Integrated Artificial Neural Network with Trainable Activation Function Enabled by Topological Insulator-based Spin-Orbit Torque Devices
arXiv (Cornell University)(2022)
摘要
Non-volatile memristors offer a salient platform for artificial neural network (ANN), but the integration of different function blocks into one hardware system remains challenging. Here we demonstrate the implementation of brain-like synaptic (SOT-S) and neuronal (SOT-N) functions in the Bi2Te3/CrTe2 heterostructure-based spin-orbit torque (SOT) device. The SOT-S unit exhibits highly linear (linearity error < 4.19%) and symmetrical long-term potentiation/depression process, resulting in better performance compared to other memristor synapses. Meanwhile, the Sigmoid-shape transition curve inherited in the SOT-N cell replaces the software-based activation function block, hence reducing the system complexity. On this basis, we employ a serial-connected, voltage-mode sensing ANN architecture to enhance the vector-matrix multiplication signal strength with low reading error of 0.61%. Furthermore, the trainable activation function of SOT-N enables the integrated SOT-ANN to execute the Batch Normalization algorithm and activation operation within one clock cycle, which bring about improved on/off-chip training performance close to the ideal baseline.
更多查看译文
关键词
trainable activation function
AI 理解论文
溯源树
样例
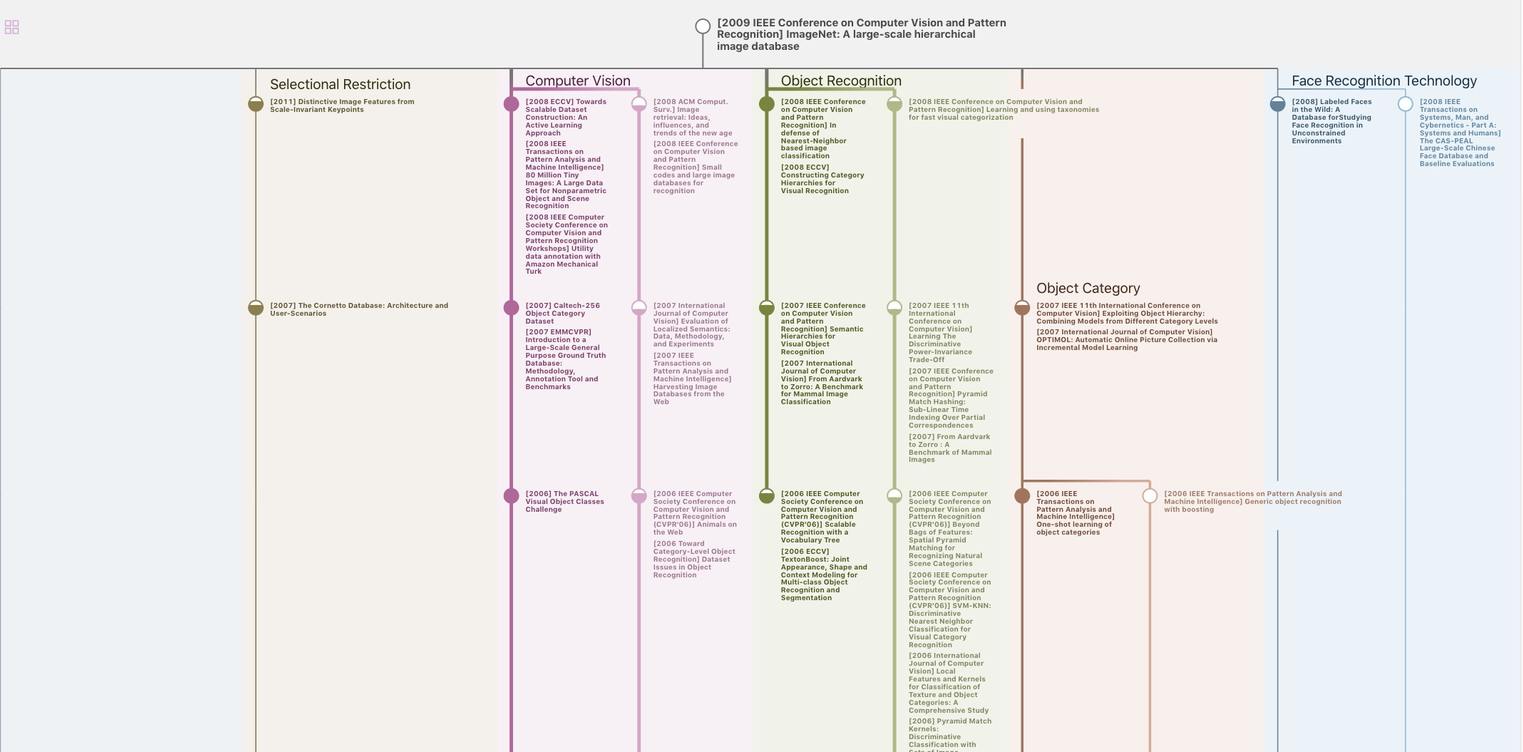
生成溯源树,研究论文发展脉络
Chat Paper
正在生成论文摘要