But That's Not Why: Inference Adjustment by Interactive Prototype Revision
PROGRESS IN PATTERN RECOGNITION, IMAGE ANALYSIS, COMPUTER VISION, AND APPLICATIONS, CIARP 2023, PT I(2024)
摘要
Prototypical part networks predict not only the class of an image but also explain why it was chosen. In some cases, however, the detected features do not relate to the depicted objects. This is especially relevant in prototypical part networks as prototypes are meant to code for high-level concepts such as semantic parts of objects. This raises the question how the inference of the networks can be improved. Here we suggest to enable the user to give hints and interactively correct the model's reasoning. It shows that even correct classifications can rely on unreasonable or spurious prototypes that result from confounding variables in a dataset. Hence, we propose simple yet effective interaction schemes for inference adjustment that enable the user to interactively revise the prototypes chosen by the model. Spurious prototypes can be removed or altered to become sensitive to object-features by the suggested mode of training. Interactive prototype revision allows machine learning naive users to adjust the logic of reasoning and change the way prototypical part networks make a decision.
更多查看译文
关键词
Prototype Learning,human-AI-Interaction,interactive ML,deep neural networks,inference correction
AI 理解论文
溯源树
样例
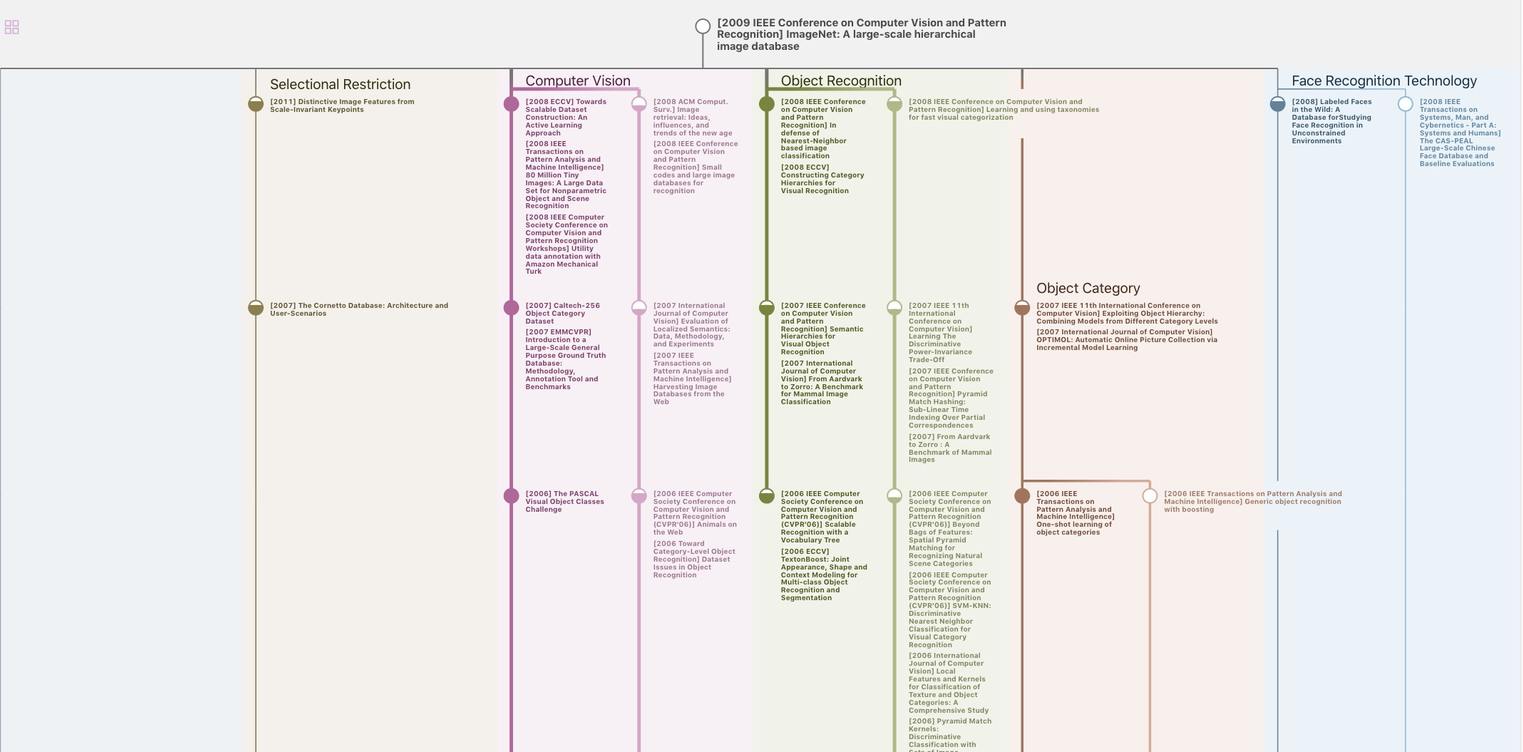
生成溯源树,研究论文发展脉络
Chat Paper
正在生成论文摘要