Predicting patient treatment response and resistance via single-cell transcriptomics of their tumors
Research Square (Research Square)(2022)
摘要
Abstract Tailoring the best treatments for individual cancer patients is an important open challenge. Here, we build a precision oncology computational pipeline for PERsonalized single-Cell Expression-based Planning for Treatments In ONcology (PERCEPTION). Our approach capitalizes on recently published matched bulk and single-cell (SC) transcriptome profiles of large-scale cell-line drug screens to build treatment response models from patients' SC tumor transcriptomics. We start by showing that PERCEPTION successfully predicts the response to monotherapy and combination treatments in screens performed in cancer and patient-tumor-derived primary cells based on their SC-expression profiles. Our key result is that PERCEPTION successfully stratifies responders to combination therapy based on the patients’ tumor’s SC-expression, as tested in two recently published clinical trials, including multiple myeloma and breast cancer. Thirdly, studying the emergence of resistance via a recent SC non-small cell lung cancer (NSCLC) patients’ cohort, we show that PERCEPTION successfully captures and quantifies the development of patients’ resistance during treatment with tyrosine kinase inhibitors. Notably, PERCEPTION predictions markedly outperform that of bulk expression-based predictors in all these cohorts. In sum, this study provides a first-of-its-kind conceptual and computational method demonstrating the feasibility of predicting patients' response from SC gene expression of their tumors.
更多查看译文
关键词
patient treatment response,tumors,resistance,single-cell
AI 理解论文
溯源树
样例
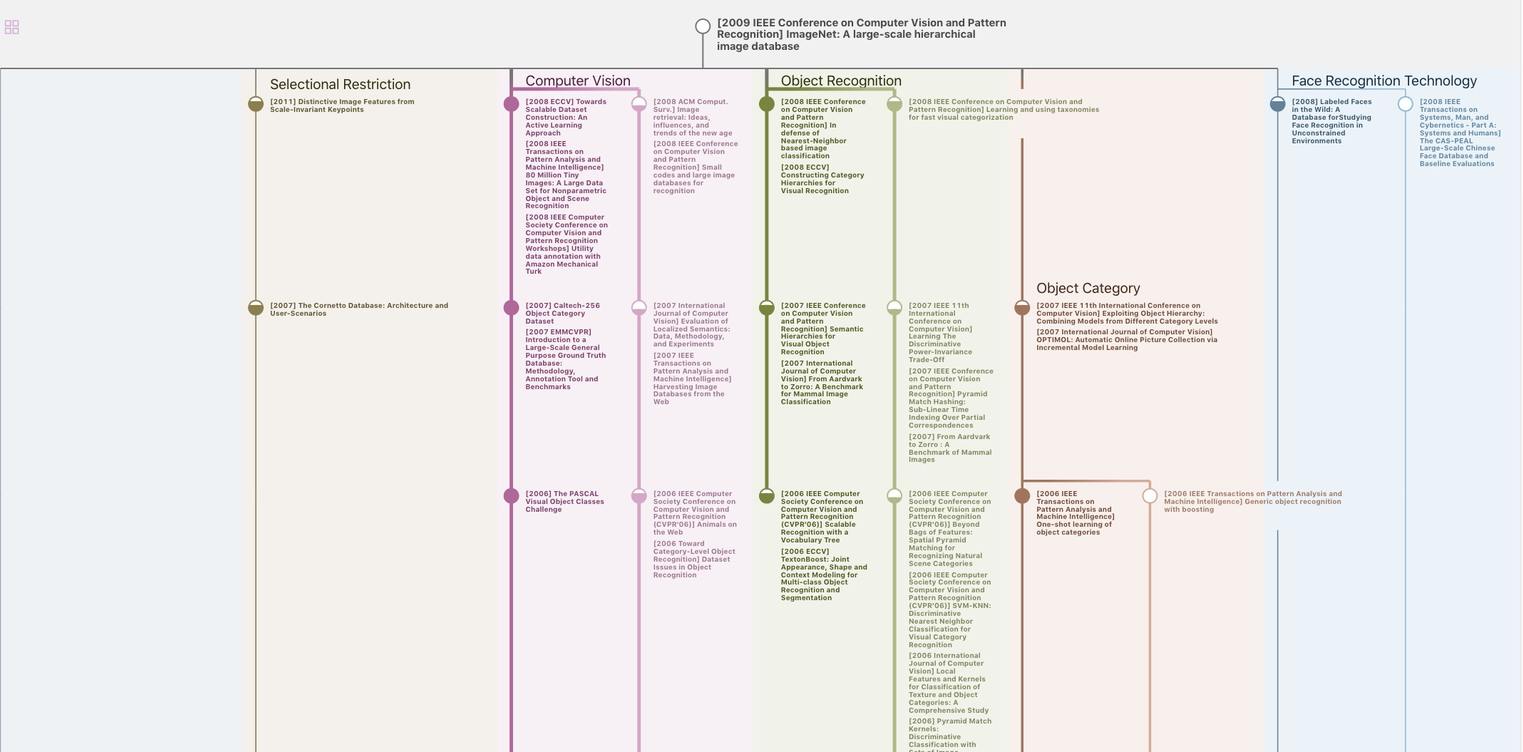
生成溯源树,研究论文发展脉络
Chat Paper
正在生成论文摘要