A Context-Integrated Transformer-Based Neural Network for Auction Design
arXiv (Cornell University)(2022)
摘要
One of the central problems in auction design is developing an incentive-compatible mechanism that maximizes the auctioneer's expected revenue. While theoretical approaches have encountered bottlenecks in multi-item auctions, recently, there has been much progress on finding the optimal mechanism through deep learning. However, these works either focus on a fixed set of bidders and items, or restrict the auction to be symmetric. In this work, we overcome such limitations by factoring \emph{public} contextual information of bidders and items into the auction learning framework. We propose $\mathtt{CITransNet}$, a context-integrated transformer-based neural network for optimal auction design, which maintains permutation-equivariance over bids and contexts while being able to find asymmetric solutions. We show by extensive experiments that $\mathtt{CITransNet}$ can recover the known optimal solutions in single-item settings, outperform strong baselines in multi-item auctions, and generalize well to cases other than those in training.
更多查看译文
关键词
neural network,context-integrated,transformer-based
AI 理解论文
溯源树
样例
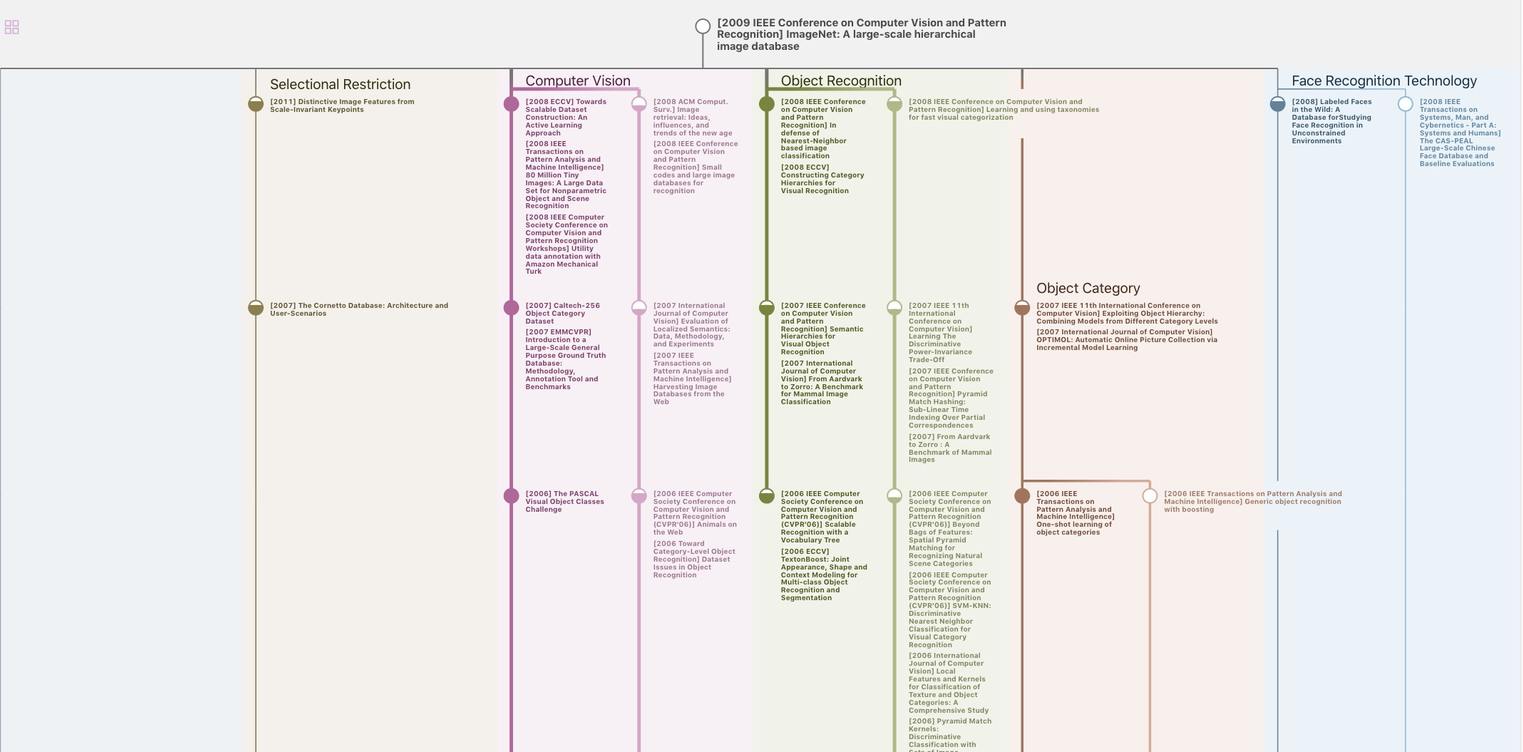
生成溯源树,研究论文发展脉络
Chat Paper
正在生成论文摘要