Learning an Efficient Multimodal Depth Completion Model
arXiv (Cornell University)(2022)
摘要
With the wide application of sparse ToF sensors in mobile devices, RGB image-guided sparse depth completion has attracted extensive attention recently, but still faces some problems. First, the fusion of multimodal information requires more network modules to process different modalities. But the application scenarios of sparse ToF measurements usually demand lightweight structure and low computational cost. Second, fusing sparse and noisy depth data with dense pixel-wise RGB data may introduce artifacts. In this paper, a light but efficient depth completion network is proposed, which consists of a two-branch global and local depth prediction module and a funnel convolutional spatial propagation network. The two-branch structure extracts and fuses cross-modal features with lightweight backbones. The improved spatial propagation module can refine the completed depth map gradually. Furthermore, corrected gradient loss is presented for the depth completion problem. Experimental results demonstrate the proposed method can outperform some state-of-the-art methods with a lightweight architecture. The proposed method also wins the championship in the MIPI2022 RGB+TOF depth completion challenge.
更多查看译文
关键词
depth,learning,model
AI 理解论文
溯源树
样例
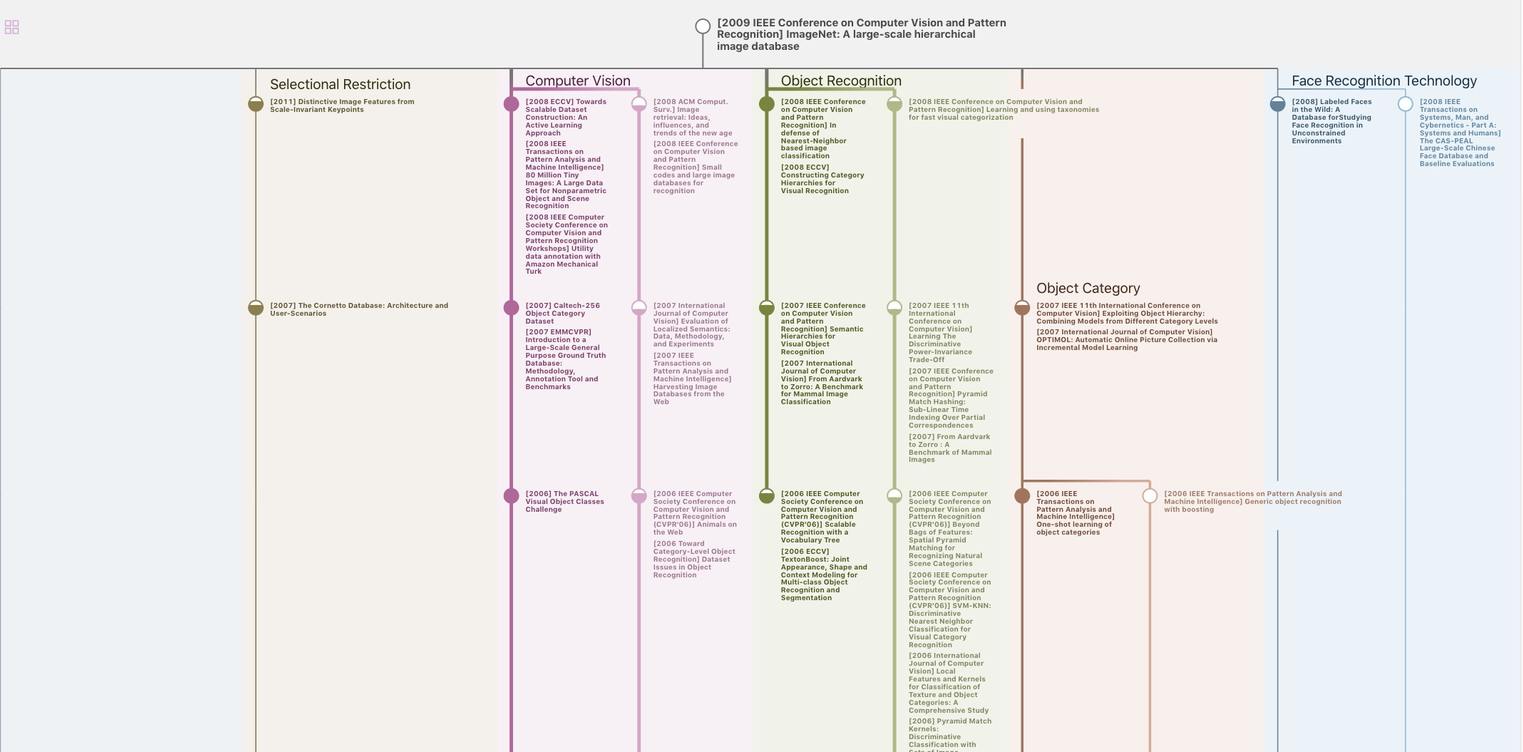
生成溯源树,研究论文发展脉络
Chat Paper
正在生成论文摘要