Federated Learning and Meta Learning: Approaches, Applications, and Directions
arXiv (Cornell University)(2024)
摘要
Over the past few years, significant advancements have been made in the field of machine learning (ML) to address resource management, interference management, autonomy, and decision-making in wireless networks. Traditional ML approaches rely on centralized methods, where data is collected at a central server for training. However, this approach poses a challenge in terms of preserving the data privacy of devices. To address this issue, federated learning (FL) has emerged as an effective solution that allows edge devices to collaboratively train ML models without compromising data privacy. In FL, local datasets are not shared, and the focus is on learning a global model for a specific task involving all devices. However, FL has limitations when it comes to adapting the model to devices with different data distributions. In such cases, meta learning is considered, as it enables the adaptation of learning models to different data distributions using only a few data samples. In this tutorial, we present a comprehensive review of FL, meta learning, and federated meta learning (FedMeta). Unlike other tutorial papers, our objective is to explore how FL, meta learning, and FedMeta methodologies can be designed, optimized, and evolved, and their applications over wireless networks. We also analyze the relationships among these learning algorithms and examine their advantages and disadvantages in real-world applications.
更多查看译文
关键词
Centralized learning,distributed learning,federated learning,meta learning,federated meta learning,wireless networks
AI 理解论文
溯源树
样例
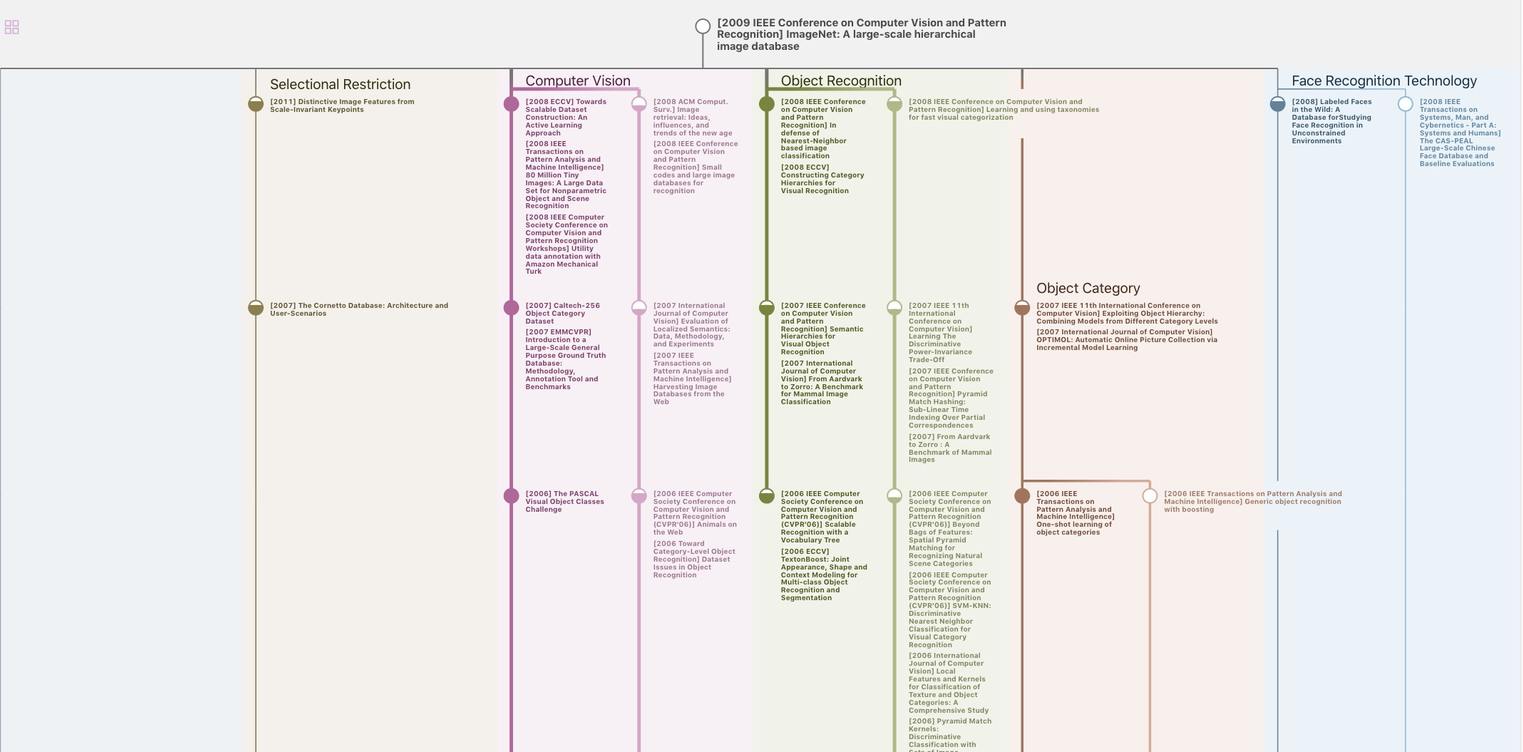
生成溯源树,研究论文发展脉络
Chat Paper
正在生成论文摘要