Open Vocabulary Multi-Label Classification with Dual-Modal Decoder on Aligned Visual-Textual Features
arXiv (Cornell University)(2022)
摘要
In computer vision, multi-label recognition are important tasks with many real-world applications, but classifying previously unseen labels remains a significant challenge. In this paper, we propose a novel algorithm, Aligned Dual moDality ClaSsifier (ADDS), which includes a Dual-Modal decoder (DM-decoder) with alignment between visual and textual features, for open-vocabulary multi-label classification tasks. Then we design a simple and yet effective method called Pyramid-Forwarding to enhance the performance for inputs with high resolutions. Moreover, the Selective Language Supervision is applied to further enhance the model performance. Extensive experiments conducted on several standard benchmarks, NUS-WIDE, ImageNet-1k, ImageNet-21k, and MS-COCO, demonstrate that our approach significantly outperforms previous methods and provides state-of-the-art performance for open-vocabulary multi-label classification, conventional multi-label classification and an extreme case called single-to-multi label classification where models trained on single-label datasets (ImageNet-1k, ImageNet-21k) are tested on multi-label ones (MS-COCO and NUS-WIDE).
更多查看译文
关键词
classification,multi-label,dual-modal,visual-textual
AI 理解论文
溯源树
样例
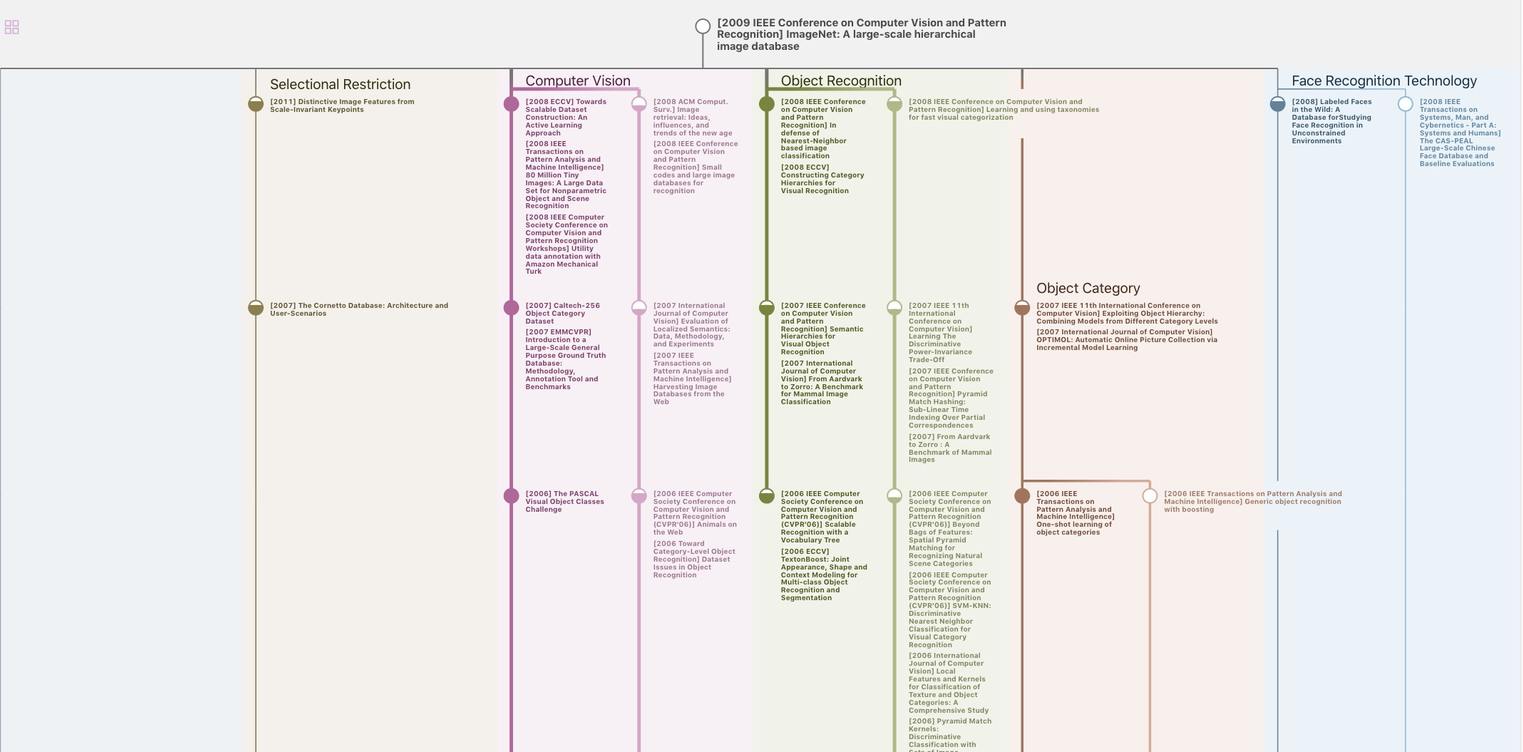
生成溯源树,研究论文发展脉络
Chat Paper
正在生成论文摘要