Personalized Federated Learning for Institutional Prediction Model using Electronic Health Records: A Covariate Adjustment Approach
2023 45TH ANNUAL INTERNATIONAL CONFERENCE OF THE IEEE ENGINEERING IN MEDICINE & BIOLOGY SOCIETY, EMBC(2023)
摘要
Federated learning (FL) has attracted attention as a technology that allows multiple medical institutions to collaborate on AI without disclosing each other's patient data. However, FL has the challenge of being unable to robustly learn when the data of participating clients is non-independently and non-identically distributed (Non-IID). Personalized Federated Learning (PFL), which constructs a personalized model for each client, has been proposed as a solution to this problem. However, conventional PFL methods do not ensure the interpretability of personalization, specifically, the identification of which data samples are contributed to each personalized learning, which is important for AI in medical applications. In this study, we propose a novel PFL framework, Federated Adjustment of Covariate (FedCov), which acquires a propensity score model representing the covariate shift among clients through prior FL, then learns a final model by weighting the contribution of each training sample to PFL based on the estimated propensity score. This approach enables both the learning of personalized models through covariate adjustment and the visualization of the contribution of each client to PFL. FedCov was evaluated in the prediction of in-hospital mortality across 50 hospitals in the eICU Collaborative Research Database, achieving an ROC-AUC of 0.750. This result outperformed the AUCs in the 0.720-0.735 range achieved by conventional FL methods and was closest to the AUC of 0.754 achieved by centralized learning.
更多查看译文
AI 理解论文
溯源树
样例
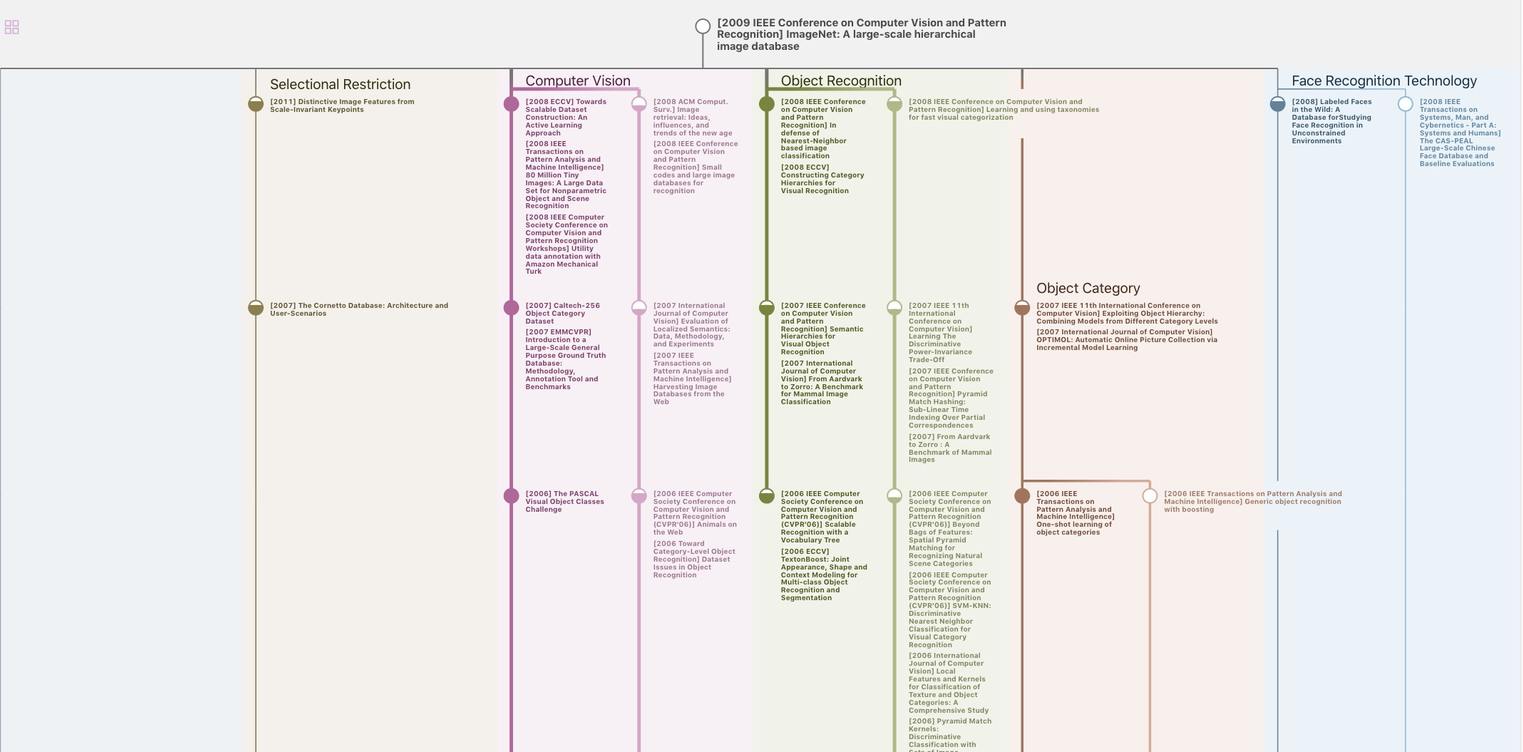
生成溯源树,研究论文发展脉络
Chat Paper
正在生成论文摘要