Training SAR Ship Detectors From Scratch with Customized Convolutional Neural Network
IEEE TRANSACTIONS ON AEROSPACE AND ELECTRONIC SYSTEMS(2023)
摘要
Deep learning-based object detectors have demonstrated remarkable performance in recent years, including their application in synthetic aperture radar (SAR) ship detection. However, these detectors often adopt backbones borrowed from classification models, requiring pretraining as a preliminary step. In addition, these borrowed backbones have several limitations that hinder their effectiveness when applied to SAR images. To overcome these drawbacks and improve accuracy, we designed a lightweight backbone called the SAR ship detection backbone and an attention frontend network called the SAR ship detection frontend to train ship detectors from scratch. The former is a meticulously designed backbone that leverages techniques such as cross-spatial partial dense blocks to extract features and has improved efficiency in accurately locating small ships. The latter is a carefully designed frontend network equipped with an attention module for prediction. The attention module comprises a spatial attention path, channel attention path, and identity mapping path. It facilitates the network's learning of more meaningful spatial and channelwise features. In addition, we defined the scales and aspect ratios of bounding boxes in datasets. The one-to-one label assigning strategy was used to match every anchor box with the corresponding ground truth. Complete intersection over union loss was also used to improve the performance of regression. The results obtained from public datasets show a favorable tradeoff between accuracy and time consumption. The findings of this work can be used as a reference for researchers to design SAR ship detectors tailored to their specific requirements and demands.
更多查看译文
关键词
Detectors,Feature extraction,Convolution,Synthetic aperture radar,Radar polarimetry,Object detection,Underwater vehicles
AI 理解论文
溯源树
样例
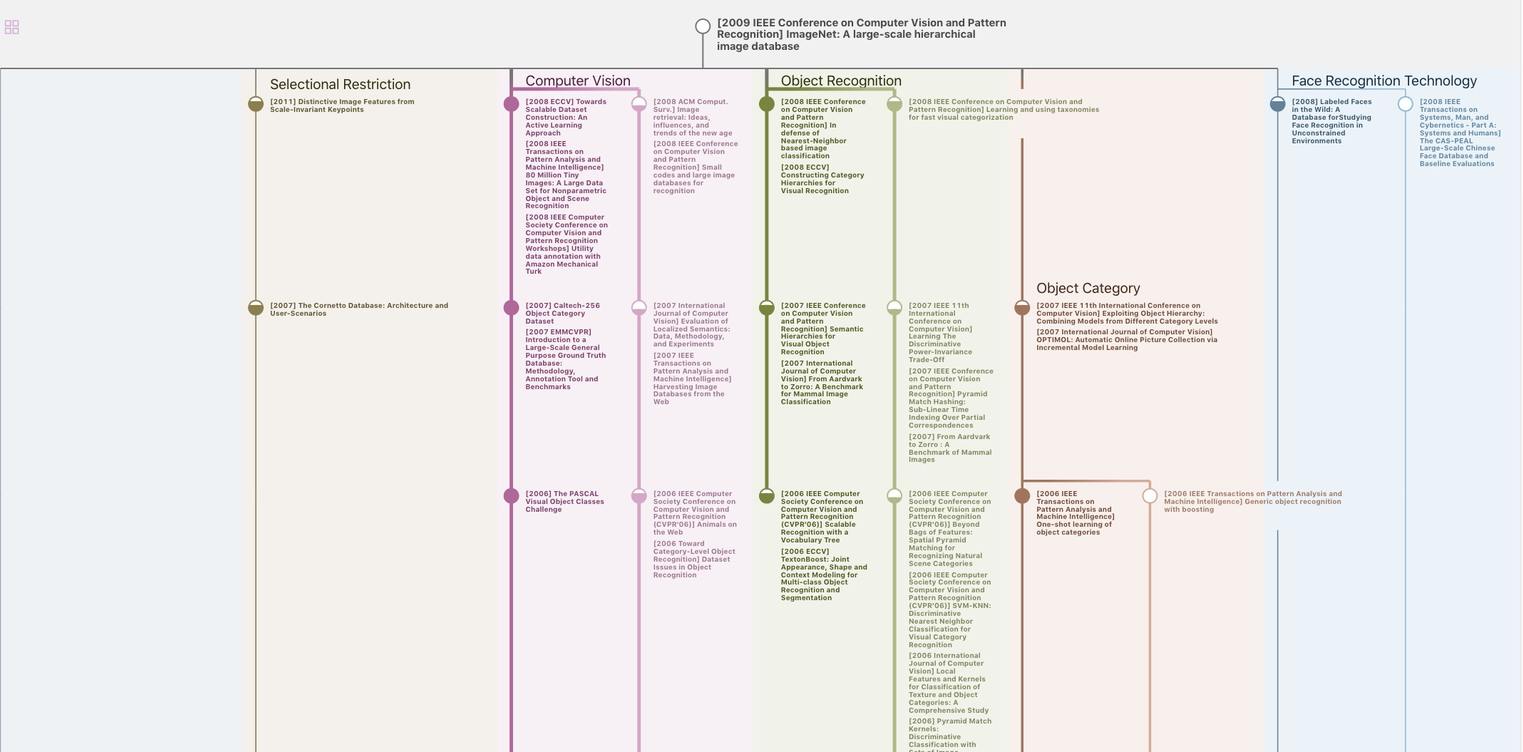
生成溯源树,研究论文发展脉络
Chat Paper
正在生成论文摘要