Model Compression for DNN-based Speaker Verification Using Weight Quantization
arXiv (Cornell University)(2022)
摘要
DNN-based speaker verification (SV) models demonstrate significant performance at relatively high computation costs. Model compression can be applied to reduce the model size for lower resource consumption. The present study exploits weight quantization to compress two widely-used SV models, namely ECAPA-TDNN and ResNet. Experimental results on VoxCeleb show that weight quantization is effective for compressing SV models. The model size can be reduced multiple times without noticeable degradation in performance. Compression of ResNet shows more robust results than ECAPA-TDNN with lower-bitwidth quantization. Analysis of the layer weights suggests that the smooth weight distribution of ResNet may be related to its better robustness. The generalization ability of the quantized model is validated via a language-mismatched SV task. Furthermore, analysis by information probing reveals that the quantized models can retain most of the speaker-relevant knowledge learned by the original models.
更多查看译文
关键词
speaker verification,quantization,dnn-based
AI 理解论文
溯源树
样例
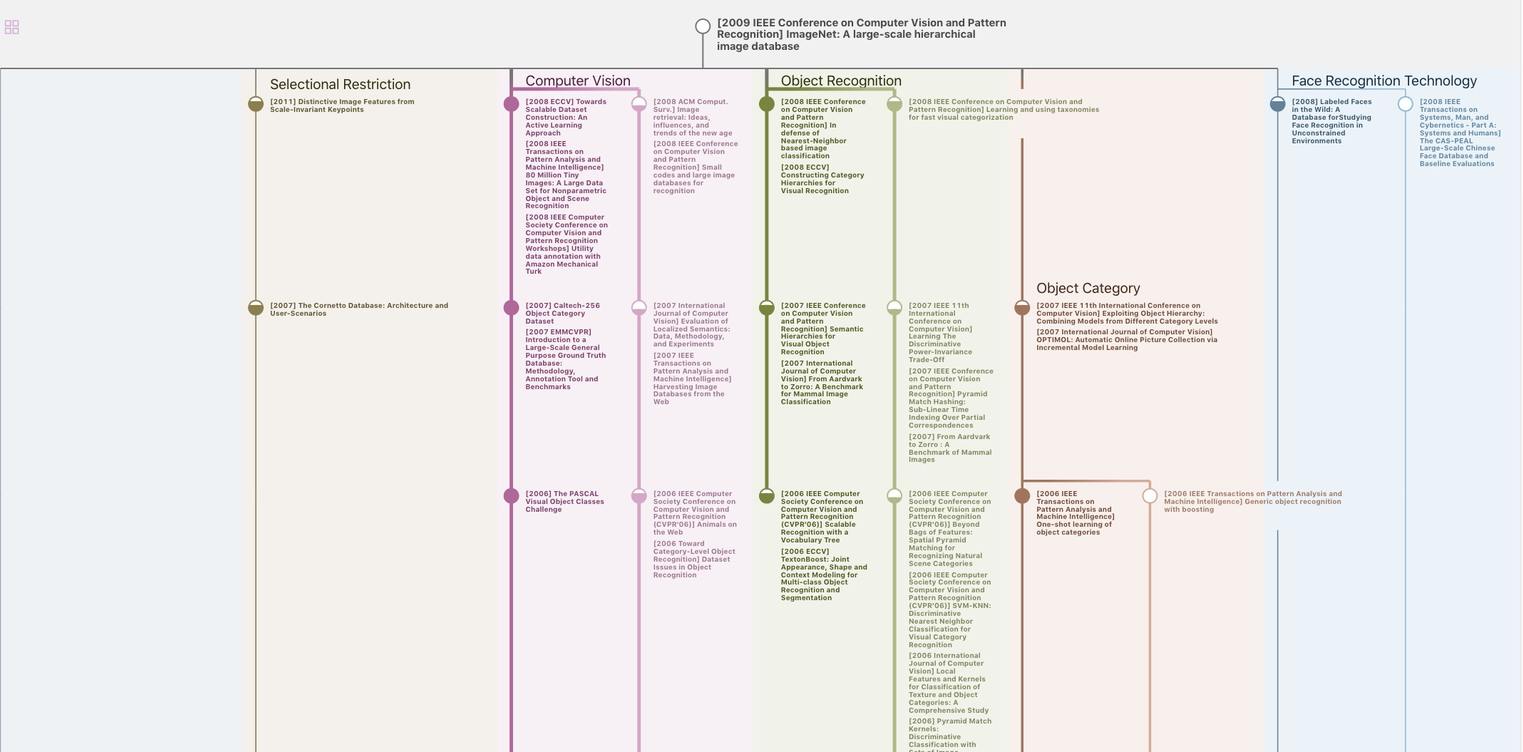
生成溯源树,研究论文发展脉络
Chat Paper
正在生成论文摘要