Simple Emergent Action Representations from Multi-Task Policy Training
arXiv (Cornell University)(2022)
摘要
The low-level sensory and motor signals in deep reinforcement learning, which exist in high-dimensional spaces such as image observations or motor torques, are inherently challenging to understand or utilize directly for downstream tasks. While sensory representations have been extensively studied, the representations of motor actions are still an area of active exploration. Our work reveals that a space containing meaningful action representations emerges when a multi-task policy network takes as inputs both states and task embeddings. Moderate constraints are added to improve its representation ability. Therefore, interpolated or composed embeddings can function as a high-level interface within this space, providing instructions to the agent for executing meaningful action sequences. Empirical results demonstrate that the proposed action representations are effective for intra-action interpolation and inter-action composition with limited or no additional learning. Furthermore, our approach exhibits superior task adaptation ability compared to strong baselines in Mujoco locomotion tasks. Our work sheds light on the promising direction of learning action representations for efficient, adaptable, and composable RL, forming the basis of abstract action planning and the understanding of motor signal space. Project page: https://sites.google.com/view/emergent-action-representation/
更多查看译文
关键词
action,policy,training,multi-task
AI 理解论文
溯源树
样例
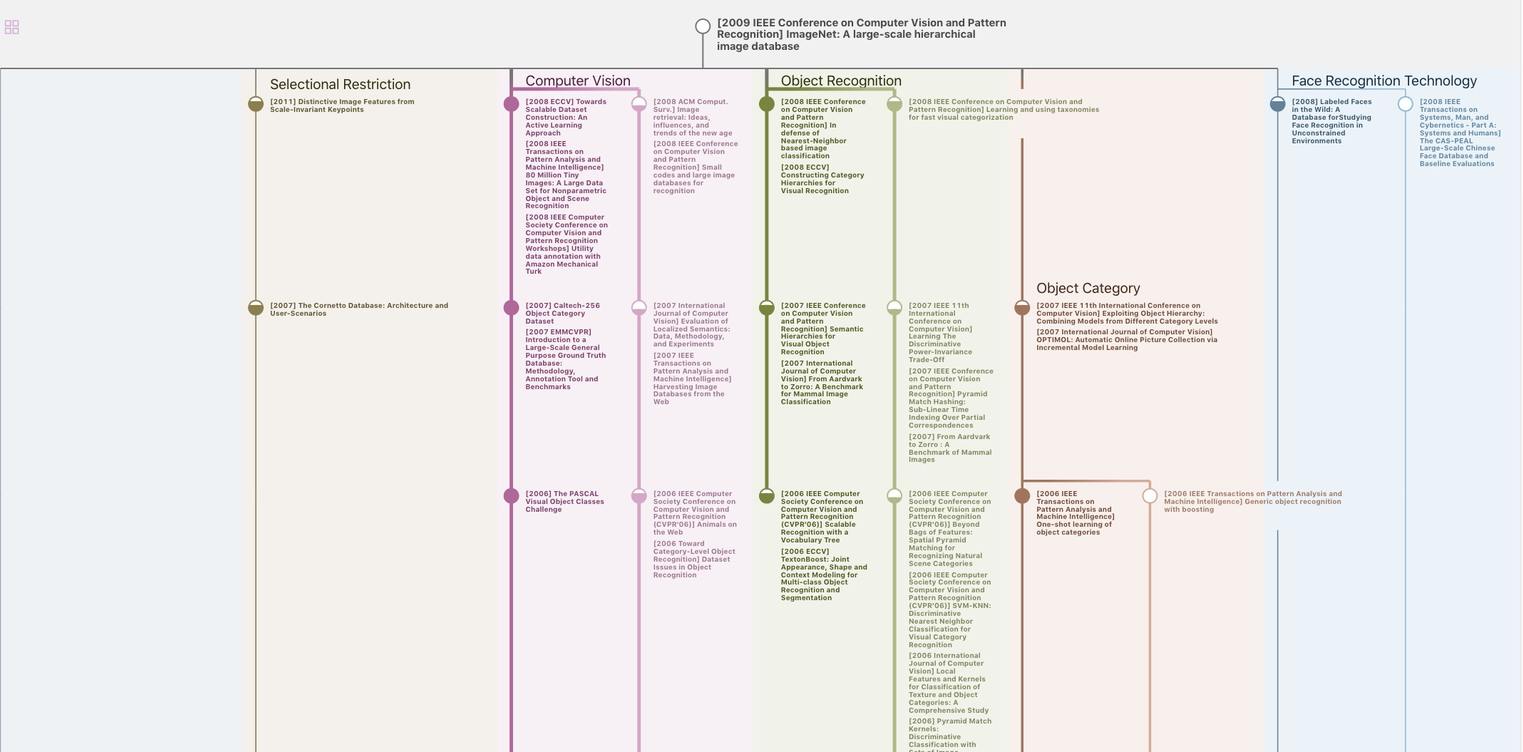
生成溯源树,研究论文发展脉络
Chat Paper
正在生成论文摘要