Micron-scale heterogeneous catalysis with Bayesian force fields from first principles and active learning
arXiv (Cornell University)(2022)
摘要
Quantum-mechanically accurate reactive molecular dynamics (MD) at the scale of billions of atoms has been achieved for the heterogeneous catalytic system of H$_2$/Pt(111) using the FLARE Bayesian force field. This achievement provides accelerated time-to-solution from first principles, with Bayesian active learning enabling efficient and autonomous training of the machine learning model. The resulting model is then deployed in LAMMPS on GPUs using the Kokkos performance portability library. The Bayesian force field provides quantitative uncertainty of predictions on every atomic environment, critical for detecting configurations in large reactive simulations that are outside of the training set. Scaling benchmarks were performed using real-application MD of the H$_2$/Pt(111) heterogeneous catalysis on the Summit supercomputer, with simulations reaching 0.5 trillion atoms on 4556 GPU nodes.
更多查看译文
关键词
heterogeneous catalysis,bayesian force fields,active learning,micron-scale
AI 理解论文
溯源树
样例
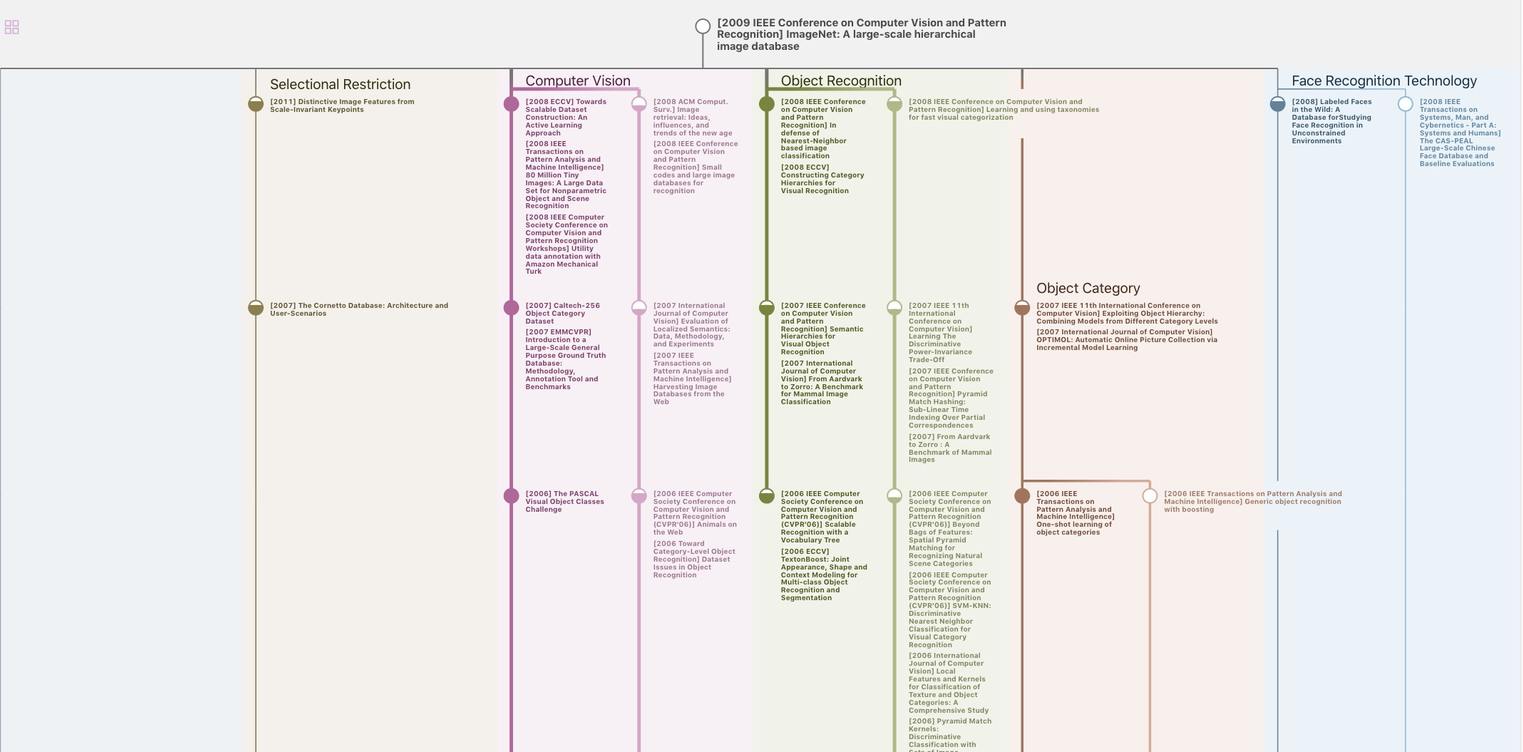
生成溯源树,研究论文发展脉络
Chat Paper
正在生成论文摘要