Self-Supervised Anomaly Detection: A Survey and Outlook
arXiv (Cornell University)(2022)
摘要
Anomaly detection (AD) plays a crucial role in various domains, including cybersecurity, finance, and healthcare, by identifying patterns or events that deviate from normal behaviour. In recent years, significant progress has been made in this field due to the remarkable growth of deep learning models. Notably, the advent of self-supervised learning has sparked the development of novel AD algorithms that outperform the existing state-of-the-art approaches by a considerable margin. This paper aims to provide a comprehensive review of the current methodologies in self-supervised anomaly detection. We present technical details of the standard methods and discuss their strengths and drawbacks. We also compare the performance of these models against each other and other state-of-the-art anomaly detection models. Finally, the paper concludes with a discussion of future directions for self-supervised anomaly detection, including the development of more effective and efficient algorithms and the integration of these techniques with other related fields, such as multi-modal learning.
更多查看译文
关键词
detection,self-supervised
AI 理解论文
溯源树
样例
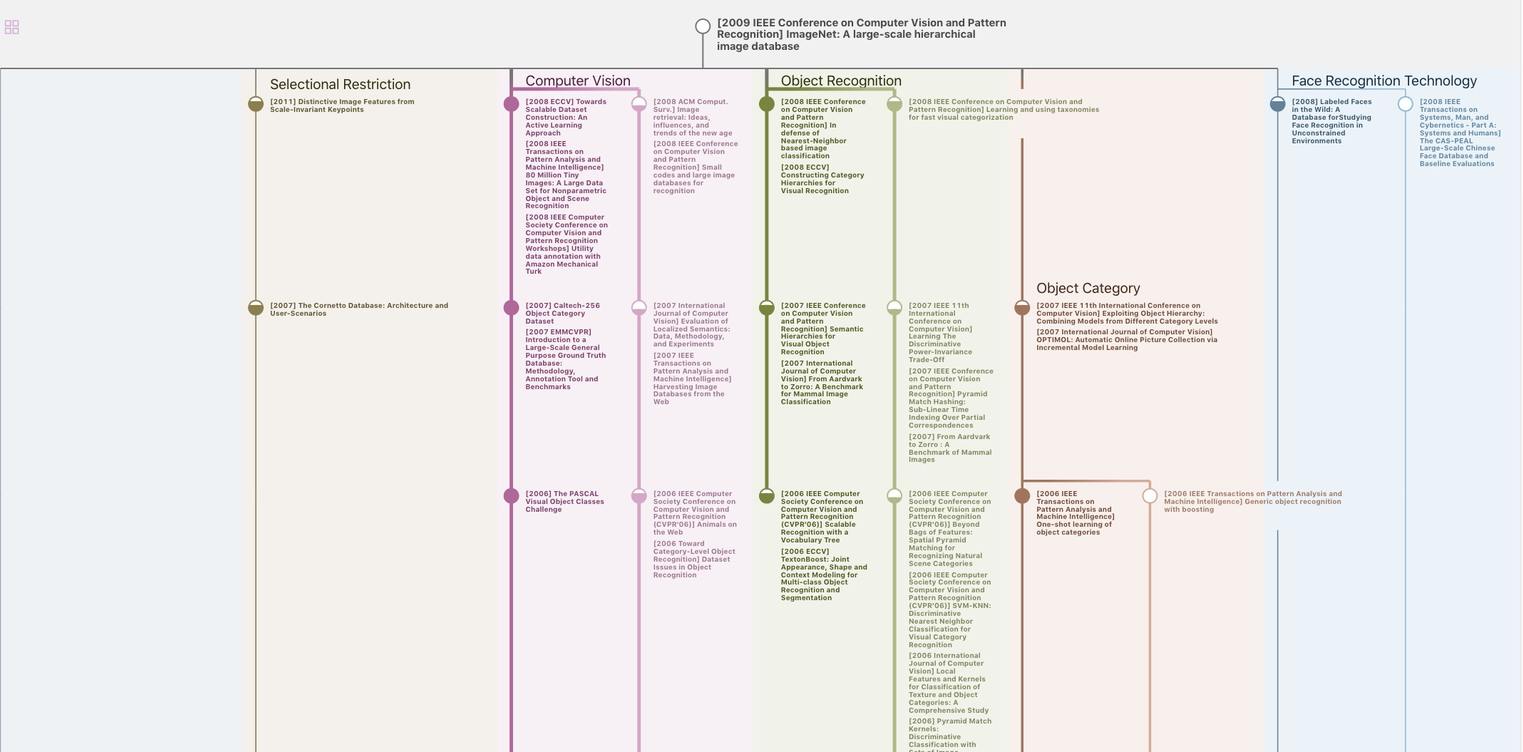
生成溯源树,研究论文发展脉络
Chat Paper
正在生成论文摘要