MoCaPose
Proceedings of the ACM on interactive, mobile, wearable and ubiquitous technologies(2022)
摘要
We present MoCaPose, a novel wearable motion capturing (MoCap) approach to continuously track the wearer's upper body's dynamic poses through multi-channel capacitive sensing integrated in fashionable, loose-fitting jackets. Unlike conventional wearable IMU MoCap based on inverse dynamics, MoCaPose decouples the sensor position from the pose system. MoCaPose uses a deep regressor to continuously predict the 3D upper body joints coordinates from 16-channel textile capacitive sensors, unbound by specific applications. The concept is implemented through two prototyping iterations to first solve the technical challenges, then establish the textile integration through fashion-technology co-design towards a design-centric smart garment. A 38-hour dataset of synchronized video and capacitive data from 21 participants was recorded for validation. The motion tracking result was validated on multiple levels from statistics (R2 ~ 0.91) and motion tracking metrics (MP JPE ~ 86mm) to the usability in pose and motion recognition (0.9 F1 for 10-class classification with unsupervised class discovery). The design guidelines impose few technical constraints, allowing the wearable system to be design-centric and usecase-specific. Overall, MoCaPose demonstrates that textile-based capacitive sensing with its unique advantages, can be a promising alternative for wearable motion tracking and other relevant wearable motion recognition applications.
更多查看译文
AI 理解论文
溯源树
样例
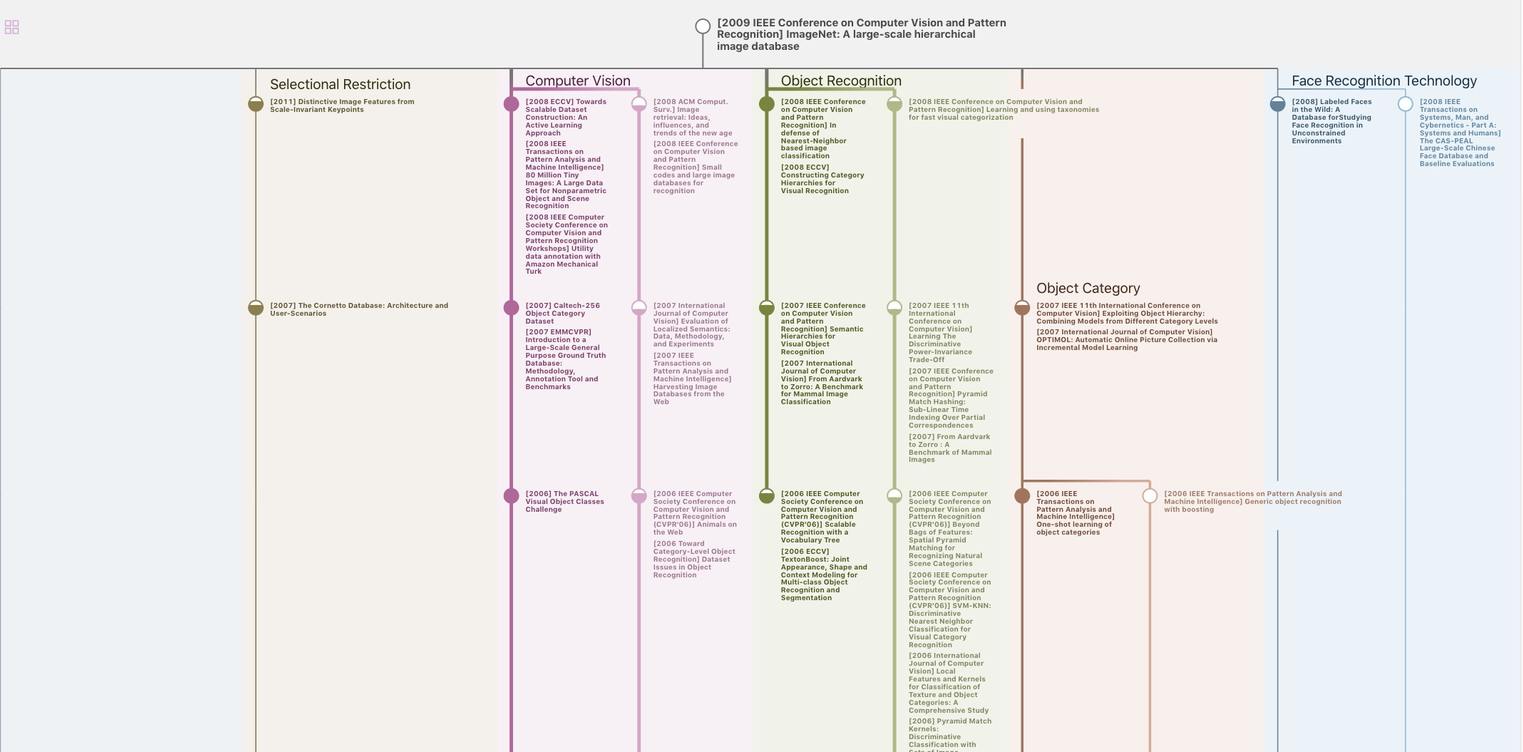
生成溯源树,研究论文发展脉络
Chat Paper
正在生成论文摘要