Are Sample-Efficient NLP Models More Robust?
arXiv (Cornell University)(2022)
摘要
Recent results in image classification and extractive question answering have observed that pre-trained models trained on less in-distribution data have better out-of-distribution performance. However, it is unclear how broadly these trends hold. We conduct a large empirical study across three tasks, three broadly-applicable modeling interventions (increasing model size, using a different adaptation method, and pre-training on more data), and 14 diverse datasets to investigate the relationship between sample efficiency (amount of data needed to reach a given ID accuracy) and robustness (how models fare on OOD evaluation). We find that higher sample efficiency is only correlated with better average OOD robustness on some modeling interventions and tasks, but not others. On individual datasets, models with lower sample efficiency can even be more robust. These results suggest that general-purpose methods for improving sample efficiency are unlikely to yield universal OOD robustness improvements, since such improvements are highly dataset- and task-dependent. Even in an era of large, multi-purpose pretrained models, task-specific decisions may often be necessary for OOD generalization.
更多查看译文
关键词
models,sample-efficient
AI 理解论文
溯源树
样例
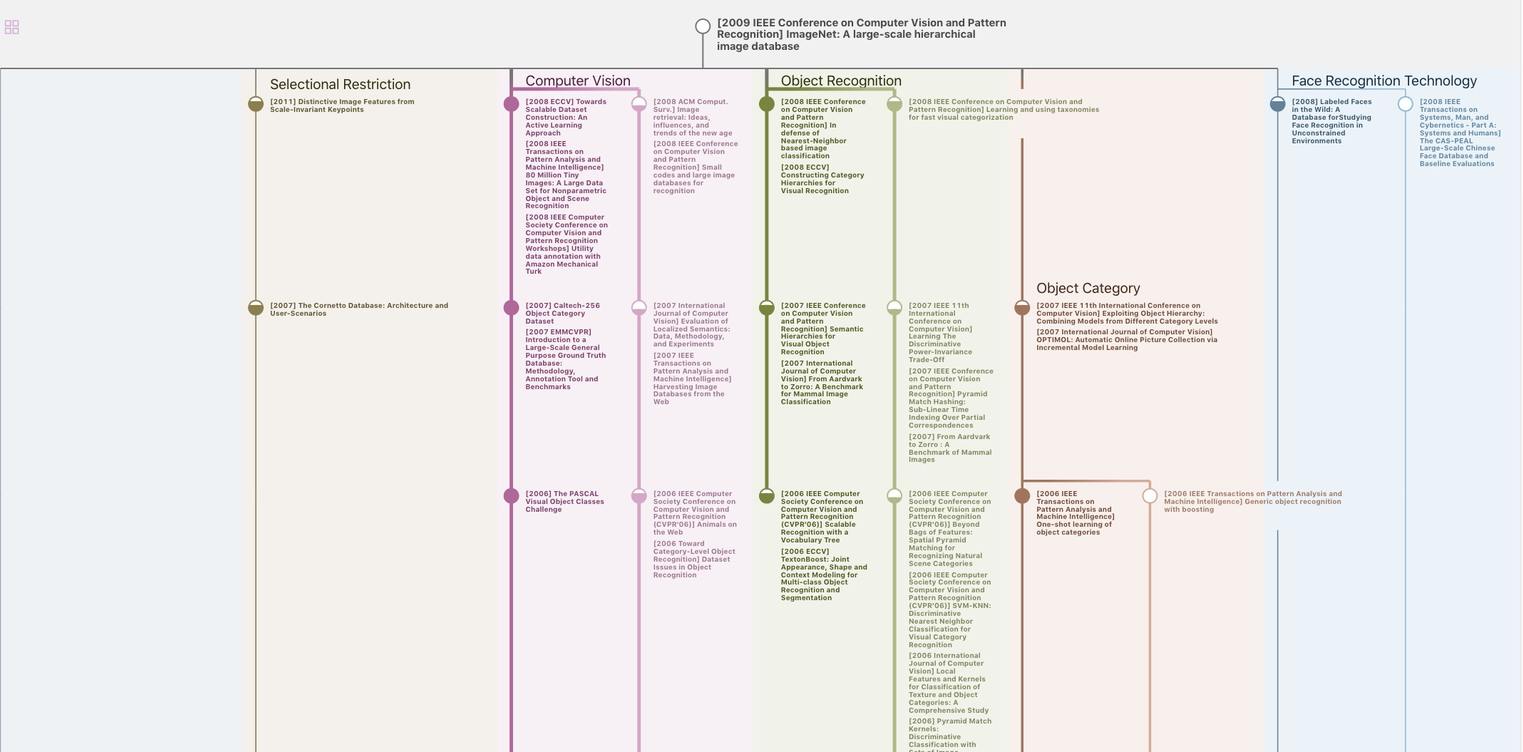
生成溯源树,研究论文发展脉络
Chat Paper
正在生成论文摘要