Improving Prediction of Late Symptoms using LSTM and Patient-reported Outcomes for Head and Neck Cancer Patients.
2023 IEEE 11th International Conference on Healthcare Informatics (ICHI)(2023)
摘要
Patient-Reported Outcomes (PRO) are collected directly from the patients using symptom questionnaires. In the case of head and neck cancer patients, PRO surveys are recorded every week during treatment with each patient's visit to the clinic and at different follow-up times after the treatment has concluded. PRO surveys can be very informative regarding the patient's status and the effect of treatment on the patient's quality of life (QoL). Processing PRO data is challenging for several reasons. First, missing data is frequent as patients might skip a question or a questionnaire altogether. Second, PROs are patient-dependent, a rating of 5 for one patient might be a rating of 10 for another patient. Finally, most patients experience severe symptoms during treatment which usually subside over time. However, for some patients, late toxicities persist negatively affecting the patient's QoL. These long-term severe symptoms are hard to predict and are the focus of this study. In this work, we model PRO data collected from head and neck cancer patients treated at the MD Anderson Cancer Center using the MD Anderson Symptom Inventory (MDASI) questionnaire as time series. We impute missing values with a combination of K nearest neighbor (KNN) and Long Short-Term Memory (LSTM) neural networks, and finally, apply LSTM to predict late symptom severity 12 months after treatment. We compare performance against clinical and ARIMA models. We show that the LSTM model combined with KNN imputation is effective in predicting late-stage symptom ratings for occurrence and severity under the AUC and F1 score metrics.
更多查看译文
关键词
Long Short-Term Memory (LSTM),Patient Reported Outcomes (PRO),Late Toxicity,Symptom Severity Prediction,KNN Baseline Imputation
AI 理解论文
溯源树
样例
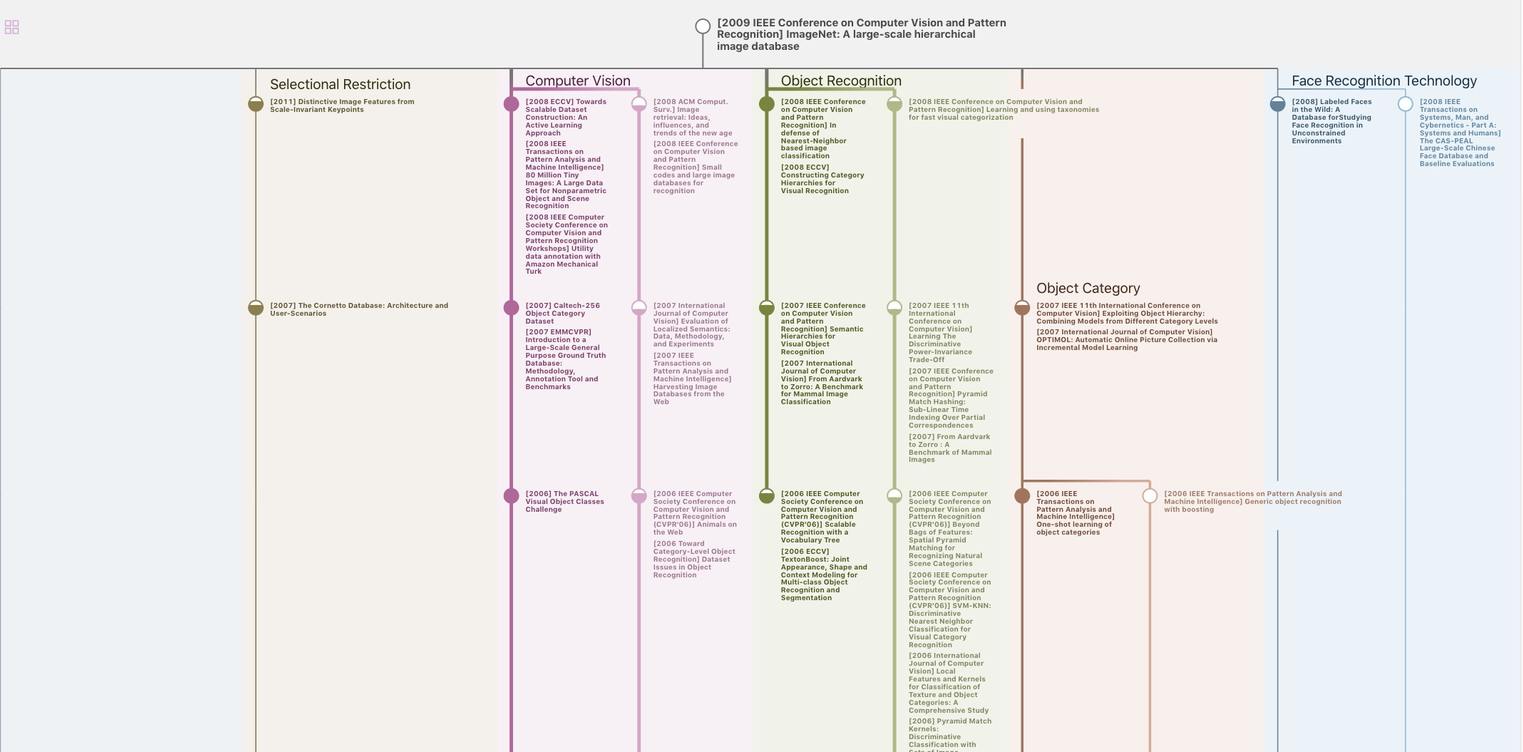
生成溯源树,研究论文发展脉络
Chat Paper
正在生成论文摘要