Upper Bounds for All and Max-gain Policy Iteration Algorithms on Deterministic MDPs
arXiv (Cornell University)(2022)
摘要
Policy Iteration (PI) is a widely used family of algorithms to compute optimal policies for Markov Decision Problems (MDPs). We derive upper bounds on the running time of PI on Deterministic MDPs (DMDPs): the class of MDPs in which every state-action pair has a unique next state. Our results include a non-trivial upper bound that applies to the entire family of PI algorithms; another to all "max-gain" switching variants; and affirmation that a conjecture regarding Howard's PI on MDPs is true for DMDPs. Our analysis is based on certain graph-theoretic results, which may be of independent interest.
更多查看译文
关键词
policy iteration algorithms,deterministic mdps,max-gain
AI 理解论文
溯源树
样例
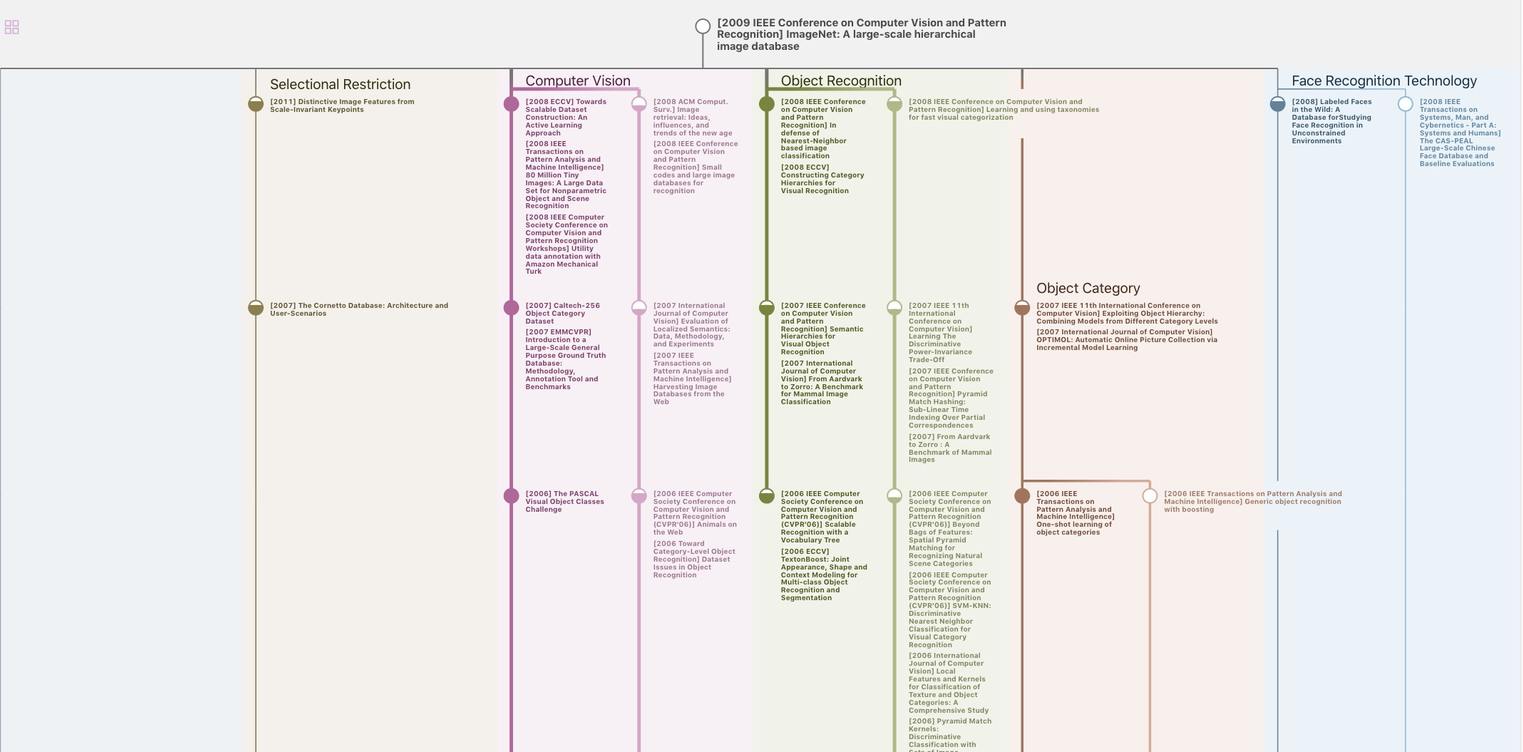
生成溯源树,研究论文发展脉络
Chat Paper
正在生成论文摘要