Automatic Annotation of PubMed Articles with MeSH Qualifiers
2023 45TH ANNUAL INTERNATIONAL CONFERENCE OF THE IEEE ENGINEERING IN MEDICINE & BIOLOGY SOCIETY, EMBC(2023)
摘要
The Medical Subject Headings (MeSH) is a comprehensive indexing vocabulary used to label millions of books and articles on PubMed. The MeSH annotation of a document consists of one or more descriptors, the main headings, and of qualifiers, subheadings specific to a descriptor. Currently, there are more than 34 million documents on PubMed, which are manually tagged with MeSH terms. In this paper, we describe a machine-learning procedure that, given a document and its MeSH descriptors, predicts the respective qualifiers. In our experiment, we restricted the dataset to documents with the Heart Transplantation descriptor and we only used the PubMed abstracts. We trained binary classifiers to predict qualifiers of this descriptor using logistic regression with a tfidf vectorizer and a fine-tuned DistilBERT model. We carried out a small-scale evaluation of our models with the Mortality qualifier on a test set consisting of 30 articles (15 positives and 15 negatives). This test set was then manually re-annotated by a cardiac surgeon, expert in thoracic transplantation. On this re-annotated test set, we obtained macroaveraged F1 scores of 0.81 for the logistic regression model and of 0.85 for the DistilBERT model. Both scores are higher than the macroaveraged F1 score of 0.76 from the initial PubMed manual annotation. Our procedure would be easily extensible to all the MeSH descriptors with sufficient training data and, we believe, would enable human annotators to complete the indexing work more easily.
更多查看译文
AI 理解论文
溯源树
样例
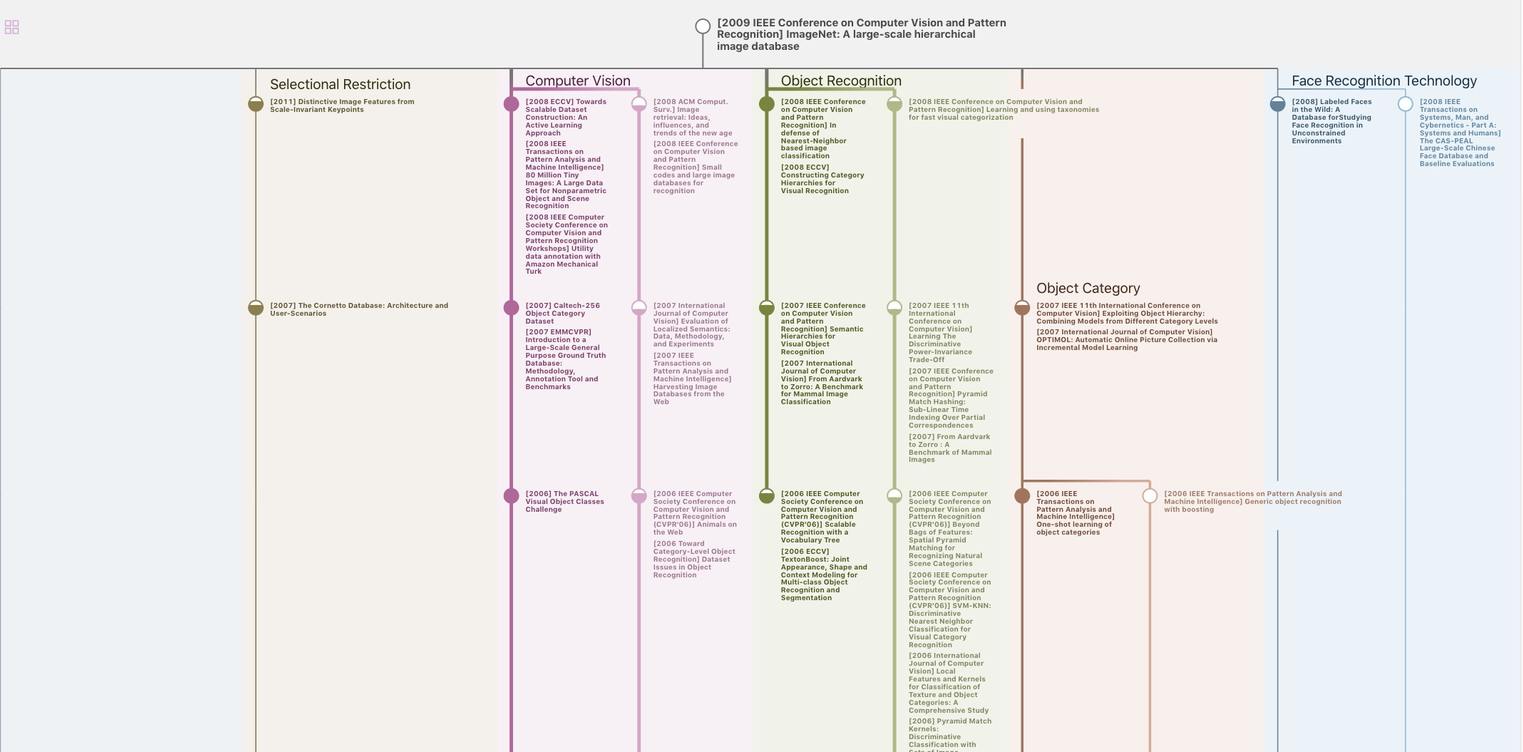
生成溯源树,研究论文发展脉络
Chat Paper
正在生成论文摘要