Seprox
Proceedings of the 41st IEEE/ACM International Conference on Computer-Aided Design(2022)
摘要
Compression techniques such as quantization and pruning are indispensable for deploying state-of-the-art Deep Neural Networks (DNNs) on resource-constrained edge devices. Quantization is widely used in practice - many commercial platforms already support 8-bits, with recent trends towards ultra-low precision (4-bits and below). Pruning, which increases network sparsity (incidence of zero-valued weights), enables compression by storing only the nonzero weights and their indices. Unfortunately, the compression benefits of pruning deteriorate or even vanish in ultra-low precision DNNs. This is due to (i) the unfavorable tradeoff between the number of bits needed to store a weight (which reduces with lower precision) and the number of bits needed to encode an index (which remains unchanged), and (ii) the lower sparsity levels that are achievable at lower precisions.
更多查看译文
AI 理解论文
溯源树
样例
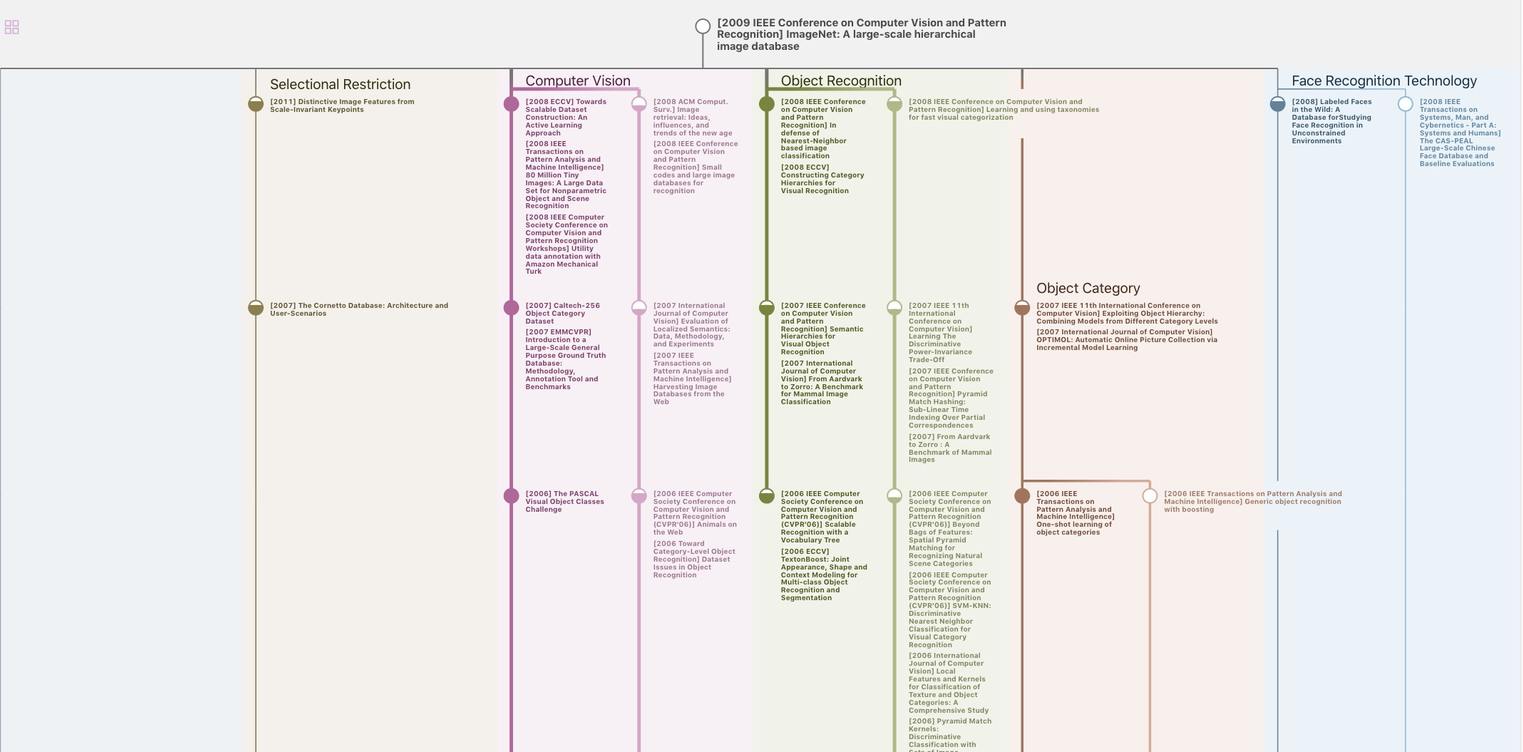
生成溯源树,研究论文发展脉络
Chat Paper
正在生成论文摘要