On Private Online Convex Optimization: Optimal Algorithms in $\ell_p$-Geometry and High Dimensional Contextual Bandits
arXiv (Cornell University)(2022)
摘要
Differentially private (DP) stochastic convex optimization (SCO) is ubiquitous in trustworthy machine learning algorithm design. This paper studies the DP-SCO problem with streaming data sampled from a distribution and arrives sequentially. We also consider the continual release model where parameters related to private information are updated and released upon each new data, often known as the online algorithms. Despite that numerous algorithms have been developed to achieve the optimal excess risks in different $\ell_p$ norm geometries, yet none of the existing ones can be adapted to the streaming and continual release setting. To address such a challenge as the online convex optimization with privacy protection, we propose a private variant of online Frank-Wolfe algorithm with recursive gradients for variance reduction to update and reveal the parameters upon each data. Combined with the adaptive differential privacy analysis, our online algorithm achieves in linear time the optimal excess risk when $1
更多
查看译文
关键词
private online convex optimization,high dimensional contextual bandits,optimal algorithms
AI 理解论文
溯源树
样例
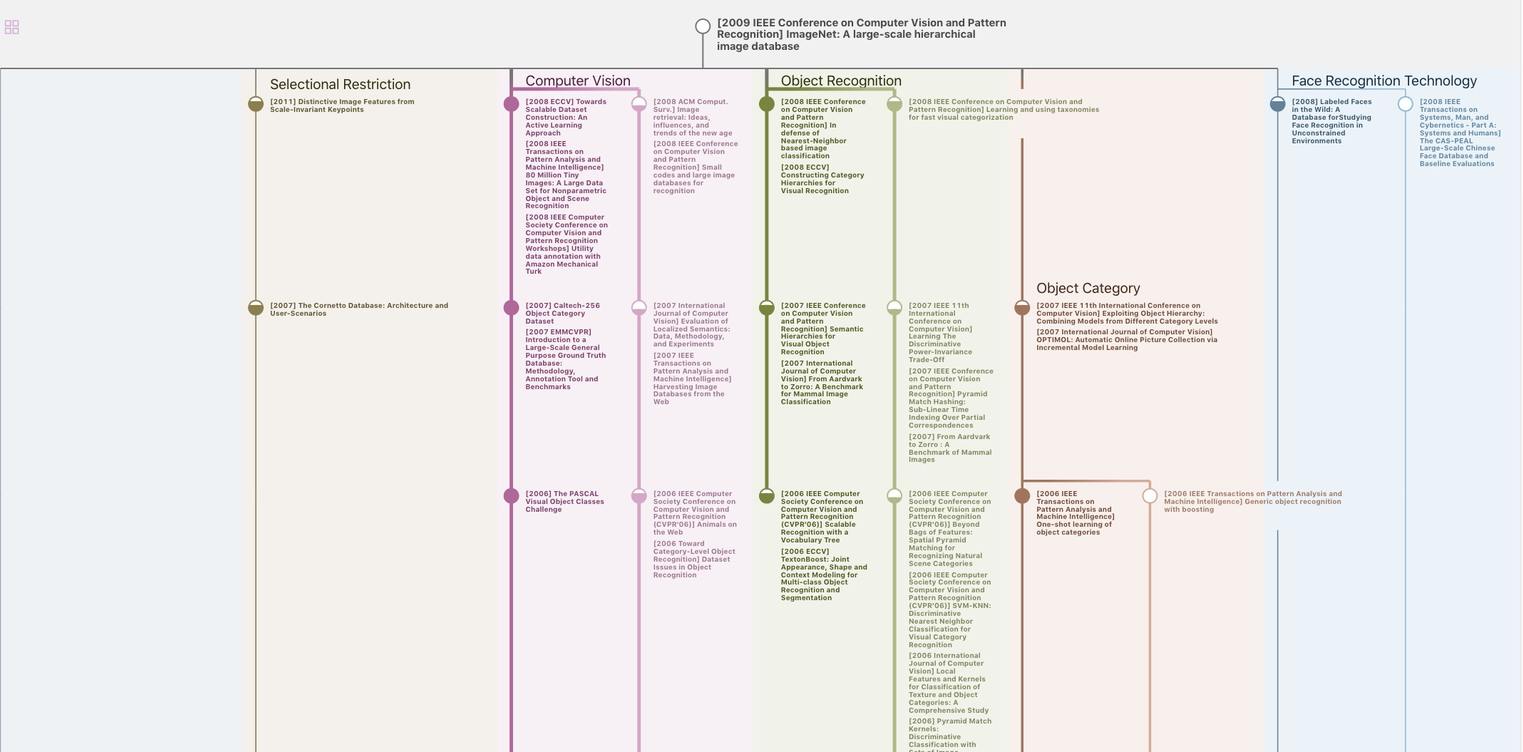
生成溯源树,研究论文发展脉络
Chat Paper
正在生成论文摘要