EMVS-MIMO Radar With Sparse Rx Geometry: Tensor Modeling and 2-D Direction Finding
IEEE TRANSACTIONS ON AEROSPACE AND ELECTRONIC SYSTEMS(2023)
摘要
The electromagnetic vector sensor (EMVS) embedded multiple-input-multiple-output (MIMO) radar, named EMVS-MIMO radar, offers a unique perspective on target localization, providing both 2-D angle and polarization status estimations of targets. However, the current frameworks limit the EMVS-MIMO radar's ability to achieve high-resolution 2-D direction finding while maintaining low complexity. To address this limitation, this article proposes a uniform rectangular array-based methodology with the intersensor spacing of the receiving array greater than half-wavelength, along with a high-resolution estimation strategy based on a parallel factor (PARAFAC) model. To optimize the identifiability of the PARAFAC model, the array measurement is first arranged into a third-order tensor, and the six possible PARAFAC tensor models are established. Factor matrices are then estimated via PARAFAC decomposition, and 2-D direction finding is achieved by combining the spatial rotational invariance technique with the normalized vector-cross product technique. The proposed PARAFAC algorithm can be extended to other sparse uniform geometries, such as L-shaped arrays and parallel linear arrays. The larger array aperture and inherent tensor gain of the proposed PARAFAC approaches offer superior estimation performance compared to existing frameworks. In addition, the flexibility of the PARAFAC model may make it suitable for single snapshot scenarios. Finally, the improvements of the proposed approaches are validated by numerical simulations.
更多查看译文
关键词
Tensors,Estimation,Sensor arrays,Radar,MIMO radar,Geometry,Mutual coupling,2-D direction finding,electromagnetic vector sensor,multiple-input-multiple-output (MIMO) radar,sparse array
AI 理解论文
溯源树
样例
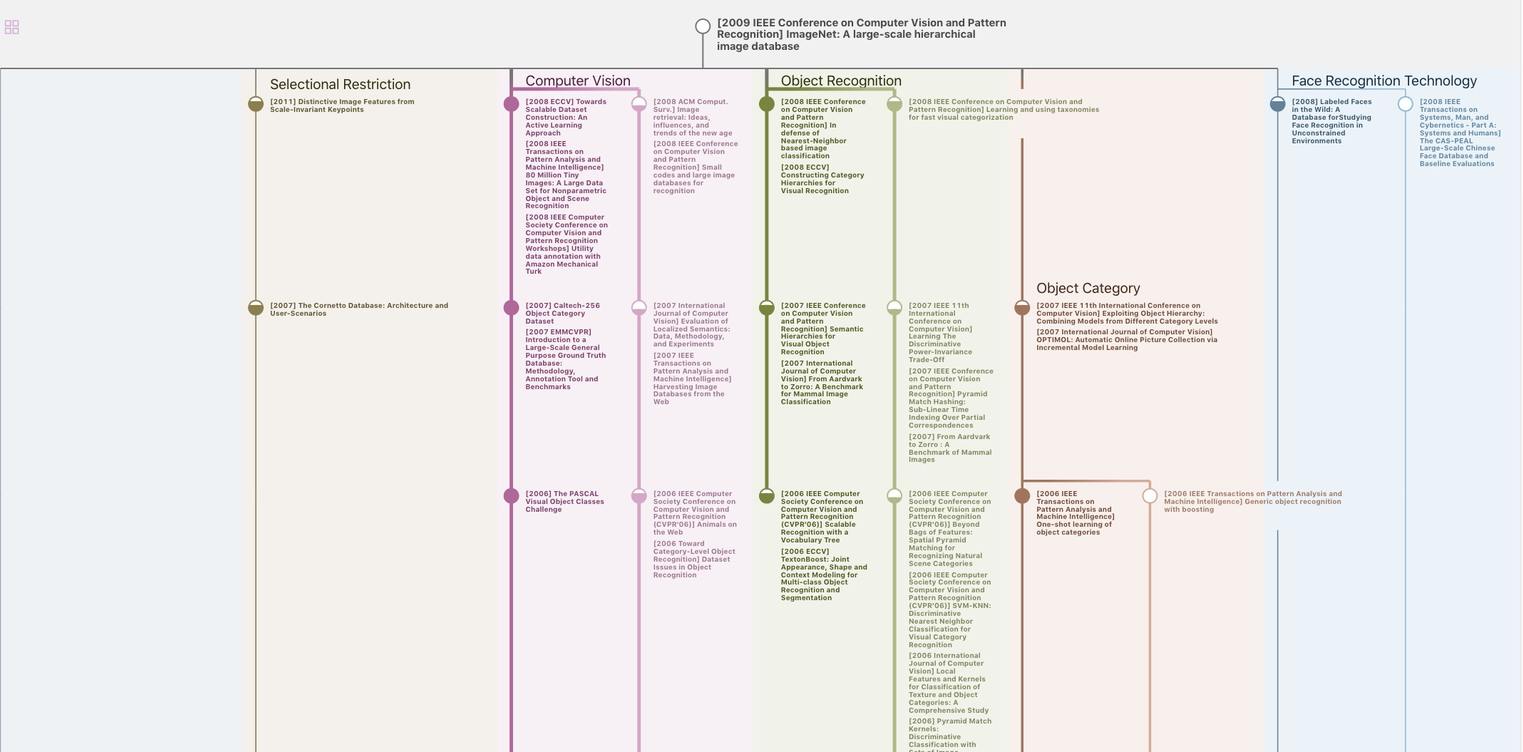
生成溯源树,研究论文发展脉络
Chat Paper
正在生成论文摘要