A Unified Framework for Analyzing and Optimizing a Class of Convex Inequity Measures
arXiv (Cornell University)(2022)
摘要
We propose a new unified framework for analyzing a new parameterized class of convex inequity measures suitable for optimization contexts. First, we propose a new class of order-based inequity measures, discuss their properties, and derive axiomatic characterizations for such measures. Then, we introduce our proposed class of convex inequity measures, discuss their theoretical properties in an absolute and relative sense, and derive an equivalent dual representation of these measures as a robustified order-based inequity measure over their dual sets. Importantly, this dual representation renders a unified mathematical expression and an alternative geometric characterization for convex inequity measures through their dual sets. Using this representation, we propose a unified framework for optimization problems with a convex inequity measure objective or constraint, including reformulations and solution methods. Finally, we provide stability results that quantify the impact of employing different convex inequity measures on the optimal value and solution of the resulting optimization problem. Our numerical results demonstrate the computational efficiency of our proposed framework over existing approaches.
更多查看译文
关键词
measures,convex
AI 理解论文
溯源树
样例
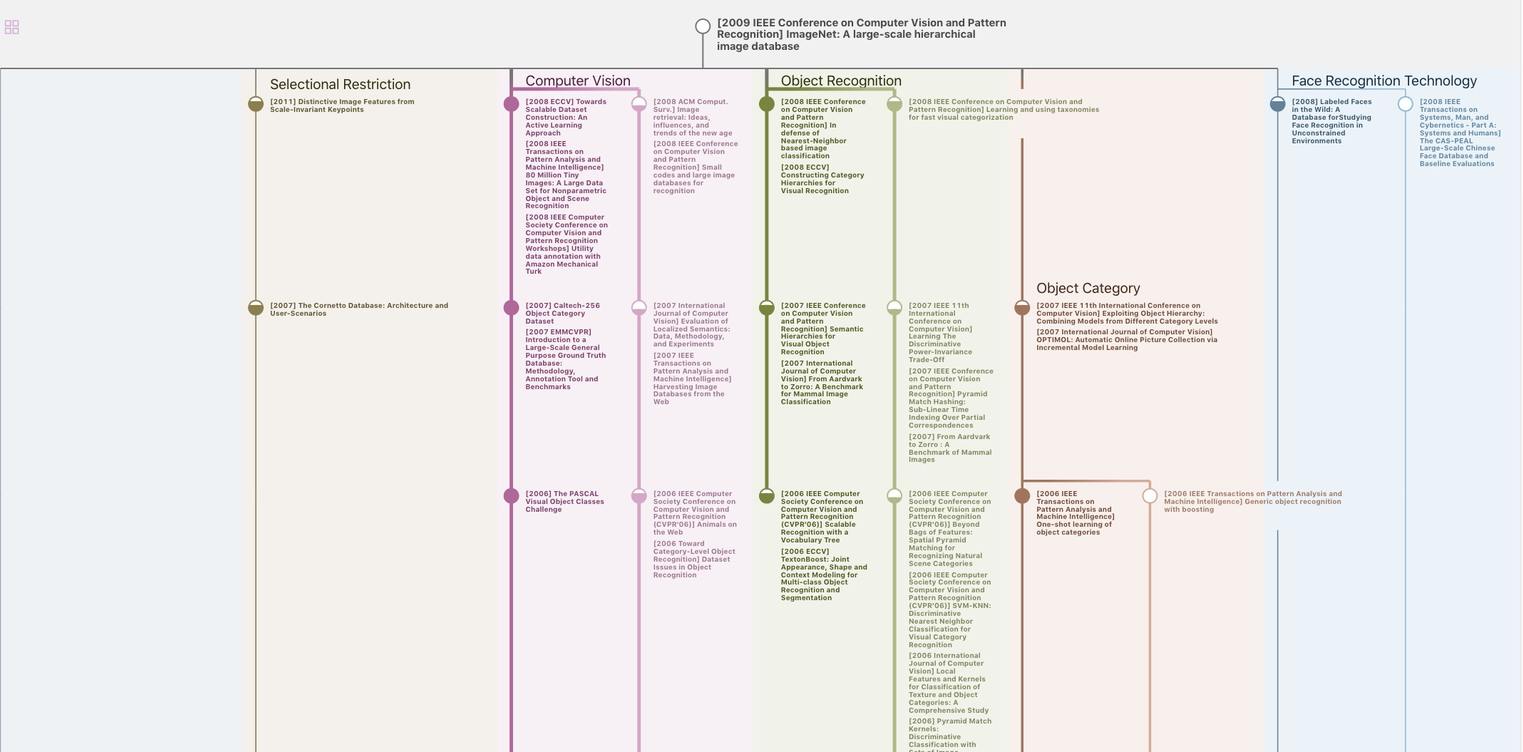
生成溯源树,研究论文发展脉络
Chat Paper
正在生成论文摘要