Iteratively Prompt Pre-trained Language Models for Chain of Thought
arXiv (Cornell University)(2022)
摘要
While Pre-trained Language Models (PLMs) internalize a great amount of world knowledge, they have been shown incapable of recalling these knowledge to solve tasks requiring complex & multi-step reasoning. Similar to how humans develop a "chain of thought" for these tasks, how can we equip PLMs with such abilities? In this work, we explore an iterative prompting framework, a new prompting paradigm which progressively elicits relevant knowledge from PLMs for multi-step inference. We identify key limitations of existing prompting methods, namely they are either restricted to queries with a single identifiable relation/predicate, or being agnostic to input contexts, which makes it difficult to capture variabilities across different inference steps. We propose an iterative context-aware prompter, which addresses these limitations by learning to dynamically synthesize prompts conditioned on the current step's contexts. Experiments on three datasets involving multi-step reasoning show the effectiveness of the iterative scheme and the context-aware prompter design.
更多查看译文
关键词
language models,thought,pre-trained
AI 理解论文
溯源树
样例
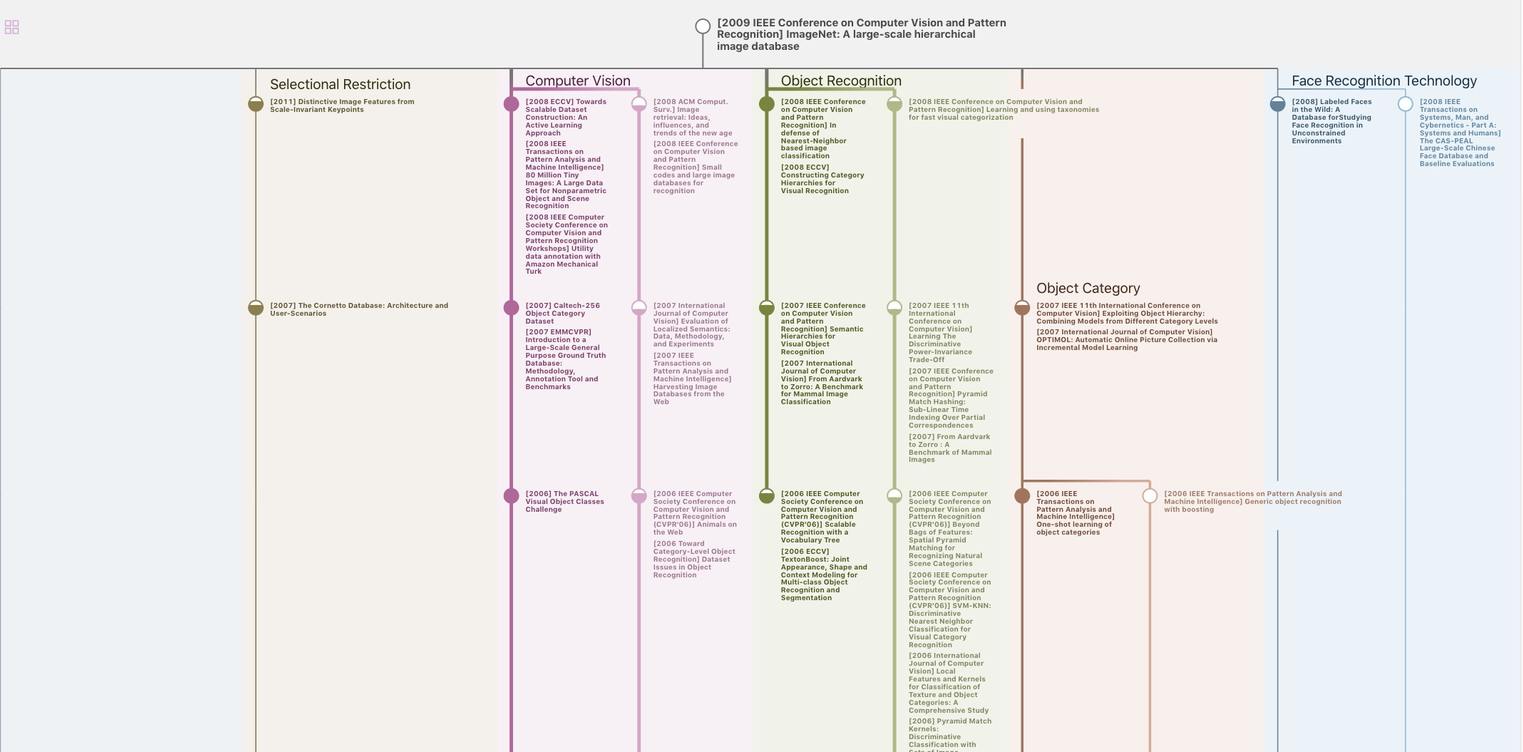
生成溯源树,研究论文发展脉络
Chat Paper
正在生成论文摘要