Class-Level Logit Perturbation
arXiv (Cornell University)(2023)
摘要
Features, logits, and labels are the three primary data when a sample passes through a deep neural network (DNN). Feature perturbation and label perturbation receive increasing attention in recent years. They have been proven to be useful in various deep learning approaches. For example, (adversarial) feature perturbation can improve the robustness or even generalization capability of learned models. However, limited studies have explicitly explored for the perturbation of logit vectors. This work discusses several existing methods related to class-level logit perturbation. A unified viewpoint between regular/irregular data augmentation and loss variations incurred by logit perturbation is established. A theoretical analysis is provided to illuminate why class-level logit perturbation is useful. Accordingly, new methodologies are proposed to explicitly learn to perturb logits for both the single-label and multilabel classification tasks. Meta-learning is also leveraged to determine the regular or irregular augmentation for each class. Extensive experiments on benchmark image classification datasets and their long-tail versions indicated the competitive performance of our learning method. As it only perturbs on logit, it can be used as a plug-in to fuse with any existing classification algorithms. All the codes are available at https://github.com/limengyang1992/lpl.
更多查看译文
关键词
Adversarial training,data augmentation,long-tail classification,multilabel classification
AI 理解论文
溯源树
样例
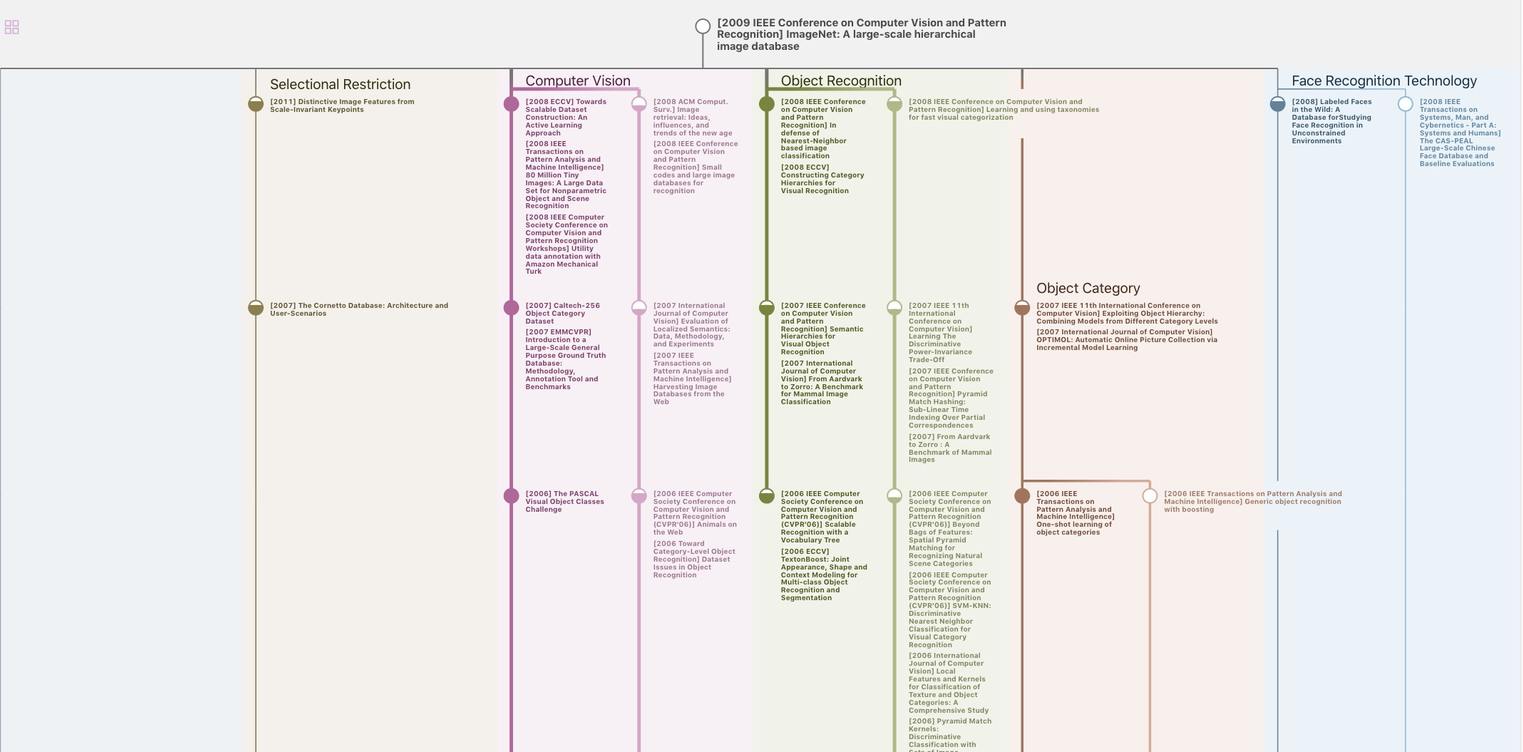
生成溯源树,研究论文发展脉络
Chat Paper
正在生成论文摘要