Federated Learning for Tabular Data: Exploring Potential Risk to Privacy
arXiv (Cornell University)(2022)
摘要
Federated Learning (FL) has emerged as a potentially powerful privacy-preserving machine learning methodology, since it avoids exchanging data between participants, but instead exchanges model parameters. FL has traditionally been applied to image, voice and similar data, but recently it has started to draw attention from domains including financial services where the data is predominantly tabular. However, the work on tabular data has not yet considered potential attacks, in particular attacks using Generative Adversarial Networks (GANs), which have been successfully applied to FL for non-tabular data. This paper is the first to explore leakage of private data in Federated Learning systems that process tabular data. We design a Generative Adversarial Networks (GANs)-based attack model which can be deployed on a malicious client to reconstruct data and its properties from other participants. As a side-effect of considering tabular data, we are able to statistically assess the efficacy of the attack (without relying on human observation such as done for FL for images). We implement our attack model in a recently developed generic FL software framework for tabular data processing. The experimental results demonstrate the effectiveness of the proposed attack model, thus suggesting that further research is required to counter GAN-based privacy attacks.
更多查看译文
关键词
privacy,tabular data,learning
AI 理解论文
溯源树
样例
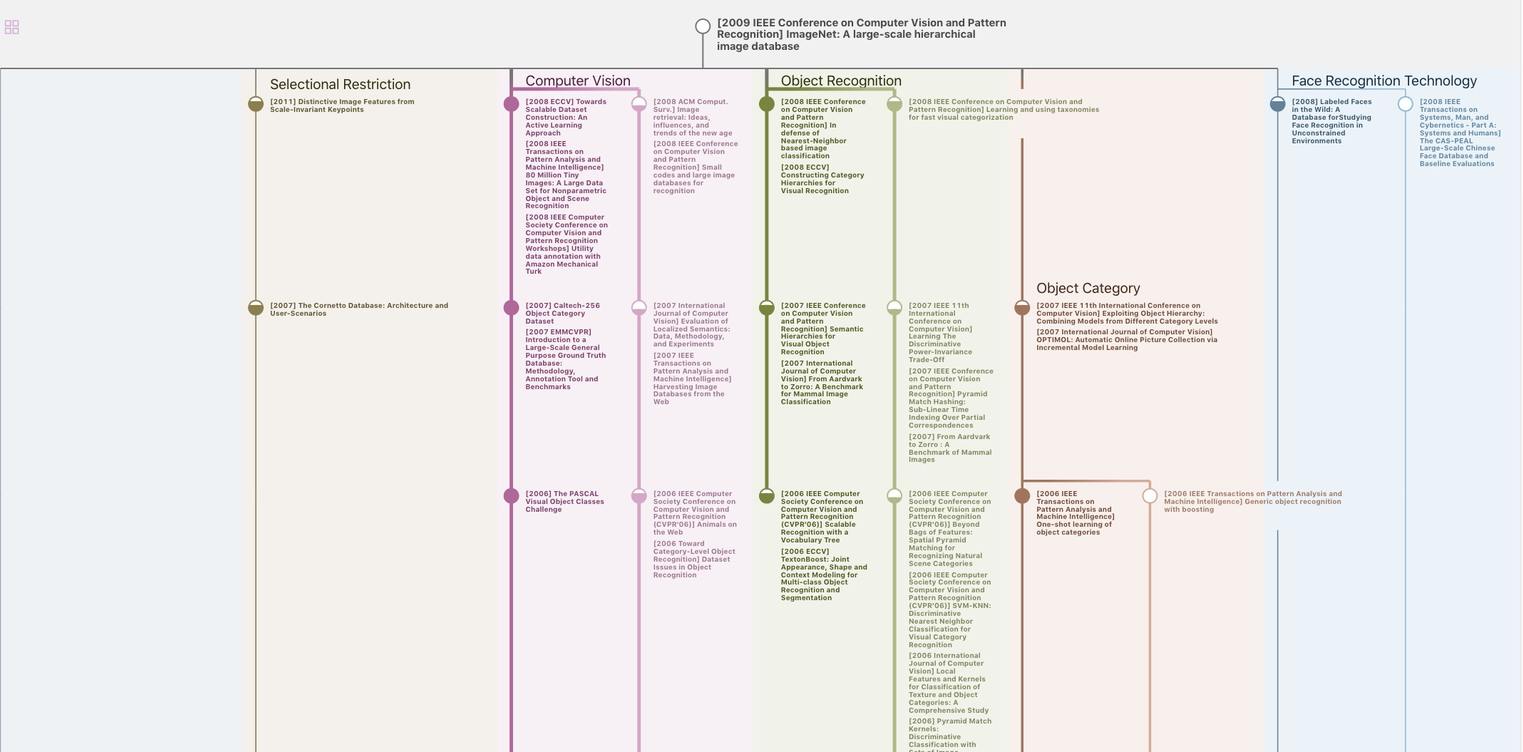
生成溯源树,研究论文发展脉络
Chat Paper
正在生成论文摘要