SLICT: Multi-input Multi-scale Surfel-Based Lidar-Inertial Continuous-Time Odometry and Mapping
arXiv (Cornell University)(2022)
摘要
While feature association to a global map has significant benefits, to keep the computations from growing exponentially, most lidar-based odometry and mapping methods opt to associate features with local maps at one voxel scale. Taking advantage of the fact that surfels (surface elements) at different voxel scales can be organized in a tree-like structure, we propose an octree-based global map of multi-scale surfels that can be updated incrementally. This alleviates the need for recalculating, for example, a k-d tree of the whole map repeatedly. The system can also take input from a single or a number of sensors, reinforcing the robustness in degenerate cases. We also propose a point-to-surfel (PTS) association scheme, continuous-time optimization on PTS and IMU preintegration factors, along with loop closure and bundle adjustment, making a complete framework for Lidar-Inertial continuous-time odometry and mapping. Experiments on public and in-house datasets demonstrate the advantages of our system compared to other state-of-the-art methods. To benefit the community, we release the source code and dataset at https://github.com/brytsknguyen/slict.
更多查看译文
关键词
mapping,multi-input,multi-scale,surfel-based,lidar-inertial,continuous-time
AI 理解论文
溯源树
样例
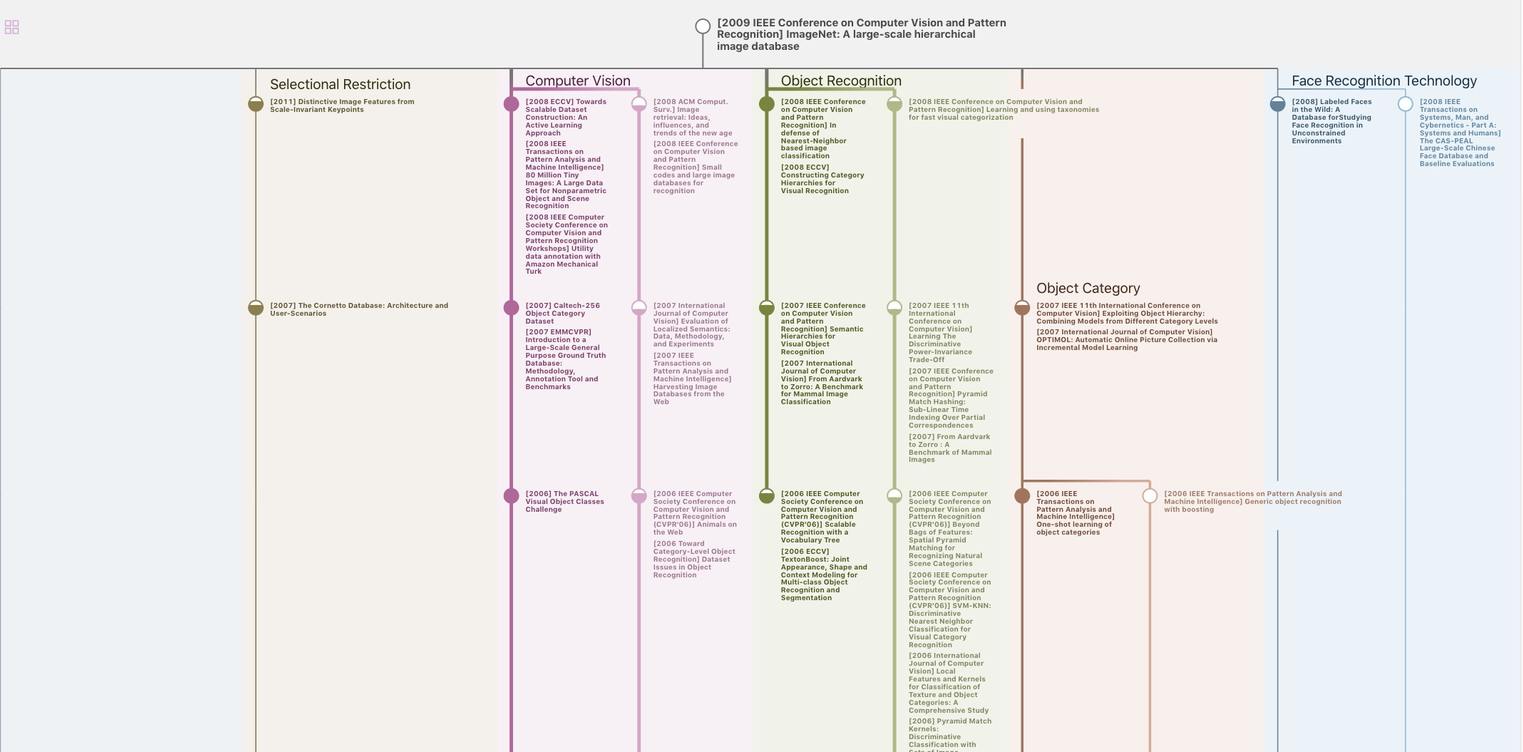
生成溯源树,研究论文发展脉络
Chat Paper
正在生成论文摘要