MED-SE: Medical Entity Definition-based Sentence Embedding
arXiv (Cornell University)(2022)
摘要
We propose Medical Entity Definition-based Sentence Embedding (MED-SE), a novel unsupervised contrastive learning framework designed for clinical texts, which exploits the definitions of medical entities. To this end, we conduct an extensive analysis of multiple sentence embedding techniques in clinical semantic textual similarity (STS) settings. In the entity-centric setting that we have designed, MED-SE achieves significantly better performance, while the existing unsupervised methods including SimCSE show degraded performance. Our experiments elucidate the inherent discrepancies between the general- and clinical-domain texts, and suggest that entity-centric contrastive approaches may help bridge this gap and lead to a better representation of clinical sentences.
更多查看译文
关键词
sentence embedding,definition-based
AI 理解论文
溯源树
样例
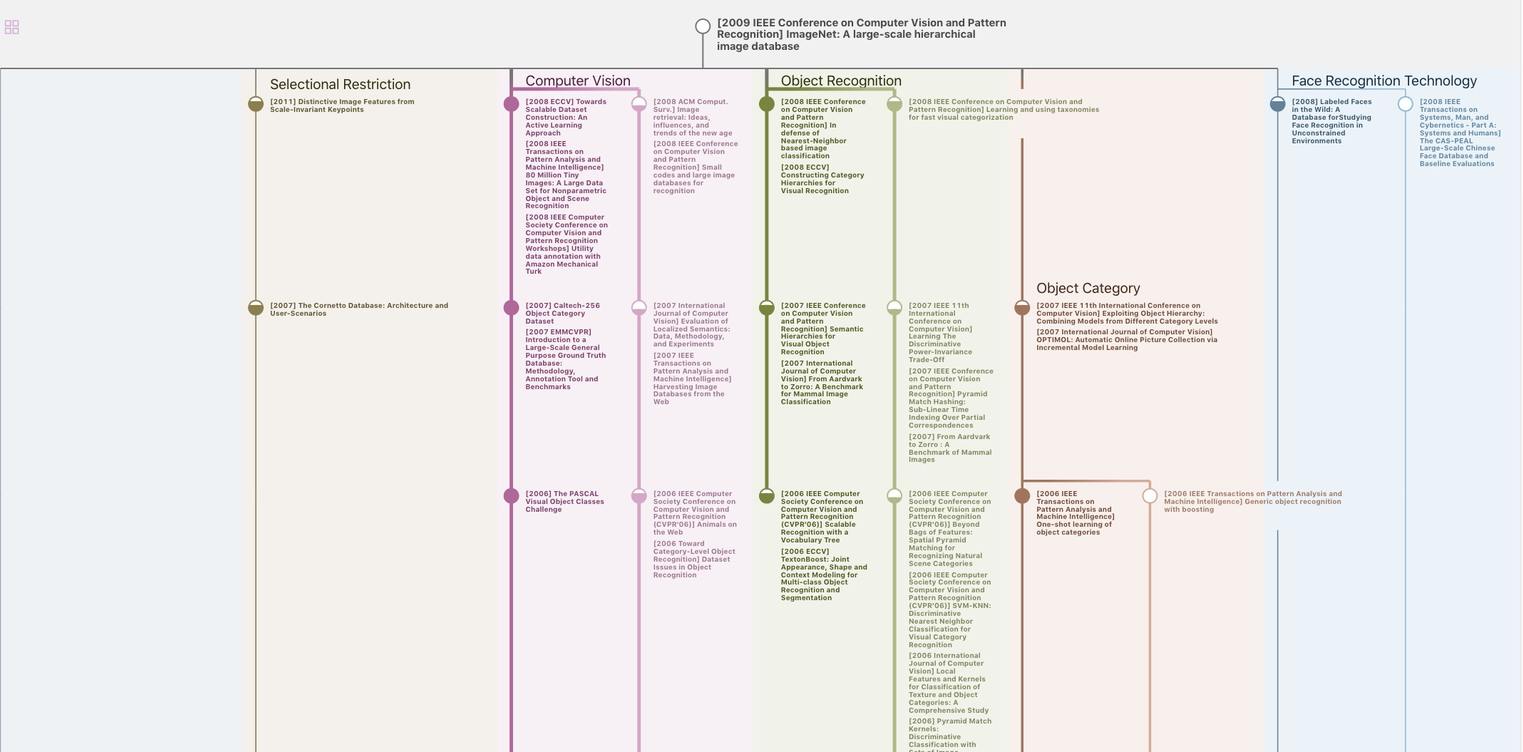
生成溯源树,研究论文发展脉络
Chat Paper
正在生成论文摘要