Learning Point-Language Hierarchical Alignment for 3D Visual Grounding
arXiv (Cornell University)(2022)
摘要
This paper presents a novel hierarchical alignment model (HAM) that learns multi-granularity visual and linguistic representations in an end-to-end manner. We extract key points and proposal points to model 3D contexts and instances, and propose point-language alignment with context modulation (PLACM) mechanism, which learns to gradually align word-level and sentence-level linguistic embeddings with visual representations, while the modulation with the visual context captures latent informative relationships. To further capture both global and local relationships, we propose a spatially multi-granular modeling scheme that applies PLACM to both global and local fields. Experimental results demonstrate the superiority of HAM, with visualized results showing that it can dynamically model fine-grained visual and linguistic representations. HAM outperforms existing methods by a significant margin and achieves state-of-the-art performance on two publicly available datasets, and won the championship in ECCV 2022 ScanRefer challenge. Code is available at~\url{https://github.com/PPjmchen/HAM}.
更多查看译文
关键词
3d,learning,point-language
AI 理解论文
溯源树
样例
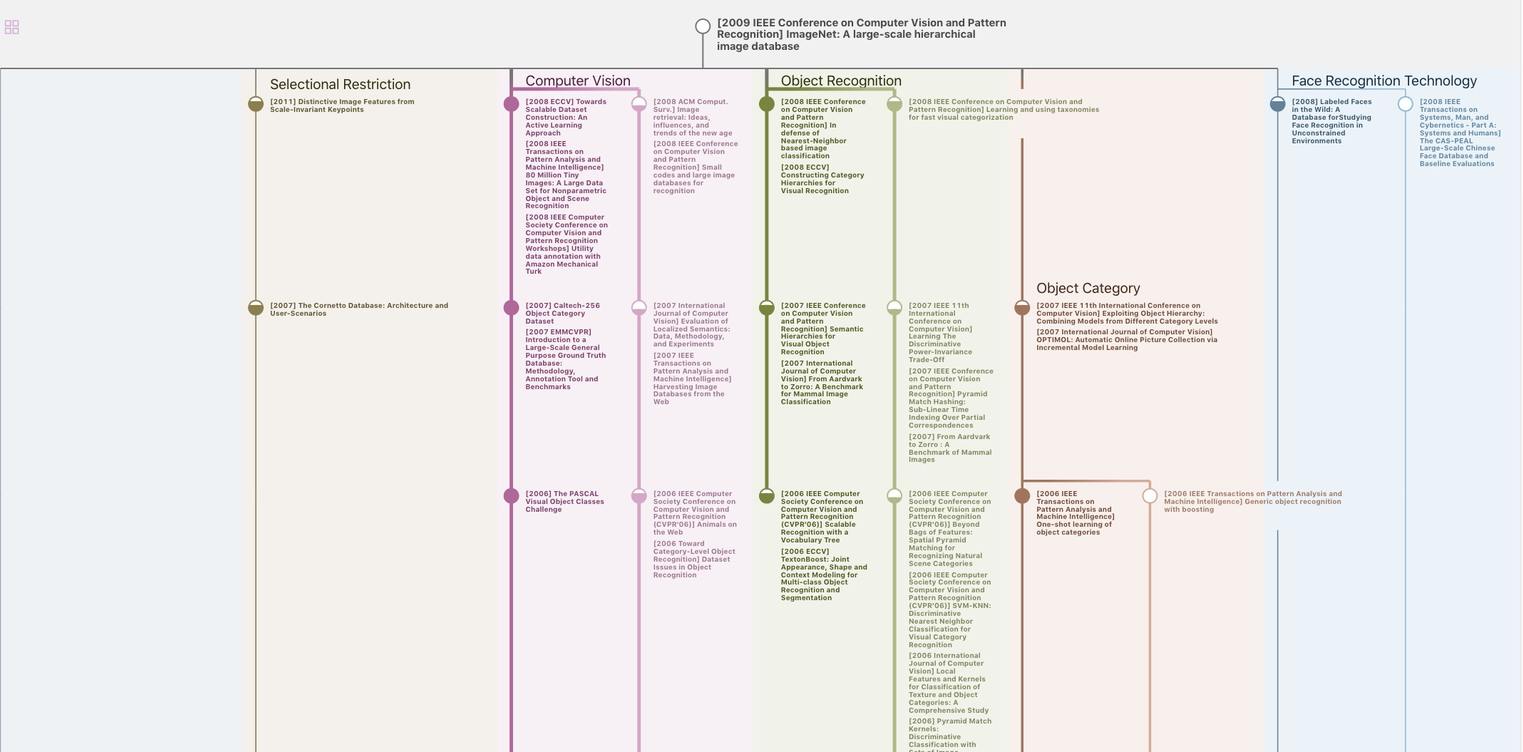
生成溯源树,研究论文发展脉络
Chat Paper
正在生成论文摘要