FP-GNN: a versatile deep learning architecture for enhanced molecular property prediction
arXiv (Cornell University)(2022)
摘要
Deep learning is an important method for molecular design and exhibits considerable ability to predict molecular properties, including physicochemical, bioactive, and ADME/T (absorption, distribution, metabolism, excretion, and toxicity) properties. In this study, we advanced a novel deep learning architecture, termed FP-GNN, which combined and simultaneously learned information from molecular graphs and fingerprints. To evaluate the FP-GNN model, we conducted experiments on 13 public datasets, an unbiased LIT-PCBA dataset, and 14 phenotypic screening datasets for breast cell lines. Extensive evaluation results showed that compared to advanced deep learning and conventional machine learning algorithms, the FP-GNN algorithm achieved state-of-the-art performance on these datasets. In addition, we analyzed the influence of different molecular fingerprints, and the effects of molecular graphs and molecular fingerprints on the performance of the FP-GNN model. Analysis of the anti-noise ability and interpretation ability also indicated that FP-GNN was competitive in real-world situations.
更多查看译文
关键词
versatile deep learning architecture,deep learning,property prediction,fp-gnn
AI 理解论文
溯源树
样例
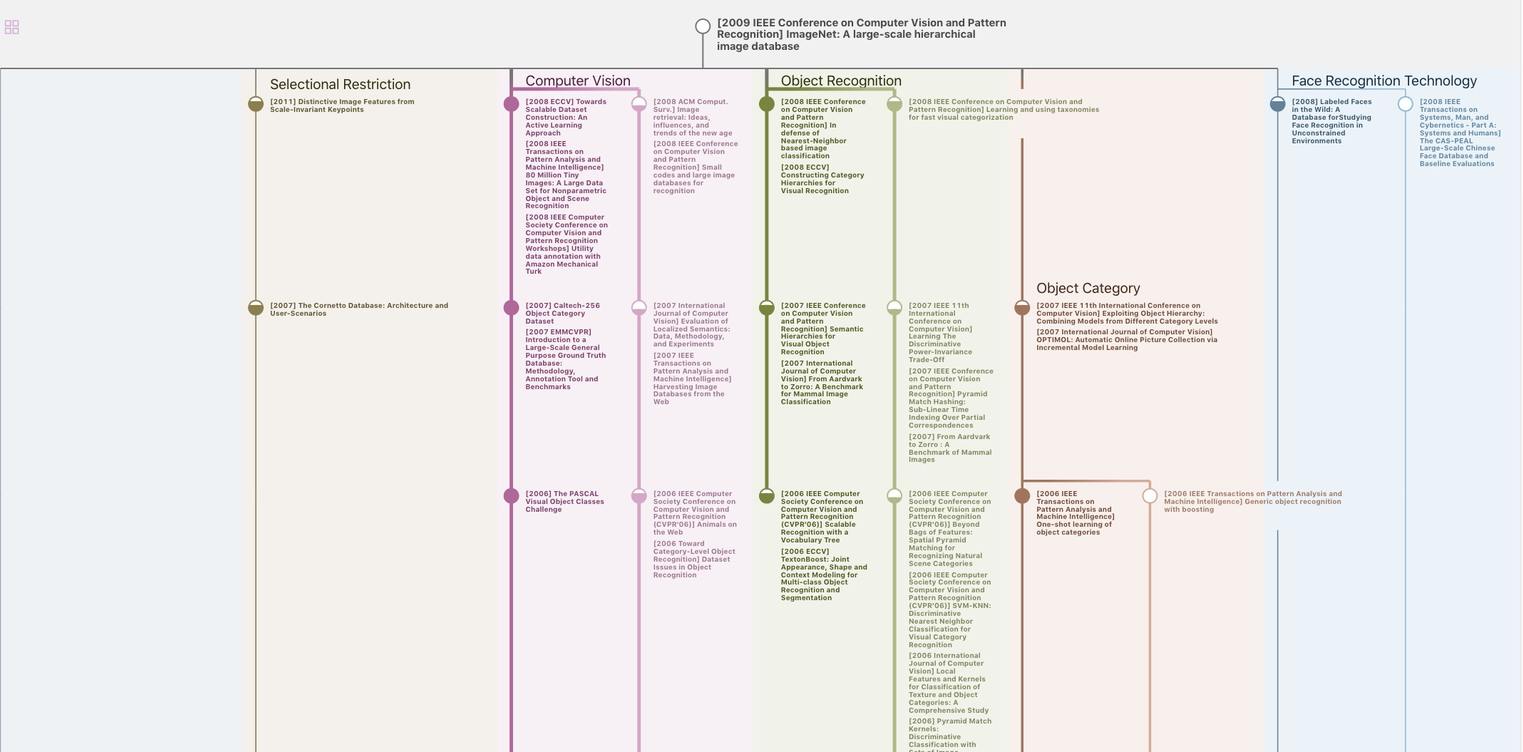
生成溯源树,研究论文发展脉络
Chat Paper
正在生成论文摘要