A Distributed Block-Split Gibbs Sampler with Hypergraph Structure for High-Dimensional Inverse Problems
JOURNAL OF COMPUTATIONAL AND GRAPHICAL STATISTICS(2023)
摘要
Sampling-based algorithms are classical approaches to perform Bayesian inference in inverse problems. They provide estimators with the associated credibility intervals to quantify the uncertainty on the estimators. Although these methods hardly scale to high dimensional problems, they have recently been paired with optimization techniques, such as proximal and splitting approaches, to address this issue. Such approaches pave the way to distributed samplers, splitting computations to make inference more scalable and faster. We introduce a distributed Split Gibbs sampler (SGS) to efficiently solve such problems involving distributions with multiple smooth and non-smooth functions composed with linear operators. The proposed approach leverages a recent approximate augmentation technique reminiscent of primal-dual optimization methods. It is further combined with a block-coordinate approach to split the primal and dual variables into blocks, leading to a distributed block-coordinate SGS. The resulting algorithm exploits the hypergraph structure of the involved linear operators to efficiently distribute the variables over multiple workers under controlled communication costs. It accommodates several distributed architectures, such as the Single Program Multiple Data and client-server architectures. Experiments on a large image deblurring problem show the performance of the proposed approach to produce high quality estimates with credibility intervals in a small amount of time. Supplementary material to reproduce the experiments is available online.
更多查看译文
关键词
Bayesian inference,Block-coordinate algorithm,Distributed architecture,High dimensional imaging inverse problems,MCMC algorithm
AI 理解论文
溯源树
样例
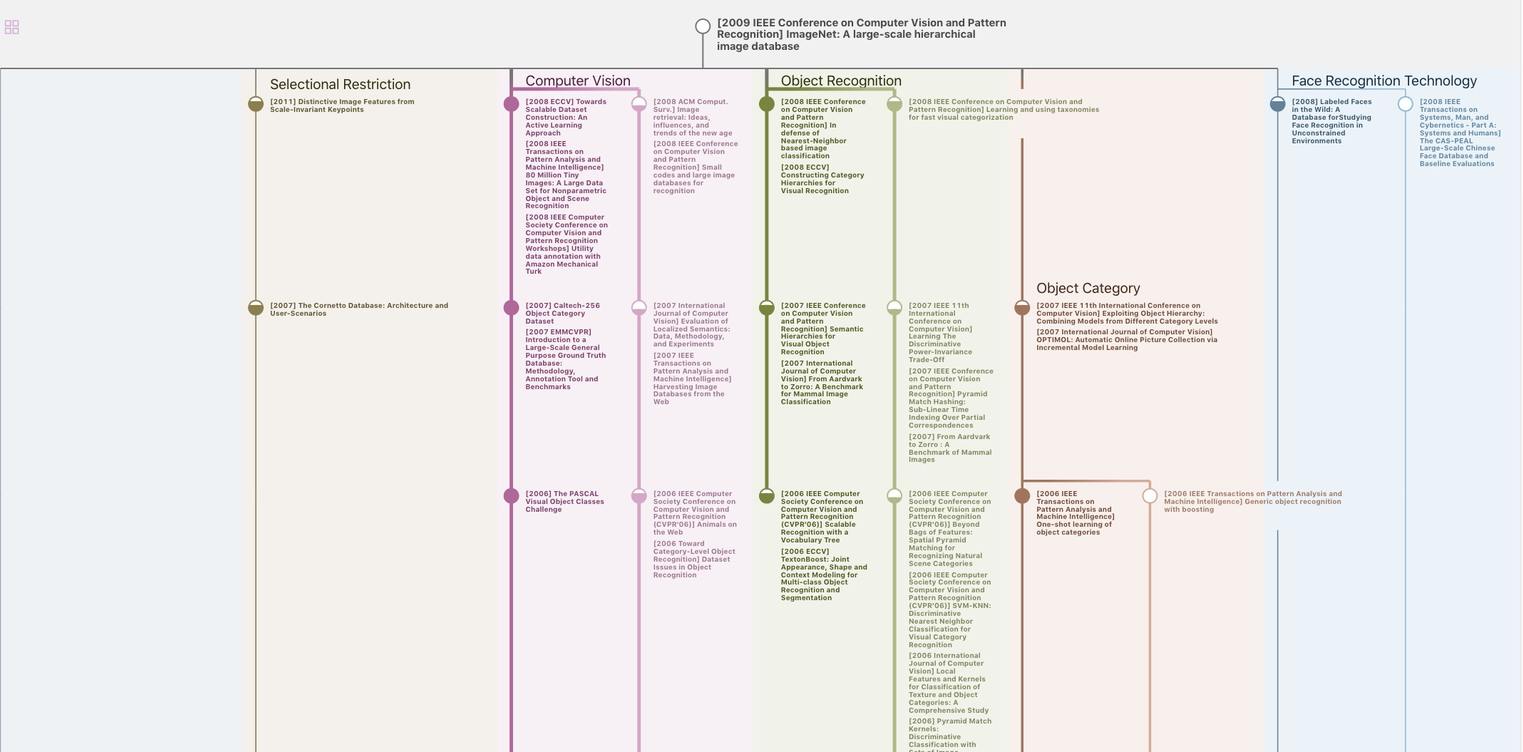
生成溯源树,研究论文发展脉络
Chat Paper
正在生成论文摘要