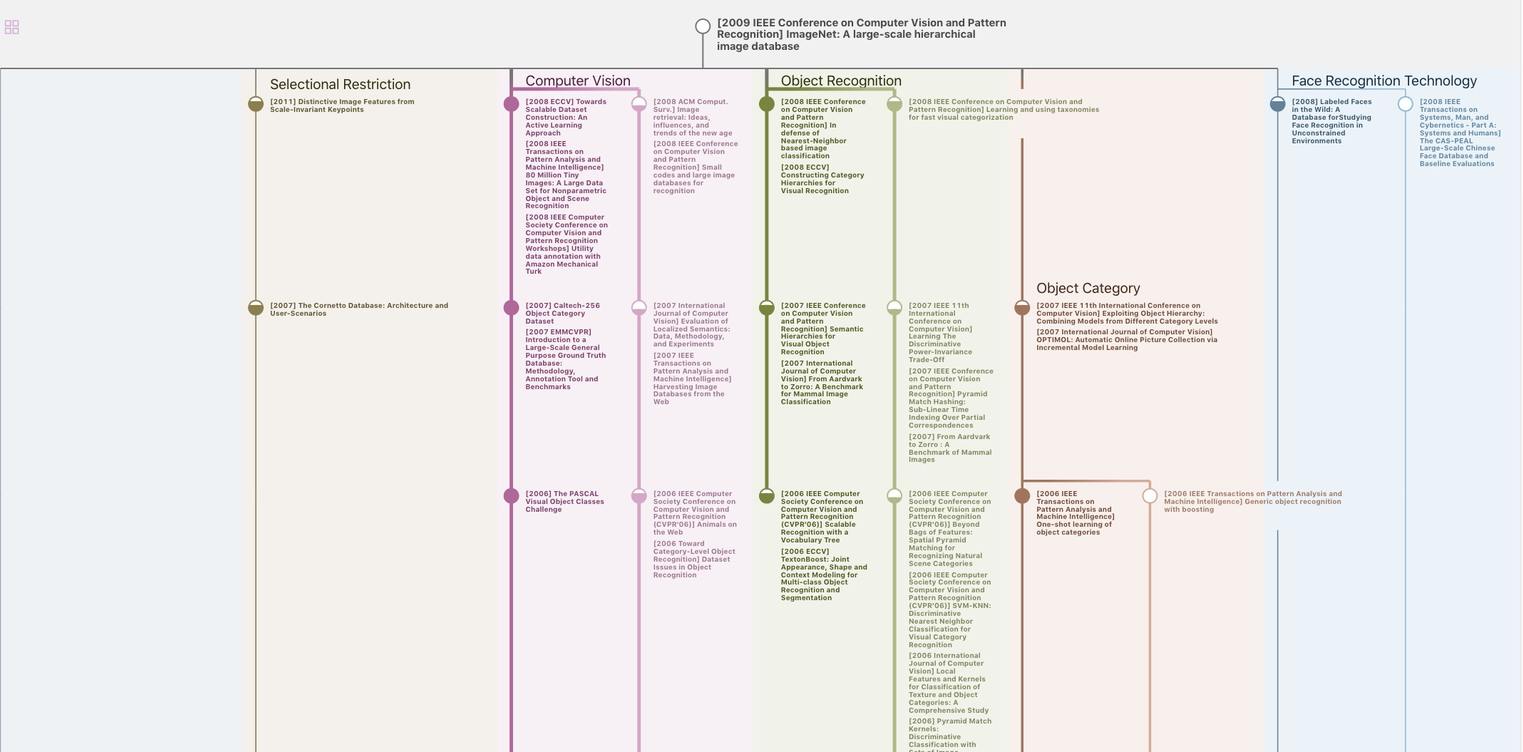
生成溯源树,研究论文发展脉络
Chat Paper
正在生成论文摘要