A Study on the Inductance and Thermal Regression and Optimization for Automatic Layout Design of Power Modules
2023 IEEE CPMT Symposium Japan (ICSJ)(2023)
摘要
Power modules with excellent inductance and temperature metrics are significant to meet the rising sophistication of energy demand in new technologies. In this paper, we use a surrogate-based approach to render optimal layouts of power modules with feasible and attractive inductance-temperature ratios at low computational budget. In particular, we use the class of feedforward networks to estimate the surrogate relationships between power module layout-design variables and inductance-temperature factors rendered from simulations; and Differential Evolution algorithms to optimize and locate feasible layout configurations of power module substrates minimizing inductance and temperature ratios. Our findings suggest the desirable classes of feedforward networks and gradient-free optimization algorithms being able to estimate and optimize power module layouts efficiently and effectively.
更多查看译文
关键词
power modules,neural networks,automatic layout design,system identification,optimization
AI 理解论文
溯源树
样例
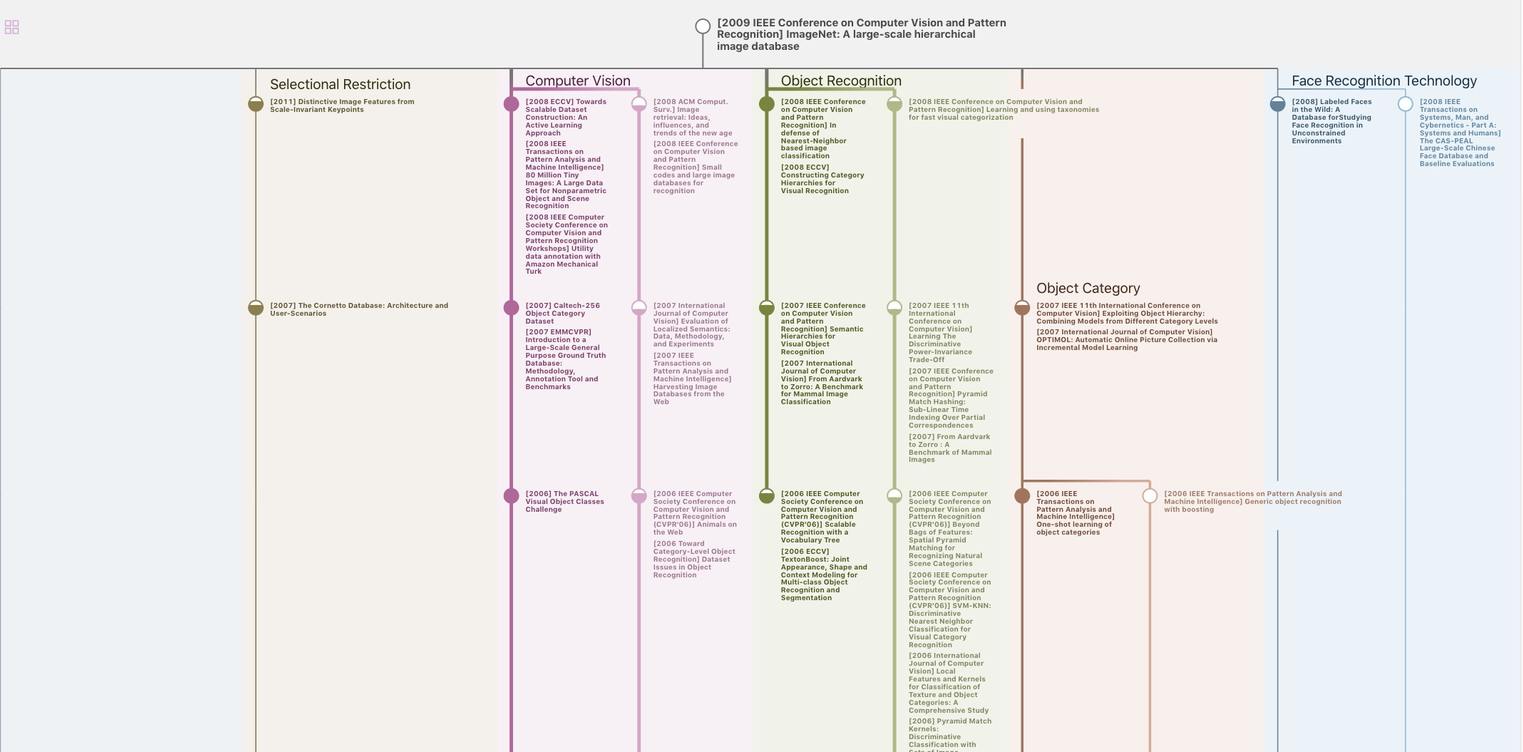
生成溯源树,研究论文发展脉络
Chat Paper
正在生成论文摘要