Machine Learning in Aerodynamic Shape Optimization
arXiv (Cornell University)(2022)
摘要
Large volumes of experimental and simulation aerodynamic data have been rapidly advancing aerodynamic shape optimization (ASO) via machine learning (ML), whose effectiveness has been growing thanks to continued developments in deep learning. In this review, we first introduce the state of the art and the unsolved challenges in ASO. Next, we present a description of ML fundamentals and detail the ML algorithms that have succeeded in ASO. Then we review ML applications contributing to ASO from three fundamental perspectives: compact geometric design space, fast aerodynamic analysis, and efficient optimization architecture. In addition to providing a comprehensive summary of the research, we comment on the practicality and effectiveness of the developed methods. We show how cutting-edge ML approaches can benefit ASO and address challenging demands like interactive design optimization. However, practical large-scale design optimizations remain a challenge due to the costly ML training expense. A deep coupling of ML model construction with ASO prior experience and knowledge, such as taking physics into account, is recommended to train ML models effectively.
更多查看译文
关键词
aerodynamic shape optimization,machine learning
AI 理解论文
溯源树
样例
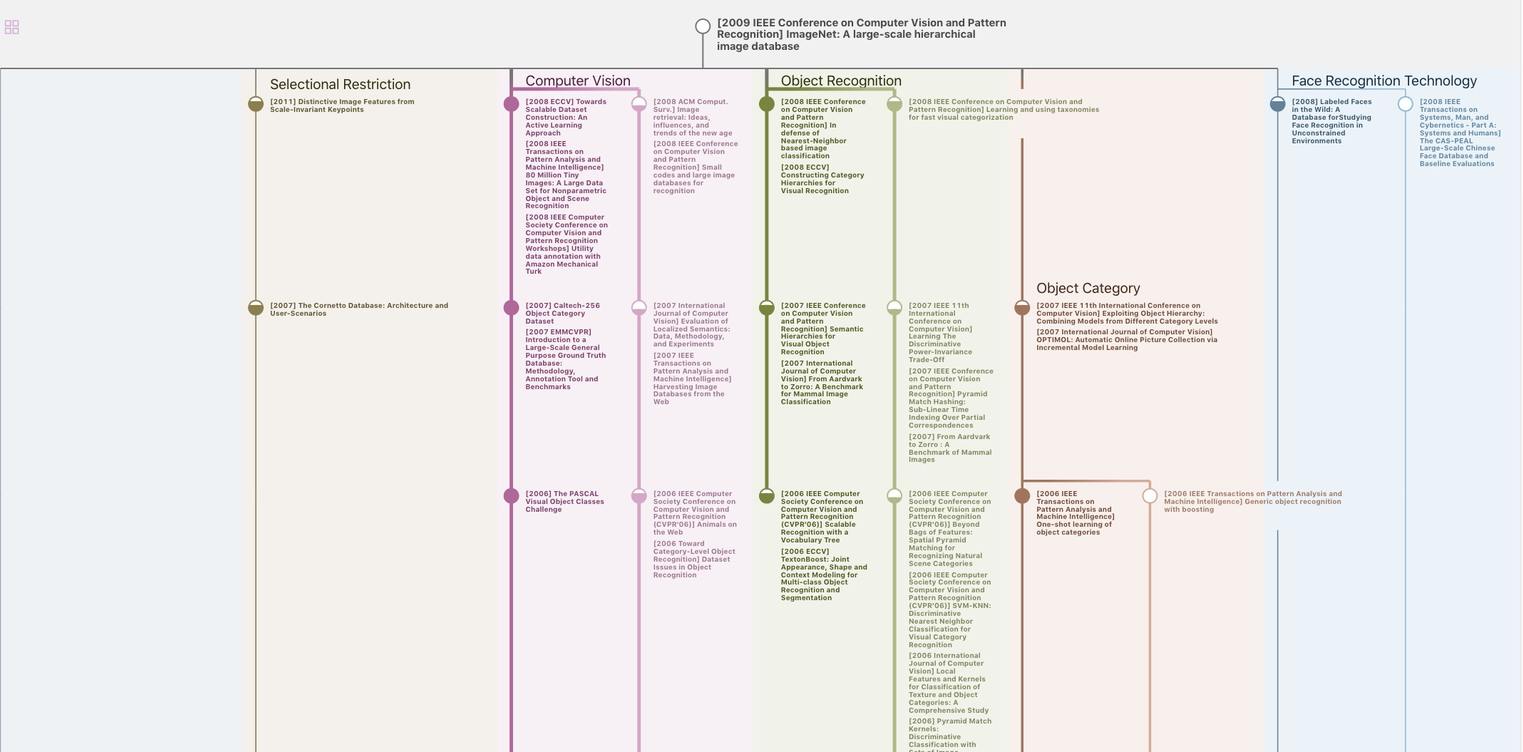
生成溯源树,研究论文发展脉络
Chat Paper
正在生成论文摘要