Combining Machine Learning and Spectroscopy to Model Reactive Atom + Diatom Collisions
arXiv (Cornell University)(2022)
摘要
The prediction of product translational, vibrational, and rotational energy distributions for arbitrary initial conditions for reactive atom+diatom collisions is of considerable practical interest in atmospheric re-entry. Due to the large number of accessible states, determination of the necessary information from explicit (quasi-classical or quantum) dynamics studies is impractical. Here, a machine-learned (ML) model based on translational energy and product vibrational states assigned from a spectroscopic, ro-vibrational coupled energy expression based on the Dunham expansion is developed and tested quantitatively. All models considered in this work reproduce final state distributions determined from quasi-classical trajectory (QCT) simulations with $R^2 \sim 0.98$. As a further validation, thermal rates determined from the machine-learned models agree with those from explicit QCT simulations and demonstrate that the atomistic details are retained by the machine learning which makes them suitable for applications in more coarse-grained simulations. More generally, it is found that ML is suitable for designing robust and accurate models from mixed computational/experimental data which may also be of interest in other areas of the physical sciences.
更多查看译文
关键词
diatom collisions,spectroscopy,atom,machine learning
AI 理解论文
溯源树
样例
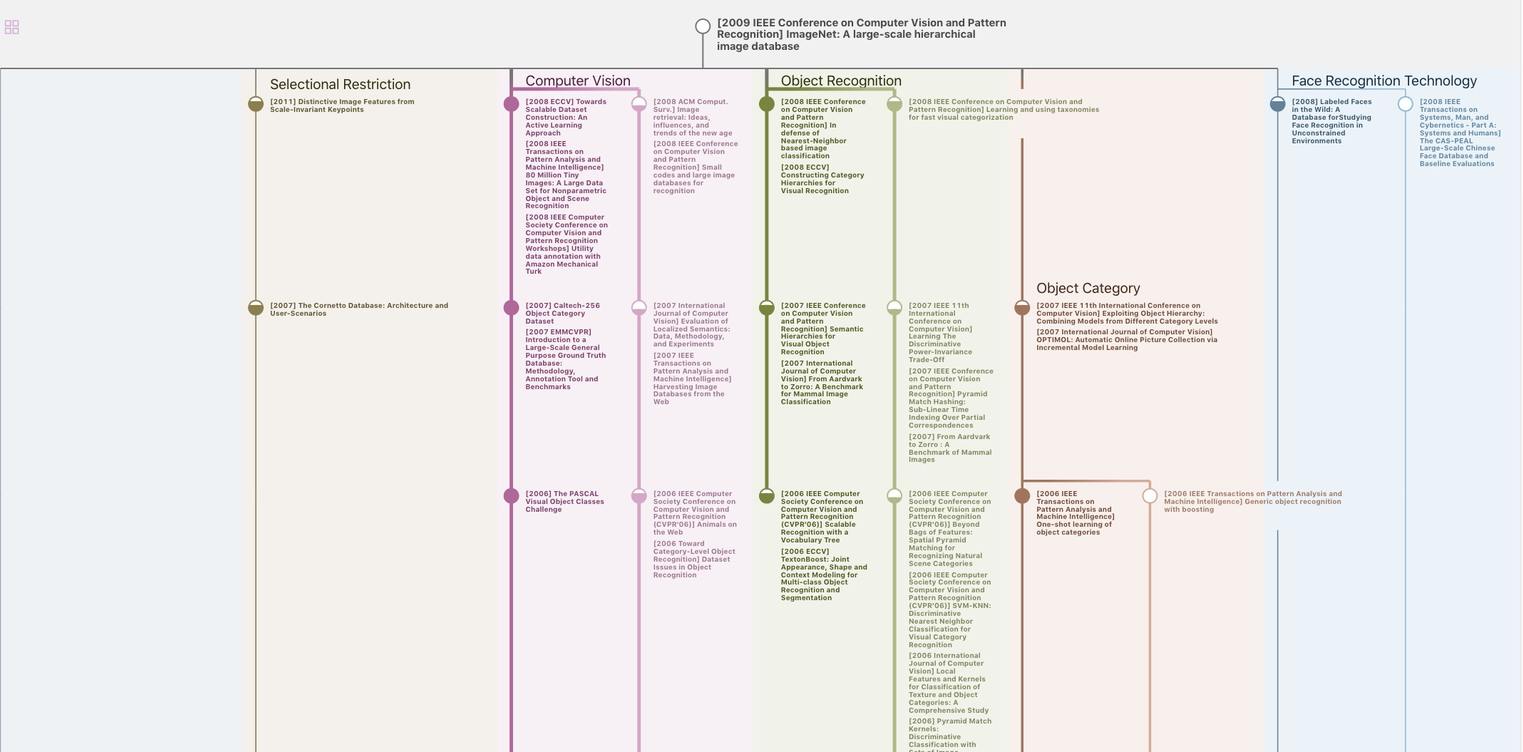
生成溯源树,研究论文发展脉络
Chat Paper
正在生成论文摘要